filmov
tv
Harnessing Artificial Data Generation to Protect Sensitive Data
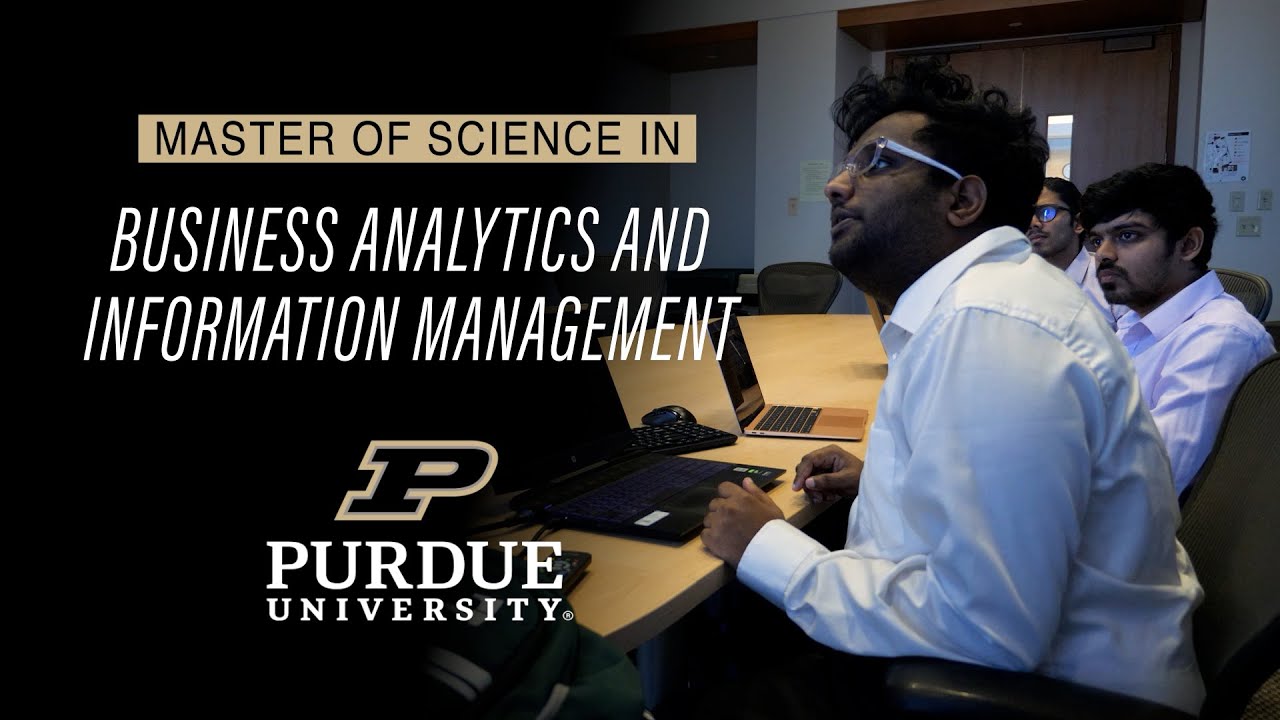
Показать описание
Abstract:
Firms collect and analyze sensitive consumer data to gain insights about their business and
develop cutting edge strategies. With increasing regulations and the risk of sensitive data leakage,
firms employ several stringent practices to ensure data privacy. However, these practices drive-
up operational costs and opportunity losses. Synthetic data generation allows firms to relax these
practices at the cost of some predictive power. The problem lies in understanding what data
generation methodologies exist, where they could be used, and how such data would perform for
various predictive modeling approaches. In collaboration with a national timeshare firm we provide
some best practices that firms in all domains can follow to adopt synthetic data into their data
science workflow to reduce operational costs and legal barriers needed to maintain data privacy.
Our empirical results demonstrate how generating and using synthetic data can perform for
various predictive models most likely to be used in business against the privacy tradeoffs one is
likely to encounter.
Student Team:
---
Make sure to LIKE this video and SUBSCRIBE to our channel to keep tabs on everything the Mitchell E. Daniels, Jr. School of Business has to offer.
Follow us on social!
Firms collect and analyze sensitive consumer data to gain insights about their business and
develop cutting edge strategies. With increasing regulations and the risk of sensitive data leakage,
firms employ several stringent practices to ensure data privacy. However, these practices drive-
up operational costs and opportunity losses. Synthetic data generation allows firms to relax these
practices at the cost of some predictive power. The problem lies in understanding what data
generation methodologies exist, where they could be used, and how such data would perform for
various predictive modeling approaches. In collaboration with a national timeshare firm we provide
some best practices that firms in all domains can follow to adopt synthetic data into their data
science workflow to reduce operational costs and legal barriers needed to maintain data privacy.
Our empirical results demonstrate how generating and using synthetic data can perform for
various predictive models most likely to be used in business against the privacy tradeoffs one is
likely to encounter.
Student Team:
---
Make sure to LIKE this video and SUBSCRIBE to our channel to keep tabs on everything the Mitchell E. Daniels, Jr. School of Business has to offer.
Follow us on social!
Комментарии