filmov
tv
22. Gradient Descent: Downhill to a Minimum
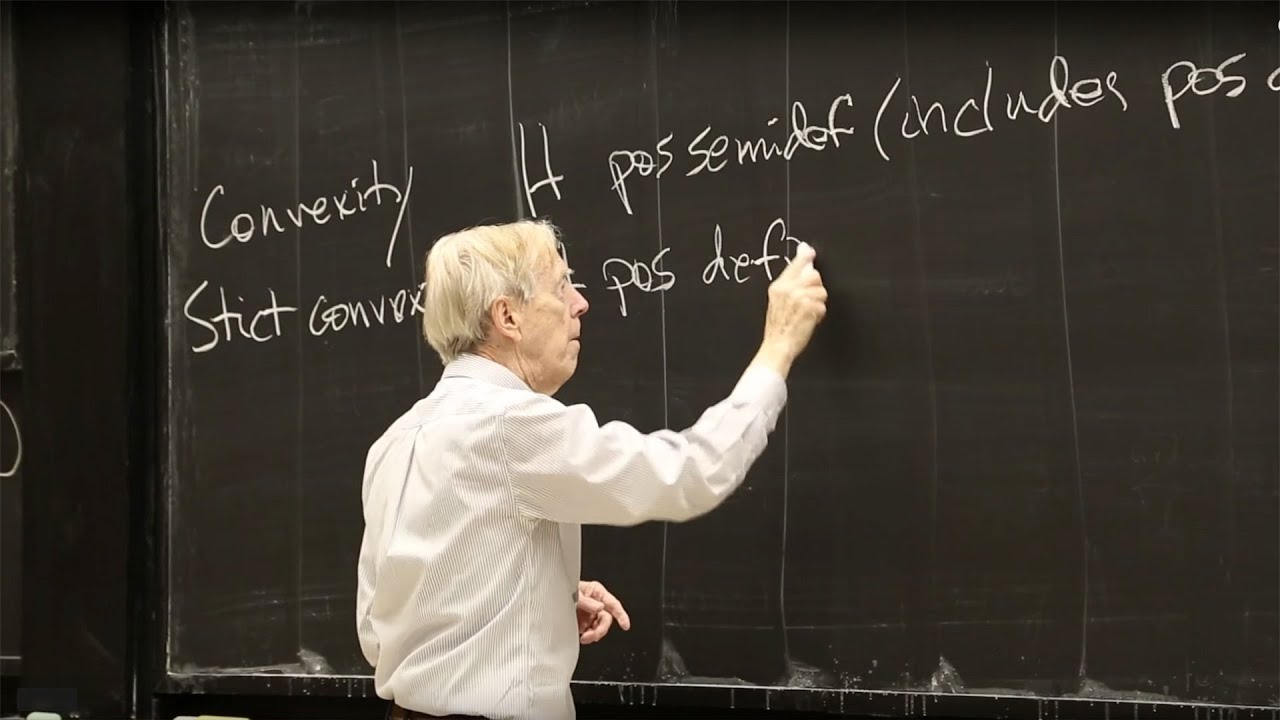
Показать описание
MIT 18.065 Matrix Methods in Data Analysis, Signal Processing, and Machine Learning, Spring 2018
Instructor: Gilbert Strang
Gradient descent is the most common optimization algorithm in deep learning and machine learning. It only takes into account the first derivative when performing updates on parameters - the stepwise process that moves downhill to reach a local minimum.
License: Creative Commons BY-NC-SA
Instructor: Gilbert Strang
Gradient descent is the most common optimization algorithm in deep learning and machine learning. It only takes into account the first derivative when performing updates on parameters - the stepwise process that moves downhill to reach a local minimum.
License: Creative Commons BY-NC-SA
22. Gradient Descent: Downhill to a Minimum
Intro to Gradient Descent || Optimizing High-Dimensional Equations
Gradient Descent in 3 minutes
Gradient Descent, Step-by-Step
Gradient Descent with momentum and Steepest Descent
Gradient Descent II
Easiest Way to Understand Gradient Descent Step by Step (downhill to a minimum with derivatives)
gradientDescent.m Gradient Descent Implementation - Machine Learning
Gradient Descent
Gradient Descent Visualised #mathematicsformachinelearning #deeplearning #gradientdescent
Gradient descent
Gradient Descent Explained
PyTorch Lecture 03: Gradient Descent
EP5: Convergence in Gradient Descent
Gradient Descent
Introduction to Optimization . Part 3 - Gradient-Based Optimization
Gradient descent method (steepest descent)
Gradient Descent Method - EXCEL/VBA
ML in 3 minute - Gradient Descent
Gradient Descent on Non-Convex Functions | Data Science Interview Questions | Machine Learning
What is Gradient Descent in Machine Learning?
Visualizing Stochastic & Batch Gradient Descent in Matplotlib
FoDA - L17 : Gradient Descent for Fitting Data with Squared Loss (Chapter 6.4)
Gradient Descent
Комментарии