filmov
tv
Handling Sparse Rewards in Reinforcement Learning Using Model Predictive Control
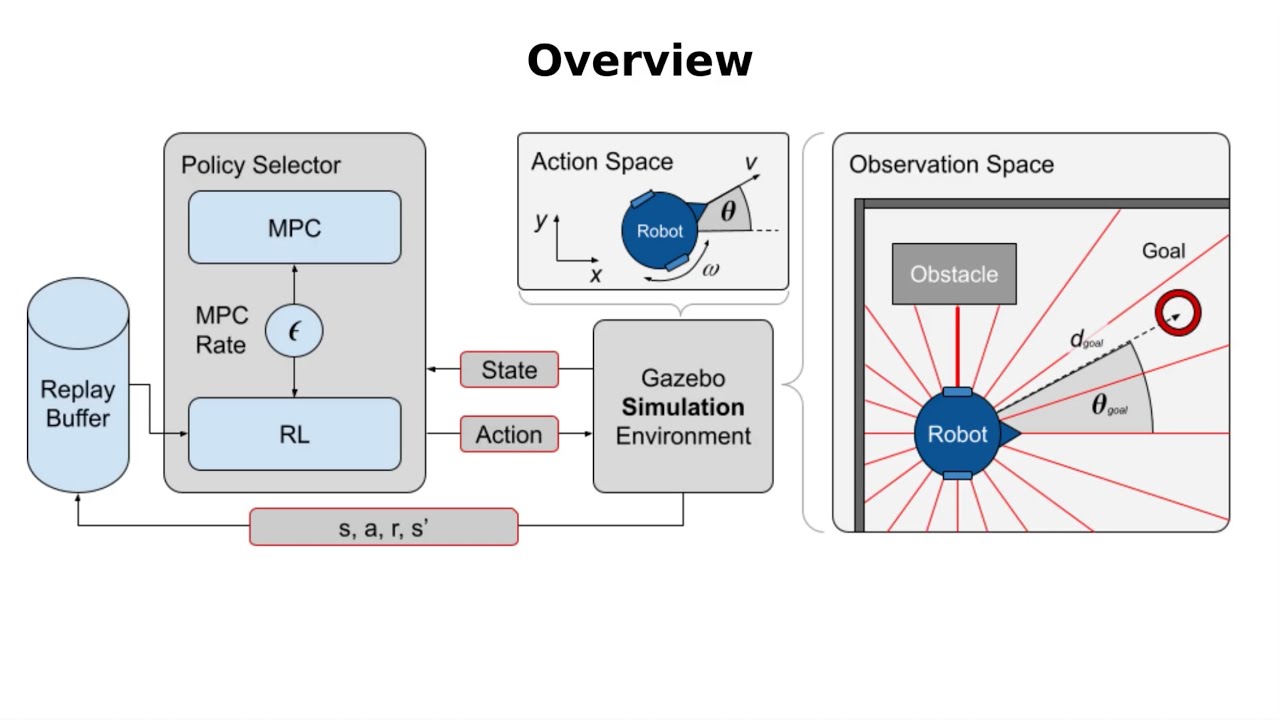
Показать описание
This video shows some results of the work presented in our paper "Handling Sparse Rewards in Reinforcement Learning Using Model Predictive Control", published by M. Dawood, N. Dengler, J. de Heuvel, and M. Bennewitz at the IEEE International Conference on Robotics & Automation (ICRA), 2023.
The design of the reward function in Reinforcement learning (RL) requires detailed domain expertise to ensure that agents are able to learn the desired behaviour. Using a sparse reward conveniently mitigates these challenges. However, the sparse reward represents a challenge on its own.
We therefore address the sparse reward problem in RL and propose to use model predictive control (MPC) as an experience source for training RL agents. Without the need for reward shaping, we successfully apply our approach in the field of mobile robot navigation both in simulation and real-world experiments. We furthermore demonstrate great improvement over pure RL algorithms in terms of success rate as well as number of collisions and timeouts. The video shows that MPC as an experience source improves the agent’s learning process for a given task in the case of sparse rewards
The design of the reward function in Reinforcement learning (RL) requires detailed domain expertise to ensure that agents are able to learn the desired behaviour. Using a sparse reward conveniently mitigates these challenges. However, the sparse reward represents a challenge on its own.
We therefore address the sparse reward problem in RL and propose to use model predictive control (MPC) as an experience source for training RL agents. Without the need for reward shaping, we successfully apply our approach in the field of mobile robot navigation both in simulation and real-world experiments. We furthermore demonstrate great improvement over pure RL algorithms in terms of success rate as well as number of collisions and timeouts. The video shows that MPC as an experience source improves the agent’s learning process for a given task in the case of sparse rewards