filmov
tv
Hila Chefer - Transformer Explainability
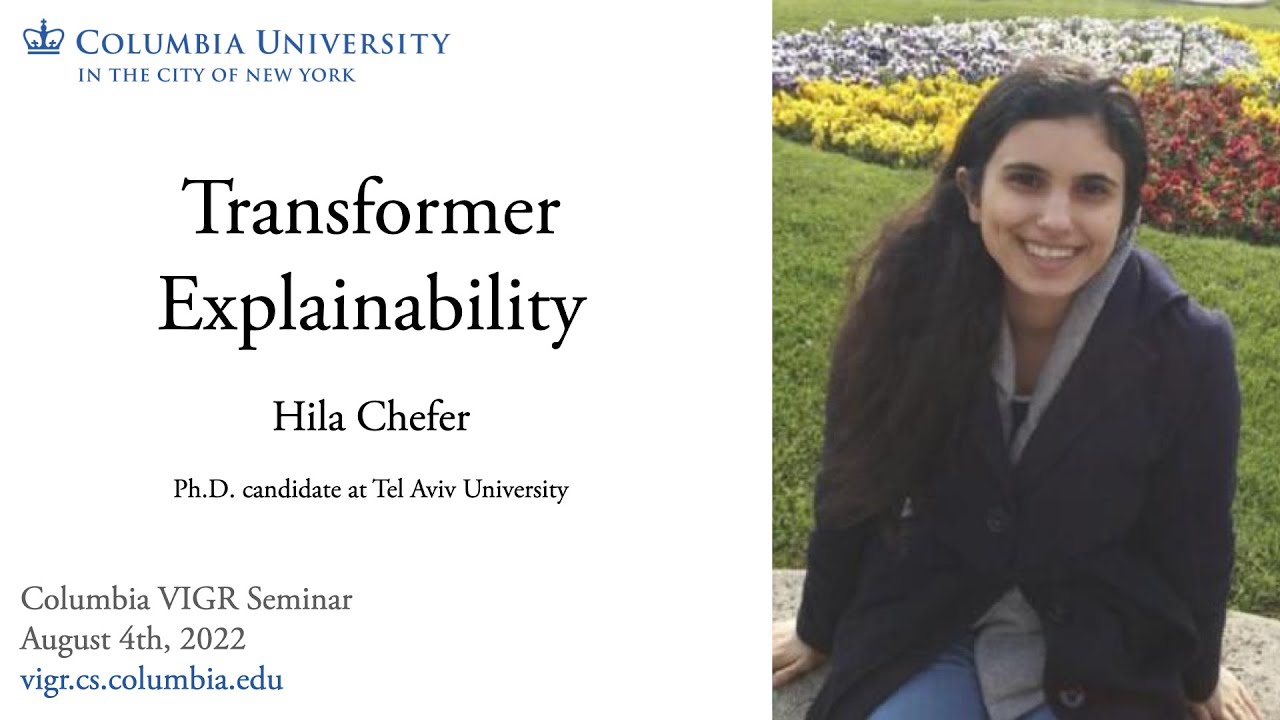
Показать описание
August 4th, 2022. Columbia University
Abstract:
Transformers have revolutionized deep learning research across many disciplines, starting from NLP and expanding to vision, speech, and more. In my talk, I will explore several milestones toward interpreting all families of Transformers, including unimodal, bi-modal, and encoder-decoder Transformers. I will present working examples and results that cover some of the most prominent models, including CLIP, ViT, and LXMERT. I will then present our recent explainability-driven fine-tuning technique that significantly improves the robustness of Vision Transformers (ViTs). The loss we employ ensures that the model bases its prediction on the relevant parts of the input rather than supportive cues (e.g., background).
Bio:
Hila is a Ph.D. candidate at Tel Aviv University, advised by Prof. Lior Wolf. Her research focuses on developing reliable XAI algorithms and leveraging them to promote model accuracy and fairness.
Abstract:
Transformers have revolutionized deep learning research across many disciplines, starting from NLP and expanding to vision, speech, and more. In my talk, I will explore several milestones toward interpreting all families of Transformers, including unimodal, bi-modal, and encoder-decoder Transformers. I will present working examples and results that cover some of the most prominent models, including CLIP, ViT, and LXMERT. I will then present our recent explainability-driven fine-tuning technique that significantly improves the robustness of Vision Transformers (ViTs). The loss we employ ensures that the model bases its prediction on the relevant parts of the input rather than supportive cues (e.g., background).
Bio:
Hila is a Ph.D. candidate at Tel Aviv University, advised by Prof. Lior Wolf. Her research focuses on developing reliable XAI algorithms and leveraging them to promote model accuracy and fairness.
Hila Chefer - Transformer Explainability
Generic Attention-model Explainability for Interpreting Bi-Modal and Encoder-Decoder Transformers
Optimizing Relevance Maps of Vision Transformers Improves Robustness
All Things ViTs || CVPR 2023 Tutorial || Hila Chefer and Sayak Paul
Intro to Transformers and Transformer Explainability
TargetCLIP: Image-based CLIP-Guided Essence Transfer [ECCV'22]
Computer Vision Meetup: Leveraging Attention for Improved Accuracy and Robustness
Transformer Model Interpretability - Tutorial
Transforming Finance: BloombergGPT's Powerful Architecture Explained
SE4AI: Explainability and Interpretability (Part 1)
Architecture of Transformers
Transformers and Self Attention - Deep Learning MSc course, WS2023-2023 HHU Lecture (01/2023)
MSG-Transformer: Exchanging Local Spatial Information by Manipulating Messenger Tokens
[2021 CVPR] Transformer Interpretability Beyond Attention Visualization paper explained!
Cutting Edge Explainability: Interpreting Transformers and Going Beyond Heatmaps (TR AI Week 2021)
Attention in Deep Learning -- Intro and Motivation
WeCNLP 2021: LXMERT Model Compression for VQA
Attention-Based Semantic Guidance for Text-to-image Diffusion Models
MNIST vision transformer
PyTorch Captum - finally understand AI's decisions?
BIRDS 2021 Eljasik-Swoboda - Querying by Example Using Bootstrapped Explainable Text Categorization
Explanation Approaches for Transformers
Stanford CS25: V1 I Transformer Circuits, Induction Heads, In-Context Learning
ECS 289G Talk: Understanding Robustness of Transformers for Image Classification
Комментарии