filmov
tv
LHMP 2021, Part 7: Invited talk by Jonathan P. How (MIT)
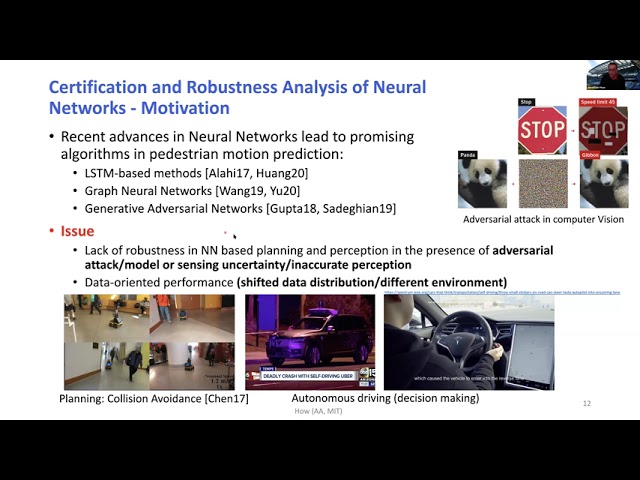
Показать описание
3rd Workshop on Long-term Human Motion Prediction (LHMP)
Part 7: Invited talk by Jonathan P. How (MIT)
Understanding pedestrian behavior is crucial for safe autonomous navigation on the roads and in crowded environments. However, pedestrian motion is highly stochastic and involves various forms of interaction with the environment and other users of the road/sidewalk. While learning from large datasets is a promising strategy for developing predictive models, current methods are often limited to batch (offline) settings, thus limiting the ability for the model to generalize to new environments and update as new data becomes available. To address this challenge, this talk presents a Similarity-based Incremental Learning Algorithm (SILA) for pedestrian motion prediction. The talk will also discuss a new sharing pipeline called SimFuse, which enables autonomous agents to update their models by communicating with other vehicles (V2V) or infrastructure (V2I). Finally, the talk will describe our recent work toward certifying learned models to provide safety and robustness guarantees. This talk will conclude by highlighting some remaining open challenges in the field.
IEEE International Conference on Robotics and Automation (ICRA),
May 31, 2021
Part 7: Invited talk by Jonathan P. How (MIT)
Understanding pedestrian behavior is crucial for safe autonomous navigation on the roads and in crowded environments. However, pedestrian motion is highly stochastic and involves various forms of interaction with the environment and other users of the road/sidewalk. While learning from large datasets is a promising strategy for developing predictive models, current methods are often limited to batch (offline) settings, thus limiting the ability for the model to generalize to new environments and update as new data becomes available. To address this challenge, this talk presents a Similarity-based Incremental Learning Algorithm (SILA) for pedestrian motion prediction. The talk will also discuss a new sharing pipeline called SimFuse, which enables autonomous agents to update their models by communicating with other vehicles (V2V) or infrastructure (V2I). Finally, the talk will describe our recent work toward certifying learned models to provide safety and robustness guarantees. This talk will conclude by highlighting some remaining open challenges in the field.
IEEE International Conference on Robotics and Automation (ICRA),
May 31, 2021