filmov
tv
What are Autoregressive (AR) Models
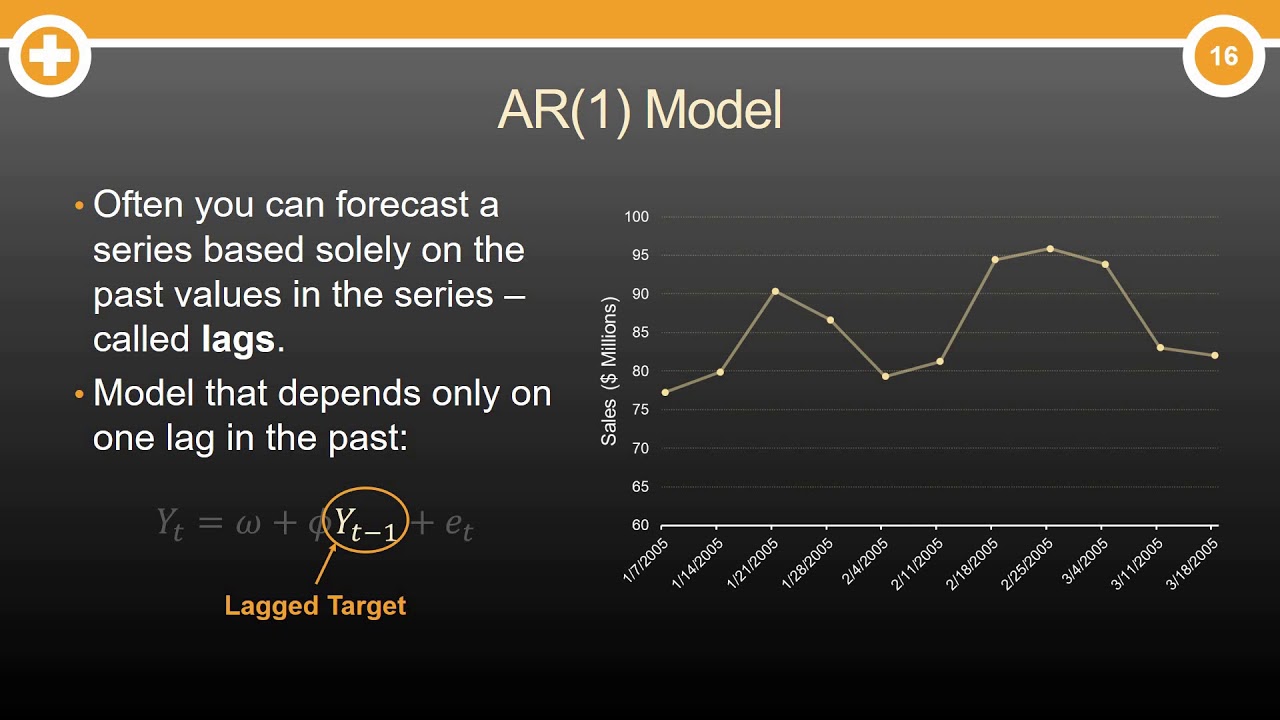
Показать описание
Time to start talking about some of the most popular models in time series - ARIMA models. First things first, let's look at the AR piece - autoregressive models!
Time Series Talk : Autoregressive Model
What are Autoregressive (AR) Models
Autoregressive Models | Auto Regression | Machine Learning for Beginners | Edureka
CFA® Level II Quant - Autoregressive (AR) Models: Mean reversion, Covariance Stationarity
Auto Regressive Time Series Model | AR Model
Econometrics - Autoregressive (AR) Models
Introduction to the Autoregressive Model
Auto Regression(AR) Model| Time Series Forecasting #2
Auto Regressive Models (AR) | Time Series Analysis | Data Analytics
How to do Autoregression with Data Analysis toolpak
Introduction to Time Series Analysis: AR MA ARIMA Models, Stationarity, and Data Differencing
Auto Regression(AR) Model in Python| Time Series Forecasting #5|
AR(1) Autoregressive Process: Mean, Autocovariances, ACF
Classical Time Series Models AR,MA,ARMA,ARIMA - Understanding time series models in python
Time Series Talk : ARMA Model
Autoregressive order 1 process - conditions for Stationary Covariance and Weak Dependence
What is the Vector Autoregressive (VAR) Model
Time Series Talk : ARIMA Model
What are ARIMA Models
LII Concept: Autoregressive (AR) models
Autoregressive (AR) Time Series Models
Autoregressive (AR) model: estimation and stability tests (Excel)
Vector Auto Regression : Time Series Talk
Autoregressive Models: The Yule-Walker Equations
Комментарии