filmov
tv
The SoftMax Derivative, Step-by-Step!!!
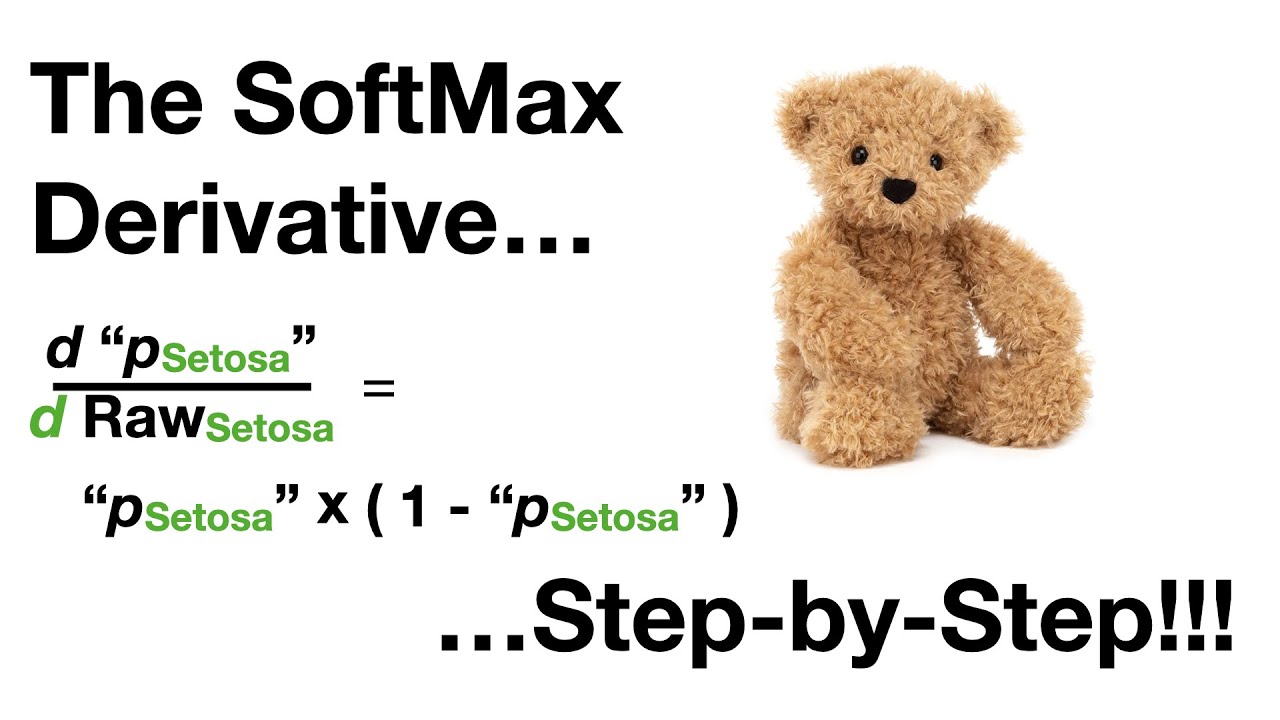
Показать описание
Here's step-by-step guide that shows you how to take the derivatives of the SoftMax function, as used as a final output layer in a Neural Networks.
For a complete index of all the StatQuest videos, check out:
If you'd like to support StatQuest, please consider...
Buying my book, The StatQuest Illustrated Guide to Machine Learning:
...or...
...a cool StatQuest t-shirt or sweatshirt:
...buying one or two of my songs (or go large and get a whole album!)
...or just donating to StatQuest!
Lastly, if you want to keep up with me as I research and create new StatQuests, follow me on twitter:
0:00 Awesome song and introduction
0:57 SoftMax derivative with respect to the output of interest
3:58 SoftMax derivative with respect to other outputs
#StatQuest #NeuralNetworks #SoftMax
For a complete index of all the StatQuest videos, check out:
If you'd like to support StatQuest, please consider...
Buying my book, The StatQuest Illustrated Guide to Machine Learning:
...or...
...a cool StatQuest t-shirt or sweatshirt:
...buying one or two of my songs (or go large and get a whole album!)
...or just donating to StatQuest!
Lastly, if you want to keep up with me as I research and create new StatQuests, follow me on twitter:
0:00 Awesome song and introduction
0:57 SoftMax derivative with respect to the output of interest
3:58 SoftMax derivative with respect to other outputs
#StatQuest #NeuralNetworks #SoftMax
The SoftMax Derivative, Step-by-Step!!!
Softmax Activation Function || Softmax Function || Quick Explained || Developers Hutt
Backpropagation for Softmax and Multi-Class Classification | Complete Mathematical Derivation
L8.8 Softmax Regression Derivatives for Gradient Descent
NN - 10 - Cross Entropy and Softmax - Derivatives
Softmax and its derivative for multi-class classification
Derivative of Sigmoid and Softmax Explained Visually
What is the derivative of the Softmax Function?
Softmax Layer from Scratch | Mathematics & Python Code
3.7 What is Softmax Activation function and how to take its Derivative or Jacobian?
Softmax Function Explained In Depth with 3D Visuals
Back propagation through Cross Entropy and Softmax
The Softmax : Data Science Basics
Mathematics: Derivative of Softmax loss function
Activation Functions - Softmax
Neural Networks Part 7: Cross Entropy Derivatives and Backpropagation
Tutorial 6-Chain Rule of Differentiation with BackPropagation
Deep Learning | Softmax Activation Function
Categorical Cross - Entropy Loss Softmax
Neural Networks Part 5: ArgMax and SoftMax
Multiclass logistic/softmax regression from scratch
Demonstration: Softmax Regression
Derivative of Cross Entropy Loss [Step By Step]
The Sigmoid Function Derivative - Step-by-step
Комментарии