filmov
tv
PAIR AI Explorables | Is the problem in the data? Examples on Fairness, Diversity, and Bias.
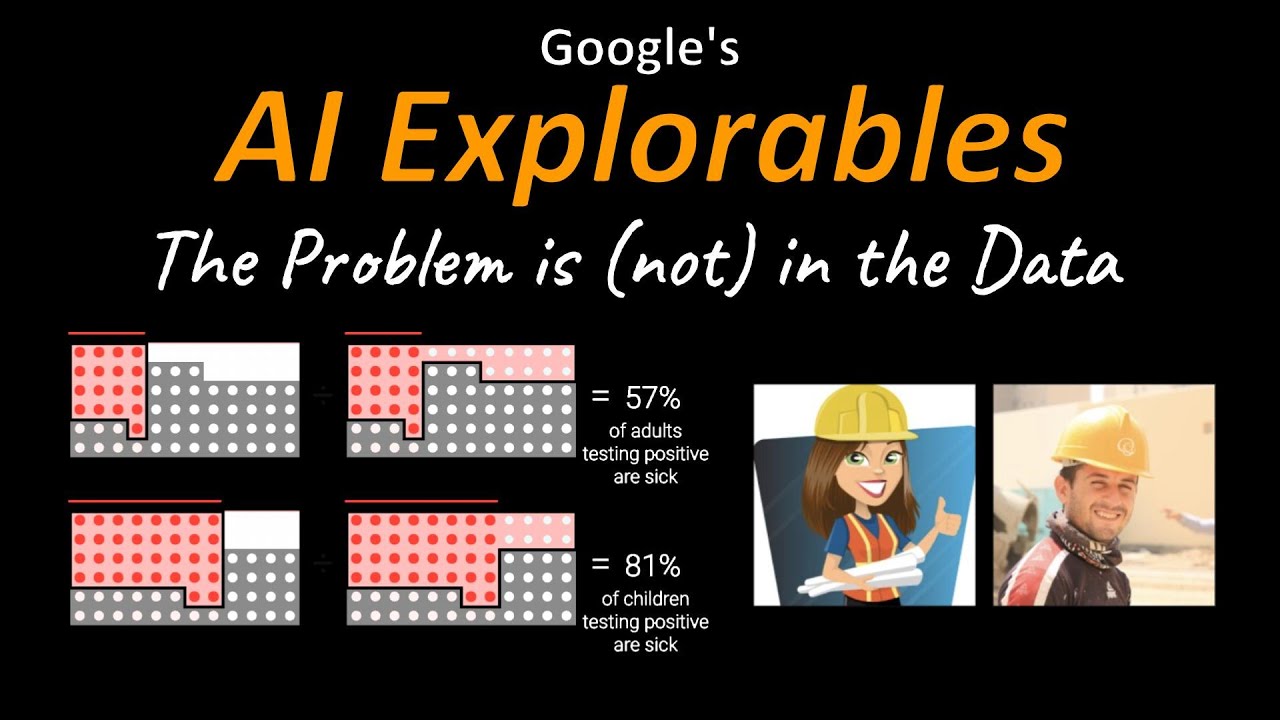
Показать описание
In the recurring debate about bias in Machine Learning models, there is a growing argument saying that "the problem is not in the data", often citing the influence of various choices like loss functions or network architecture. In this video, we take a look at PAIR's AI Explorables through the lens of whether or not the bias problem is a data problem.
OUTLINE:
0:00 - Intro & Overview
1:45 - Recap: Bias in ML
4:25 - AI Explorables
5:40 - Measuring Fairness Explorable
11:00 - Hidden Bias Explorable
16:10 - Measuring Diversity Explorable
23:00 - Conclusion & Comments
Links:
If you want to support me, the best thing to do is to share out the content :)
If you want to support me financially (completely optional and voluntary, but a lot of people have asked for this):
Bitcoin (BTC): bc1q49lsw3q325tr58ygf8sudx2dqfguclvngvy2cq
Ethereum (ETH): 0x7ad3513E3B8f66799f507Aa7874b1B0eBC7F85e2
Litecoin (LTC): LQW2TRyKYetVC8WjFkhpPhtpbDM4Vw7r9m
Monero (XMR): 4ACL8AGrEo5hAir8A9CeVrW8pEauWvnp1WnSDZxW7tziCDLhZAGsgzhRQABDnFy8yuM9fWJDviJPHKRjV4FWt19CJZN9D4n
OUTLINE:
0:00 - Intro & Overview
1:45 - Recap: Bias in ML
4:25 - AI Explorables
5:40 - Measuring Fairness Explorable
11:00 - Hidden Bias Explorable
16:10 - Measuring Diversity Explorable
23:00 - Conclusion & Comments
Links:
If you want to support me, the best thing to do is to share out the content :)
If you want to support me financially (completely optional and voluntary, but a lot of people have asked for this):
Bitcoin (BTC): bc1q49lsw3q325tr58ygf8sudx2dqfguclvngvy2cq
Ethereum (ETH): 0x7ad3513E3B8f66799f507Aa7874b1B0eBC7F85e2
Litecoin (LTC): LQW2TRyKYetVC8WjFkhpPhtpbDM4Vw7r9m
Monero (XMR): 4ACL8AGrEo5hAir8A9CeVrW8pEauWvnp1WnSDZxW7tziCDLhZAGsgzhRQABDnFy8yuM9fWJDviJPHKRjV4FWt19CJZN9D4n
Комментарии