filmov
tv
Label Noise: Ignorance is Bliss
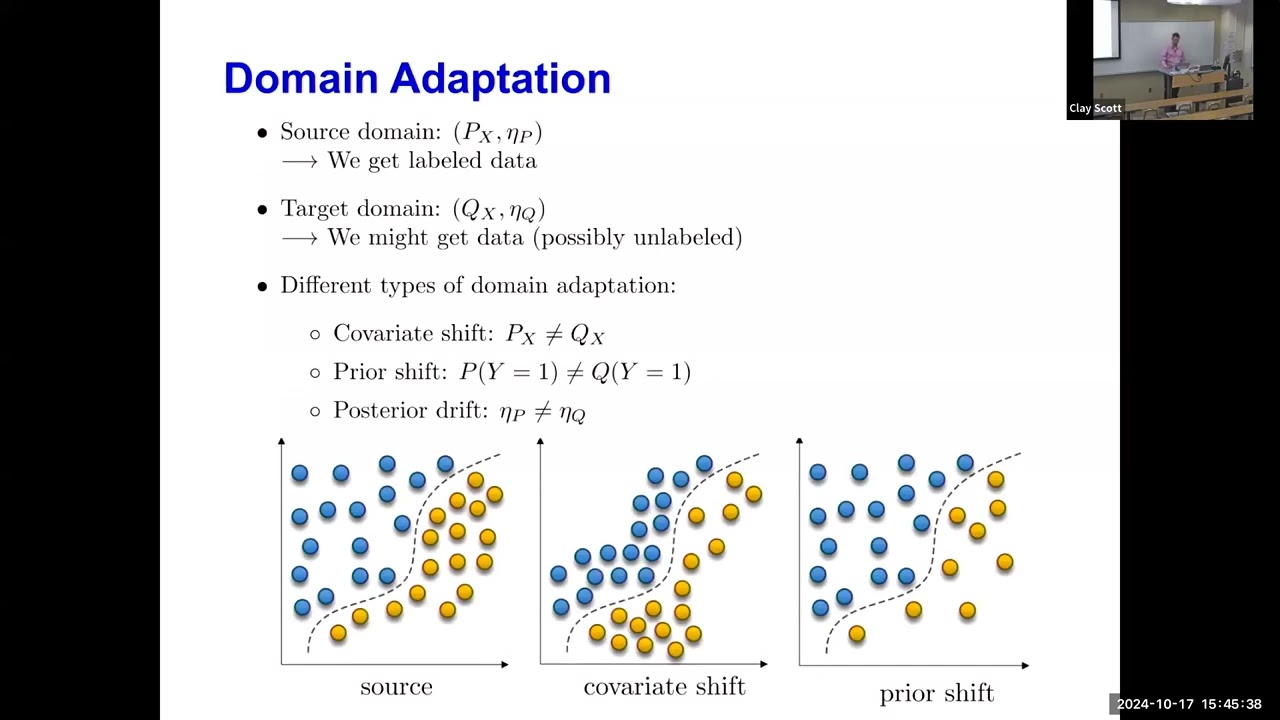
Показать описание
Clay Scott
Professor
Electrical Engineering and Computer Science
Abstract: We establish a new theoretical framework for learning under multi-class, instance-dependent label noise. At the heart of our framework is the concept of \emph{relative signal strength} (RSS), which is a point-wise measure of noisiness. Using relative signal strength, we establish nearly matching upper and lower bounds for excess risk. Our theoretical findings reveal a surprising result: the extremely simple \emph{Noise Ignorant Empirical Risk Minimization (NI-ERM)} principle, which conducts empirical risk minimization as if no label noise exists, is nearly minimax optimal. Finally, we translate these theoretical insights into practice: by using NI-ERM to fit a linear classifier on top of a self-supervised feature extractor, we achieve state-of-the-art performance on the CIFAR-N data challenge.
Bio: Clayton Scott received his PhD in Electrical Engineering from Rice University in 2004, and is currently Professor of Electrical Engineering and Computer Science at the University of Michigan. His research interests include statistical machine learning theory and algorithms, with an emphasis on nonparametric methods for supervised and unsupervised learning. He has also worked on a number of applications including brain imaging, nuclear threat detection, environmental monitoring, and computational biology. In 2010, he received the Career Award from the National Science Foundation.
Professor
Electrical Engineering and Computer Science
Abstract: We establish a new theoretical framework for learning under multi-class, instance-dependent label noise. At the heart of our framework is the concept of \emph{relative signal strength} (RSS), which is a point-wise measure of noisiness. Using relative signal strength, we establish nearly matching upper and lower bounds for excess risk. Our theoretical findings reveal a surprising result: the extremely simple \emph{Noise Ignorant Empirical Risk Minimization (NI-ERM)} principle, which conducts empirical risk minimization as if no label noise exists, is nearly minimax optimal. Finally, we translate these theoretical insights into practice: by using NI-ERM to fit a linear classifier on top of a self-supervised feature extractor, we achieve state-of-the-art performance on the CIFAR-N data challenge.
Bio: Clayton Scott received his PhD in Electrical Engineering from Rice University in 2004, and is currently Professor of Electrical Engineering and Computer Science at the University of Michigan. His research interests include statistical machine learning theory and algorithms, with an emphasis on nonparametric methods for supervised and unsupervised learning. He has also worked on a number of applications including brain imaging, nuclear threat detection, environmental monitoring, and computational biology. In 2010, he received the Career Award from the National Science Foundation.