filmov
tv
ChatGPT fails 95% of Business Use Cases
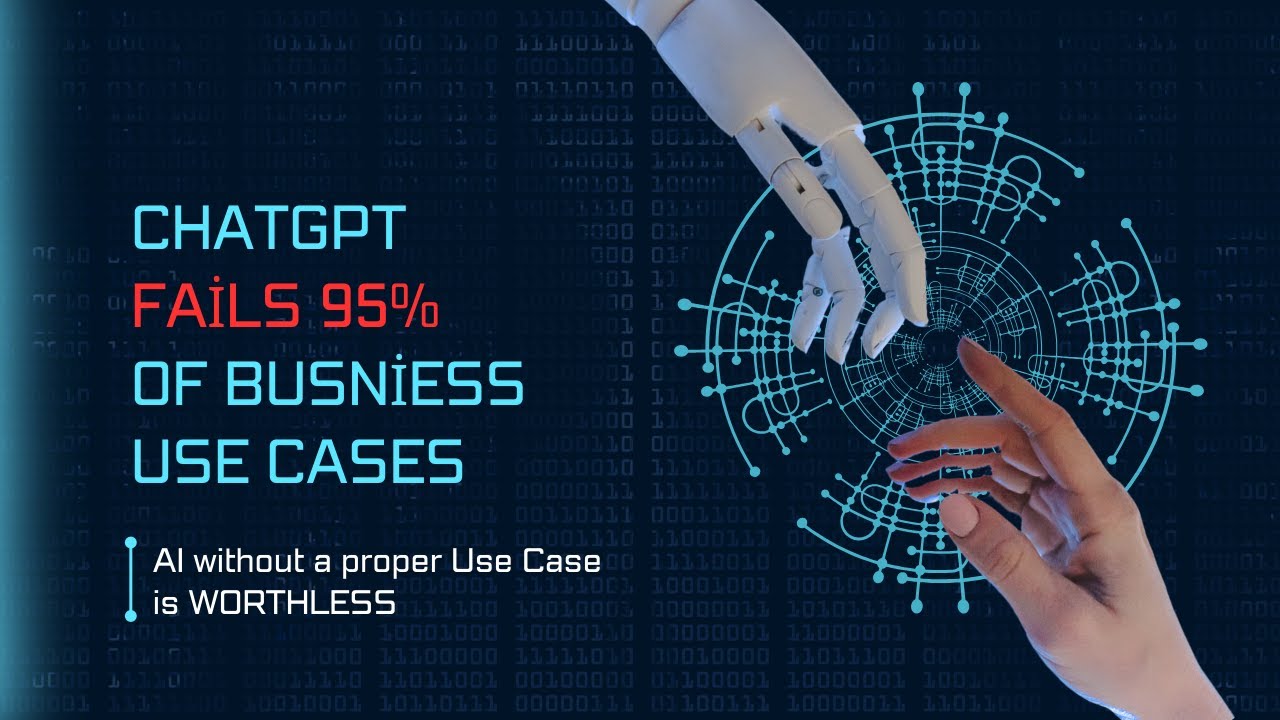
Показать описание
Instead of explaining it, here's what ChatGPT answered when prompted with the title of this video:
The assertion that "ChatGPT fails 95% of Business Use Cases" can be understood from several angles, depending on the specific business requirements, the expectations set for AI models, and the maturity of the use cases being applied. Let's break this down from a professional data science and AI/ML perspective:
1. Understanding Business Requirements and Context
Business Context: Many business use cases require a deep understanding of the industry, domain-specific knowledge, and the ability to interpret nuanced contexts. ChatGPT, being a general-purpose language model, may struggle with specialized tasks that require detailed domain expertise.
Expectation vs. Reality: Businesses might expect AI models to perform at a level that matches or exceeds human experts, particularly in complex decision-making processes. ChatGPT, however, is designed to generate human-like text based on patterns it has learned, which may not always align with the intricate needs of specific business cases.
2. Technical Limitations
Data Availability and Quality: ChatGPT's performance is heavily dependent on the data it has been trained on. If a business use case requires up-to-date or proprietary data that the model hasn't been trained on, its effectiveness can be severely limited.
Contextual Understanding: ChatGPT can sometimes struggle with maintaining long-term context, especially in complex, multi-step business processes. This can lead to incorrect or incomplete responses in use cases where sustained understanding is critical.
3. Domain-Specific AI Models vs. General-Purpose Models
Specialized Models: In many business scenarios, specialized AI models like OrYx Genie or other domain-specific models are more effective because they are trained on targeted data and fine-tuned to understand specific contexts.
Generalization: While ChatGPT can handle a wide range of tasks, it often lacks the depth required for high-stakes business decisions, which can result in suboptimal performance for most use cases.
4. Deployment and Scalability Challenges
Integration with Existing Systems: Businesses often face challenges integrating AI models like ChatGPT into existing workflows and systems. This is especially true in sectors with rigid compliance, data privacy concerns, or legacy systems that are not easily adaptable to AI technologies.
Scalability: Even when ChatGPT can address a business need, scaling that solution across an enterprise or adapting it to various departments or geographies can introduce significant challenges that may lead to failure in practice.
5. Mitigating Failures in Business Use Cases
Use of Hybrid Models: Combining general-purpose models like ChatGPT with domain-specific models, such as those found in the OrYx platform, could mitigate failures by allowing each model to contribute where it is strongest.
Human-in-the-Loop: Implementing a human-in-the-loop approach can help bridge gaps where the AI model falls short, ensuring that critical decisions are reviewed or augmented by human experts.
Customization and Fine-Tuning: Fine-tuning ChatGPT on specific business datasets can significantly improve performance, making it more aligned with the particular needs of the business.
Conclusion
While ChatGPT can be a powerful tool, it has its limitations, especially when applied to highly specialized, complex, or sensitive business use cases. For optimal results, a combination of specialized models, robust data engineering, and human oversight is often required. A deep understanding of both the model's capabilities and the specific business context is critical to overcoming the challenges and maximizing the AI's value in business applications.
The assertion that "ChatGPT fails 95% of Business Use Cases" can be understood from several angles, depending on the specific business requirements, the expectations set for AI models, and the maturity of the use cases being applied. Let's break this down from a professional data science and AI/ML perspective:
1. Understanding Business Requirements and Context
Business Context: Many business use cases require a deep understanding of the industry, domain-specific knowledge, and the ability to interpret nuanced contexts. ChatGPT, being a general-purpose language model, may struggle with specialized tasks that require detailed domain expertise.
Expectation vs. Reality: Businesses might expect AI models to perform at a level that matches or exceeds human experts, particularly in complex decision-making processes. ChatGPT, however, is designed to generate human-like text based on patterns it has learned, which may not always align with the intricate needs of specific business cases.
2. Technical Limitations
Data Availability and Quality: ChatGPT's performance is heavily dependent on the data it has been trained on. If a business use case requires up-to-date or proprietary data that the model hasn't been trained on, its effectiveness can be severely limited.
Contextual Understanding: ChatGPT can sometimes struggle with maintaining long-term context, especially in complex, multi-step business processes. This can lead to incorrect or incomplete responses in use cases where sustained understanding is critical.
3. Domain-Specific AI Models vs. General-Purpose Models
Specialized Models: In many business scenarios, specialized AI models like OrYx Genie or other domain-specific models are more effective because they are trained on targeted data and fine-tuned to understand specific contexts.
Generalization: While ChatGPT can handle a wide range of tasks, it often lacks the depth required for high-stakes business decisions, which can result in suboptimal performance for most use cases.
4. Deployment and Scalability Challenges
Integration with Existing Systems: Businesses often face challenges integrating AI models like ChatGPT into existing workflows and systems. This is especially true in sectors with rigid compliance, data privacy concerns, or legacy systems that are not easily adaptable to AI technologies.
Scalability: Even when ChatGPT can address a business need, scaling that solution across an enterprise or adapting it to various departments or geographies can introduce significant challenges that may lead to failure in practice.
5. Mitigating Failures in Business Use Cases
Use of Hybrid Models: Combining general-purpose models like ChatGPT with domain-specific models, such as those found in the OrYx platform, could mitigate failures by allowing each model to contribute where it is strongest.
Human-in-the-Loop: Implementing a human-in-the-loop approach can help bridge gaps where the AI model falls short, ensuring that critical decisions are reviewed or augmented by human experts.
Customization and Fine-Tuning: Fine-tuning ChatGPT on specific business datasets can significantly improve performance, making it more aligned with the particular needs of the business.
Conclusion
While ChatGPT can be a powerful tool, it has its limitations, especially when applied to highly specialized, complex, or sensitive business use cases. For optimal results, a combination of specialized models, robust data engineering, and human oversight is often required. A deep understanding of both the model's capabilities and the specific business context is critical to overcoming the challenges and maximizing the AI's value in business applications.