filmov
tv
Were RNNs All We Needed? (2 Oct 2024)
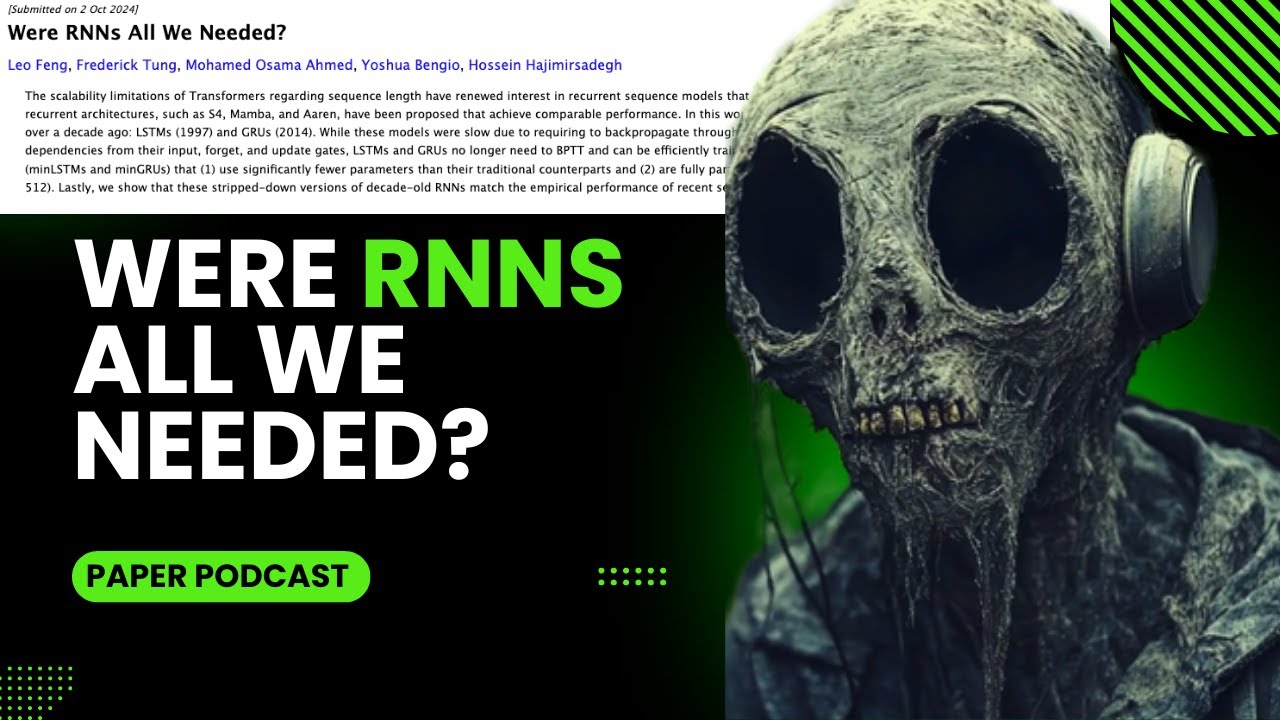
Показать описание
Title: Were RNNs All We Needed?
Date: 2 Oct 2024
Authors: Leo Feng, Frederick Tung, Mohamed Osama Ahmed, Yoshua Bengio, Hossein Hajimirsadegh
Summary
This research paper explores the potential of traditional recurrent neural networks (RNNs), specifically LSTMs and GRUs, for long-sequence tasks. The authors argue that these models, despite their historical limitations due to sequential computation and the need for backpropagation through time (BPTT), can be made highly efficient and competitive with modern sequence models like Transformers. The key innovation lies in simplifying these RNNs by removing hidden state dependencies within their gates, thereby enabling parallel training using the parallel prefix scan algorithm. This leads to significantly faster training times and improved memory efficiency while retaining comparable performance on tasks like selective copying, reinforcement learning, and language modelling. The authors conclude by posing a thought-provoking question: "Were RNNs all we needed?", highlighting the potential for these simplified RNNs to revolutionise sequence modelling.
Key Topics
RNN Efficiency, Minimal RNNs, Sequence Modelling, Parallel Scan, Empirical Performance
Date: 2 Oct 2024
Authors: Leo Feng, Frederick Tung, Mohamed Osama Ahmed, Yoshua Bengio, Hossein Hajimirsadegh
Summary
This research paper explores the potential of traditional recurrent neural networks (RNNs), specifically LSTMs and GRUs, for long-sequence tasks. The authors argue that these models, despite their historical limitations due to sequential computation and the need for backpropagation through time (BPTT), can be made highly efficient and competitive with modern sequence models like Transformers. The key innovation lies in simplifying these RNNs by removing hidden state dependencies within their gates, thereby enabling parallel training using the parallel prefix scan algorithm. This leads to significantly faster training times and improved memory efficiency while retaining comparable performance on tasks like selective copying, reinforcement learning, and language modelling. The authors conclude by posing a thought-provoking question: "Were RNNs all we needed?", highlighting the potential for these simplified RNNs to revolutionise sequence modelling.
Key Topics
RNN Efficiency, Minimal RNNs, Sequence Modelling, Parallel Scan, Empirical Performance
Were RNNs All We Needed? (Paper Explained)
Calling Out AI Hype In Research: Are RNNs All We Needed?
Gerard presents: Were RNNs All We Needed?
Were RNNs All We Needed? (2 Oct 2024)
Were RNNs All We Needed?
Were RNNs All We Needed - Google Illuminate Podcast
Recurrent Neural Networks (RNNs), Clearly Explained!!!
Were RNNs All We Needed? (Live Paper Reading)
Which career is really best? AI VS ML VS DL VS Data Science | AI vs Data science | ML vs DL #BYwB
Were RNNs All We Needed?
Were RNNs All We Needed?
Podcast: Were RNNs All We Needed?
Recurrent Neural Networks (RNN) and Long Short-Term Memory (LSTM)
Were RNNs All We Needed Paper - By Google NotebookLM
[QA] Were RNNs All We Needed?
Were RNNs All We Needed? (Feng et al., 2024)
[arxiv 2024] Were RNNs All We Needed?
Podcast: Were RNNs All We Needed 20241008
PyTorch or Tensorflow? Which Should YOU Learn!
Podcast-3: Were RNNs All We Needed?
165 - An introduction to RNN and LSTM
MIT 6.S191 (2019): Recurrent Neural Networks
What is LSTM (Long Short Term Memory)?
Lecture 10 | Recurrent Neural Networks
Комментарии