filmov
tv
Striding CUDA like i'm Johnnie Walker
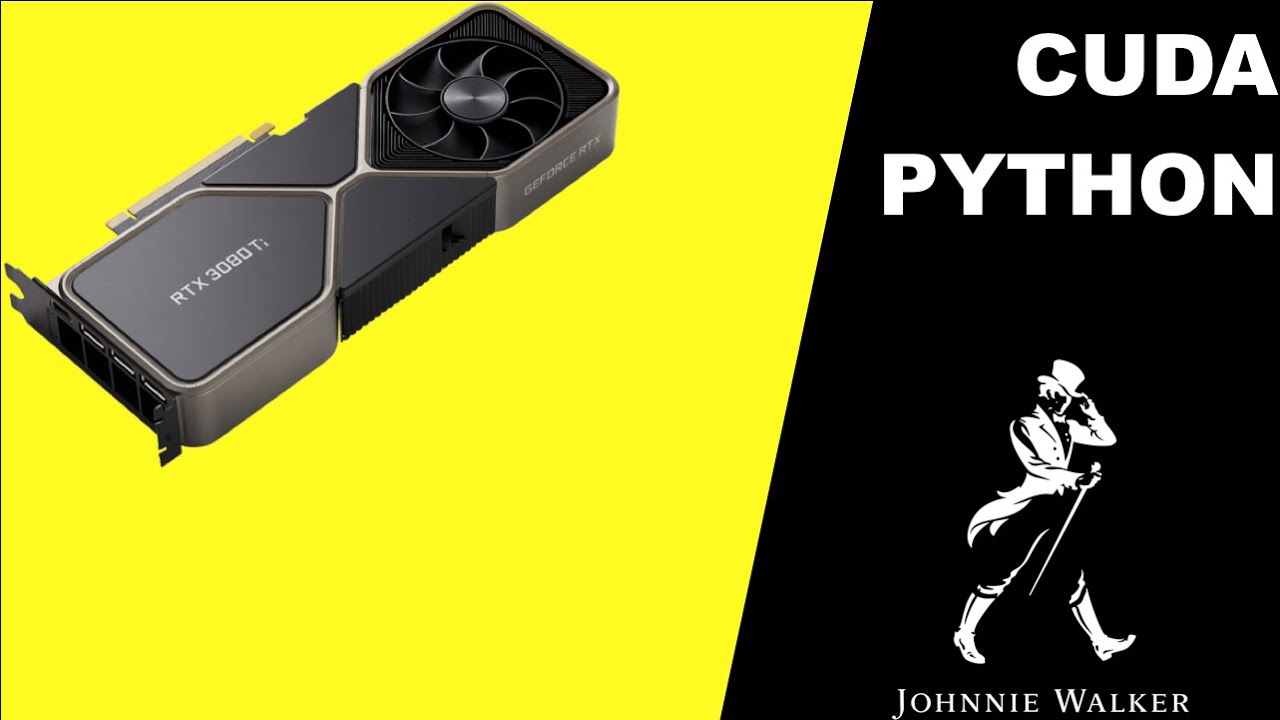
Показать описание
💡 Giveaway steps:
✅ 2. Wait for #GTC23 to start and join the Keynote livestream.
✅ 3. Attend GTC sessions (there’s really a lot of sessions going on - just pick one you’re interested in) 😄
⏱Outline:
00:00 Intro
01:45 4080 RTX Giveaway steps
02:42 Jupyter notebook preperations
02:47 GPU in use
03:27 Simple array declaration
03:43 numba's vectorize decorator
03:56 CUDA kernel
05:12 Grids and blocks
05:57 Caveat
06:27 Striding kernels
06:58 Example
07:32 Example: 1 block of 4 threads
07:39 Elements processed by each thread
08:02 Performance analysis
10:18 Outro
📚 CUDA things to know:
CUDA (Compute Unified Device Architecture) is a parallel computing platform and programming model created by NVIDIA for accelerating the computation tasks of graphics processing units (GPUs). CUDA programming is a way of programming GPUs using the CUDA programming language, which is based on the C and C++ programming languages. CUDA allows developers to offload computationally intensive tasks to the GPU, which can perform calculations in parallel with much greater efficiency than traditional CPUs. This makes it a popular choice for high-performance computing applications in a wide range of fields, including scientific computing, machine learning, computer vision, and more. To write programs using CUDA, developers use special extensions to the C and C++ programming languages that enable them to express parallelism and manage data transfers between the CPU and GPU. These extensions include special keywords and functions that allow developers to launch kernels (small, self-contained units of code that execute on the GPU) and manage memory allocation and data transfers between the CPU and GPU. In summary, CUDA programming provides a powerful way to harness the parallel computing capabilities of GPUs for high-performance computing tasks.
Now when I say “CUDA Python”, this refers to the use of the CUDA parallel computing platform and programming model with the Python programming language. This allows Python developers to harness the power of GPUs for high-performance computing tasks, without needing to learn a new programming language. CUDA is typically used with the Numba library, which allows Python functions to be compiled to CUDA kernels. Numba provides decorators that can be used to specify which functions should be compiled to run on the GPU, and which arguments should be transferred between the CPU and GPU.
With CUDA Python and Numba, Python developers can write high-performance code that can take advantage of the massively parallel nature of GPUs. This makes it a popular choice for scientific computing and data analysis, where performance is critical for working with large datasets or running complex simulations. It's important to note that while CUDA can provide significant performance gains, it's not always the best choice for all tasks. It's important to consider factors such as the size of the problem, the available hardware, and the specific requirements of the application before deciding whether to use CUDA Python.
CUDA is fast because it offloads computation-intensive tasks to the highly parallel architecture of modern graphics processing units (GPUs). GPUs were originally designed to render complex 3D graphics, but they are also highly effective at performing parallel computations. A typical CPU has a small number of cores optimized for serial processing, whereas a GPU has thousands of smaller, more power-efficient cores that can perform many calculations simultaneously. This allows a GPU to perform many more computations per second than a CPU, especially for tasks that can be highly parallelized, such as image and video processing, machine learning, and scientific simulations. CUDA is a parallel computing platform and programming model developed by NVIDIA that allows developers to write code in a way that can be executed on the GPU. This means that developers can take advantage of the GPU's parallel processing capabilities to accelerate their computations. By utilizing CUDA, applications can see significant speedups, sometimes by orders of magnitude, compared to traditional CPU-based computations. In summary, CUDA is fast because it leverages the parallel architecture of GPUs, which can perform many computations simultaneously, resulting in significant speedups for a variety of computational tasks.
🙏🏻 Credits:
Dan G. for directing
Moe D. for editing
Samer S. for brainstorming
Bensound for audio
This video is created under a creative common's license.
#gtc23 #cuda #stride
✅ 2. Wait for #GTC23 to start and join the Keynote livestream.
✅ 3. Attend GTC sessions (there’s really a lot of sessions going on - just pick one you’re interested in) 😄
⏱Outline:
00:00 Intro
01:45 4080 RTX Giveaway steps
02:42 Jupyter notebook preperations
02:47 GPU in use
03:27 Simple array declaration
03:43 numba's vectorize decorator
03:56 CUDA kernel
05:12 Grids and blocks
05:57 Caveat
06:27 Striding kernels
06:58 Example
07:32 Example: 1 block of 4 threads
07:39 Elements processed by each thread
08:02 Performance analysis
10:18 Outro
📚 CUDA things to know:
CUDA (Compute Unified Device Architecture) is a parallel computing platform and programming model created by NVIDIA for accelerating the computation tasks of graphics processing units (GPUs). CUDA programming is a way of programming GPUs using the CUDA programming language, which is based on the C and C++ programming languages. CUDA allows developers to offload computationally intensive tasks to the GPU, which can perform calculations in parallel with much greater efficiency than traditional CPUs. This makes it a popular choice for high-performance computing applications in a wide range of fields, including scientific computing, machine learning, computer vision, and more. To write programs using CUDA, developers use special extensions to the C and C++ programming languages that enable them to express parallelism and manage data transfers between the CPU and GPU. These extensions include special keywords and functions that allow developers to launch kernels (small, self-contained units of code that execute on the GPU) and manage memory allocation and data transfers between the CPU and GPU. In summary, CUDA programming provides a powerful way to harness the parallel computing capabilities of GPUs for high-performance computing tasks.
Now when I say “CUDA Python”, this refers to the use of the CUDA parallel computing platform and programming model with the Python programming language. This allows Python developers to harness the power of GPUs for high-performance computing tasks, without needing to learn a new programming language. CUDA is typically used with the Numba library, which allows Python functions to be compiled to CUDA kernels. Numba provides decorators that can be used to specify which functions should be compiled to run on the GPU, and which arguments should be transferred between the CPU and GPU.
With CUDA Python and Numba, Python developers can write high-performance code that can take advantage of the massively parallel nature of GPUs. This makes it a popular choice for scientific computing and data analysis, where performance is critical for working with large datasets or running complex simulations. It's important to note that while CUDA can provide significant performance gains, it's not always the best choice for all tasks. It's important to consider factors such as the size of the problem, the available hardware, and the specific requirements of the application before deciding whether to use CUDA Python.
CUDA is fast because it offloads computation-intensive tasks to the highly parallel architecture of modern graphics processing units (GPUs). GPUs were originally designed to render complex 3D graphics, but they are also highly effective at performing parallel computations. A typical CPU has a small number of cores optimized for serial processing, whereas a GPU has thousands of smaller, more power-efficient cores that can perform many calculations simultaneously. This allows a GPU to perform many more computations per second than a CPU, especially for tasks that can be highly parallelized, such as image and video processing, machine learning, and scientific simulations. CUDA is a parallel computing platform and programming model developed by NVIDIA that allows developers to write code in a way that can be executed on the GPU. This means that developers can take advantage of the GPU's parallel processing capabilities to accelerate their computations. By utilizing CUDA, applications can see significant speedups, sometimes by orders of magnitude, compared to traditional CPU-based computations. In summary, CUDA is fast because it leverages the parallel architecture of GPUs, which can perform many computations simultaneously, resulting in significant speedups for a variety of computational tasks.
🙏🏻 Credits:
Dan G. for directing
Moe D. for editing
Samer S. for brainstorming
Bensound for audio
This video is created under a creative common's license.
#gtc23 #cuda #stride
Комментарии