filmov
tv
Distributed Trajectory Optimization for Legged Locomotion -- ACC 2020
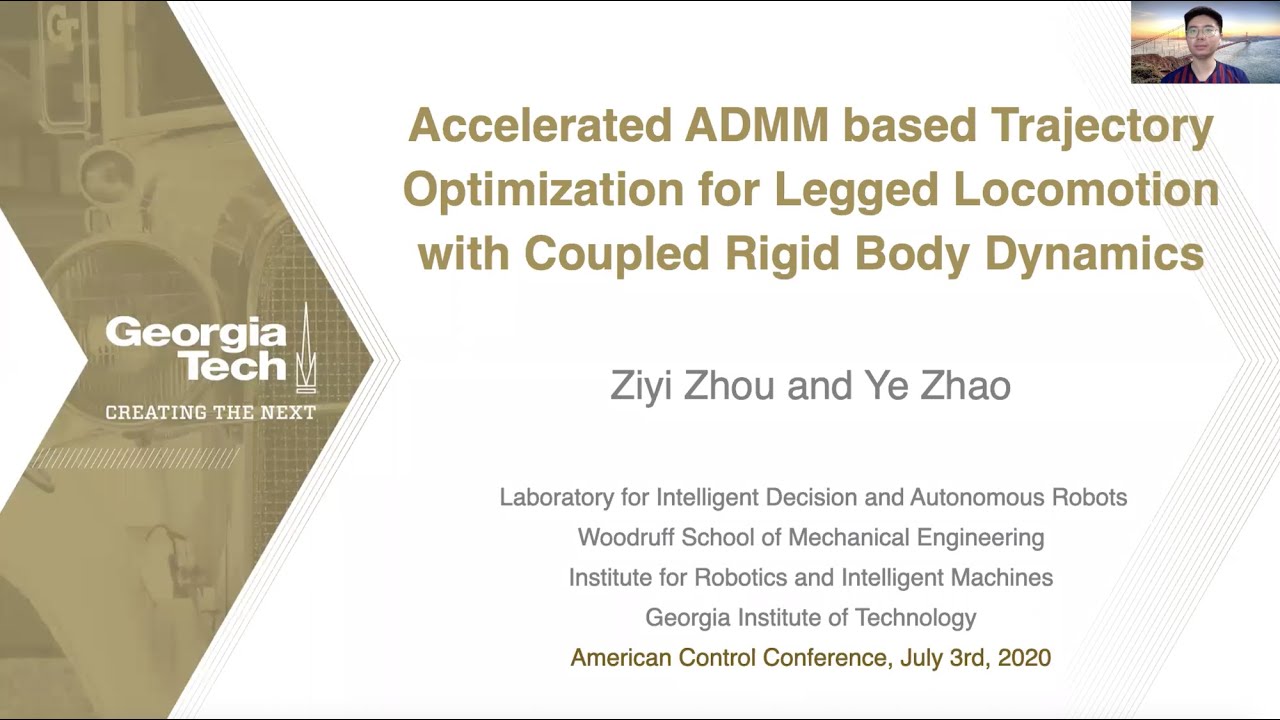
Показать описание
This work is presented by LIDAR student Ziyi Zhou.
Trajectory optimization is becoming increasingly powerful in addressing motion planning problems of underactuated robotic systems. Numerous prior studies solve such a class of large non-convex optimal control problems in a hierarchical fashion. However, numerical accuracy issues are prone to occur when one uses a full-order model to track reference trajectories generated from a reduced-order model. This study investigates an approach of Alternating Direction Method of Multipliers (ADMM) and proposes a new splitting scheme for legged locomotion problems. Rigid body dynamics constraints and other general constraints such as box and cone constraints are decomposed to multiple sub-problems in a principled manner. The resulting multi-block ADMM framework enables us to leverage the efficiency of an unconstrained optimization method–Differential Dynamical Programming–to iteratively solve the optimizations using centroidal and whole-body models. Furthermore, we propose a Stage-wise Accelerated ADMM with over-relaxation and varying-penalty schemes to improve the overall convergence rate. We evaluate and validate the performance of the proposed ADMM algorithm on a car-parking example and a bipedal locomotion problem over rough terrains.
Trajectory optimization is becoming increasingly powerful in addressing motion planning problems of underactuated robotic systems. Numerous prior studies solve such a class of large non-convex optimal control problems in a hierarchical fashion. However, numerical accuracy issues are prone to occur when one uses a full-order model to track reference trajectories generated from a reduced-order model. This study investigates an approach of Alternating Direction Method of Multipliers (ADMM) and proposes a new splitting scheme for legged locomotion problems. Rigid body dynamics constraints and other general constraints such as box and cone constraints are decomposed to multiple sub-problems in a principled manner. The resulting multi-block ADMM framework enables us to leverage the efficiency of an unconstrained optimization method–Differential Dynamical Programming–to iteratively solve the optimizations using centroidal and whole-body models. Furthermore, we propose a Stage-wise Accelerated ADMM with over-relaxation and varying-penalty schemes to improve the overall convergence rate. We evaluate and validate the performance of the proposed ADMM algorithm on a car-parking example and a bipedal locomotion problem over rough terrains.