filmov
tv
The multi-armed bandit model in reinforcement learning
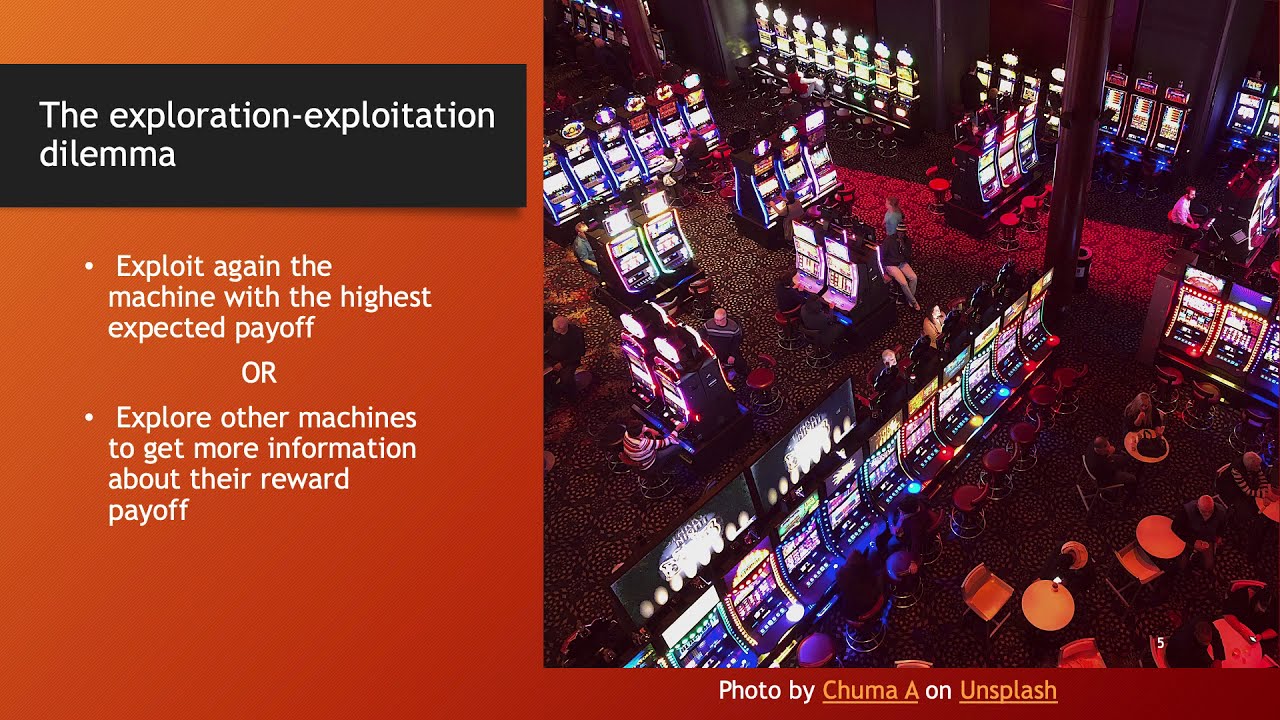
Показать описание
The K-armed bandit is a classic reinforcement learning problem that exemplifies the exploration–exploitation tradeoff dilemma. The name of the problem comes from the example of a gambler in a casino, playing a row of slot machines. Each machine has one lever to pull and will give some reward specific to that machine, with the payoff scheme evolving in time. The gambler has to decide on his strategy: keep playing the machine that paid the best reward so far or explore another machine for a while. The objective of the gambler is to maximise his rewards over a sequence of actions.