filmov
tv
Genetic susceptibility for brain disorders, clinical disease trajectories & end-stage neuropathology
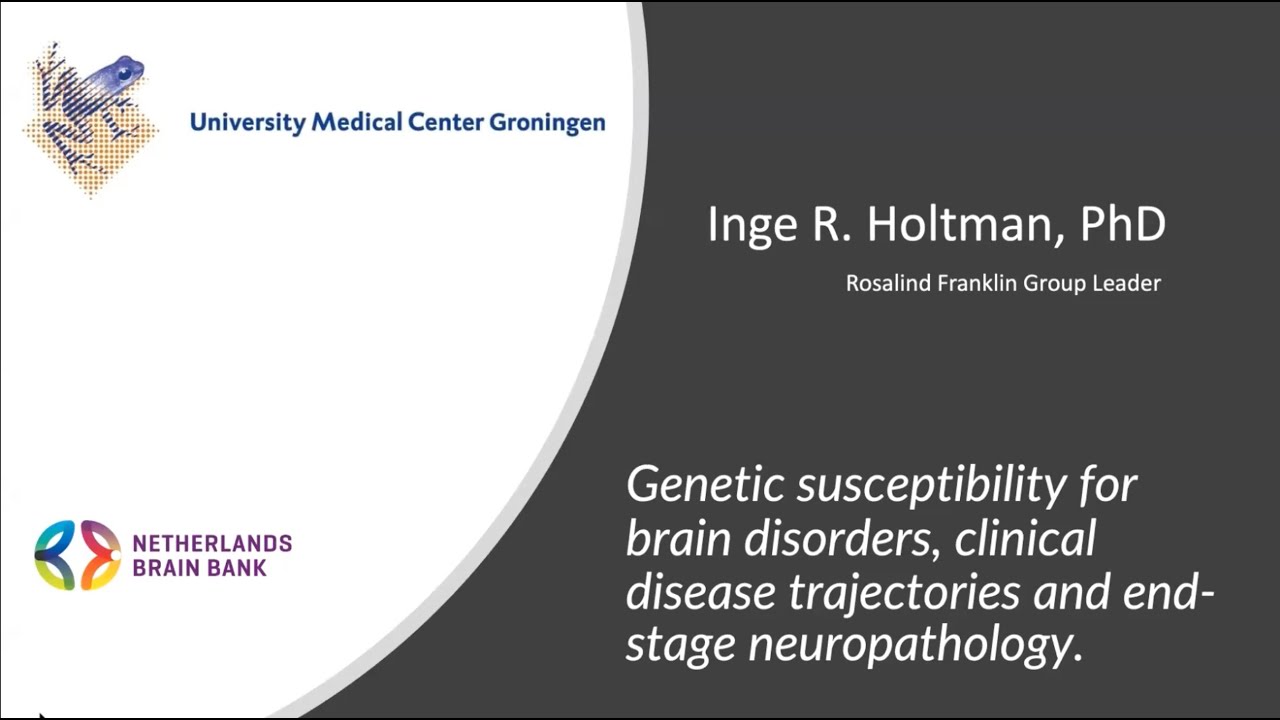
Показать описание
This talk was held on 14th February 2023, and was presented by Dr Inge Holtman from the University of Groningen.
Full title: The Netherlands Neurogenetics Database: Genetic susceptibility for brain disorders, clinical disease trajectories and end-stage neuropathology.
Abstract: The brain is a highly complex organ that consists of many intricately linked substructures and cell types and is susceptible to a wide range of brain disorders. Many brain disorders show considerable overlap in clinical manifestations, genetic risk factors, and pathophysiological mechanisms, suggesting a complex relationship between clinical symptoms, neuropathology, and genetic susceptibility both within and between clinical diagnosis. Brain diseases are often wrongly diagnosed, as up to 30% show a mismatch between a clinical and neuropathologically based diagnosis. A better delineation of the relationships between these disorders might enable improved diagnosis, better prognosis, and personalized therapeutic interventions. This clearly illustrates the need for a cross-diagnostic, data-driven approach. To this end, we recently established the Netherlands Neurogenetics Database which aims to integrate the extensive clinical, and neuropathological data of the Netherlands Brain Bank (NBB) with genetics data from a large number of donors.
The first aim of this project is to convert a large corpus of clinical history data, consisting of medical summaries made by medically trained staff of the NBB, into standardized clinical disease trajectories. For this, we aimed to identify and define clinical signs and symptoms associated with brain disorders. We identified 90 signs and symptoms, divided over 14 groupings, within 5 domains, such as ‘memory impairment’ and ‘hallucinations’. Different Natural Language Processing (NLP) models, including Google BERT, were trained and optimized with Optuna to identify these signs and symptoms in individual sentences in the clinical history data. After Optuna optimization, BERT and T5 were able to reliably identify most signs and symptoms with a micro-F1 score above 0.9. Out of the 90 attributes, 81 reached an F1 score of 0.8 or higher in the best performing BERT model. The final model was used to predict signs and symptoms in the full corpus. We subsequently compiled the attributes according to the main neuropathological diagnoses from the NBB and identified clear disease-associated signs and symptom profiles, supporting the validity of this approach. In addition, we were able to identify signs and symptoms that differ between disease subtypes, which might help to distinguish brain-disorder subtypes. Finally, these clinical attributes, in conjunction with polygenic risk scores were used to model the temporal aspects of disease progression, and to train machine learning models to more reliably predict neuropathological diagnosis.
This video is part of a series of biweekly neurogenomics seminars organised by Dr Sarah Marzi.
Full title: The Netherlands Neurogenetics Database: Genetic susceptibility for brain disorders, clinical disease trajectories and end-stage neuropathology.
Abstract: The brain is a highly complex organ that consists of many intricately linked substructures and cell types and is susceptible to a wide range of brain disorders. Many brain disorders show considerable overlap in clinical manifestations, genetic risk factors, and pathophysiological mechanisms, suggesting a complex relationship between clinical symptoms, neuropathology, and genetic susceptibility both within and between clinical diagnosis. Brain diseases are often wrongly diagnosed, as up to 30% show a mismatch between a clinical and neuropathologically based diagnosis. A better delineation of the relationships between these disorders might enable improved diagnosis, better prognosis, and personalized therapeutic interventions. This clearly illustrates the need for a cross-diagnostic, data-driven approach. To this end, we recently established the Netherlands Neurogenetics Database which aims to integrate the extensive clinical, and neuropathological data of the Netherlands Brain Bank (NBB) with genetics data from a large number of donors.
The first aim of this project is to convert a large corpus of clinical history data, consisting of medical summaries made by medically trained staff of the NBB, into standardized clinical disease trajectories. For this, we aimed to identify and define clinical signs and symptoms associated with brain disorders. We identified 90 signs and symptoms, divided over 14 groupings, within 5 domains, such as ‘memory impairment’ and ‘hallucinations’. Different Natural Language Processing (NLP) models, including Google BERT, were trained and optimized with Optuna to identify these signs and symptoms in individual sentences in the clinical history data. After Optuna optimization, BERT and T5 were able to reliably identify most signs and symptoms with a micro-F1 score above 0.9. Out of the 90 attributes, 81 reached an F1 score of 0.8 or higher in the best performing BERT model. The final model was used to predict signs and symptoms in the full corpus. We subsequently compiled the attributes according to the main neuropathological diagnoses from the NBB and identified clear disease-associated signs and symptom profiles, supporting the validity of this approach. In addition, we were able to identify signs and symptoms that differ between disease subtypes, which might help to distinguish brain-disorder subtypes. Finally, these clinical attributes, in conjunction with polygenic risk scores were used to model the temporal aspects of disease progression, and to train machine learning models to more reliably predict neuropathological diagnosis.
This video is part of a series of biweekly neurogenomics seminars organised by Dr Sarah Marzi.