filmov
tv
Yiping Lu - Optimization Of Neural Network: A Continuous Depth Limit Point Of View And Beyond
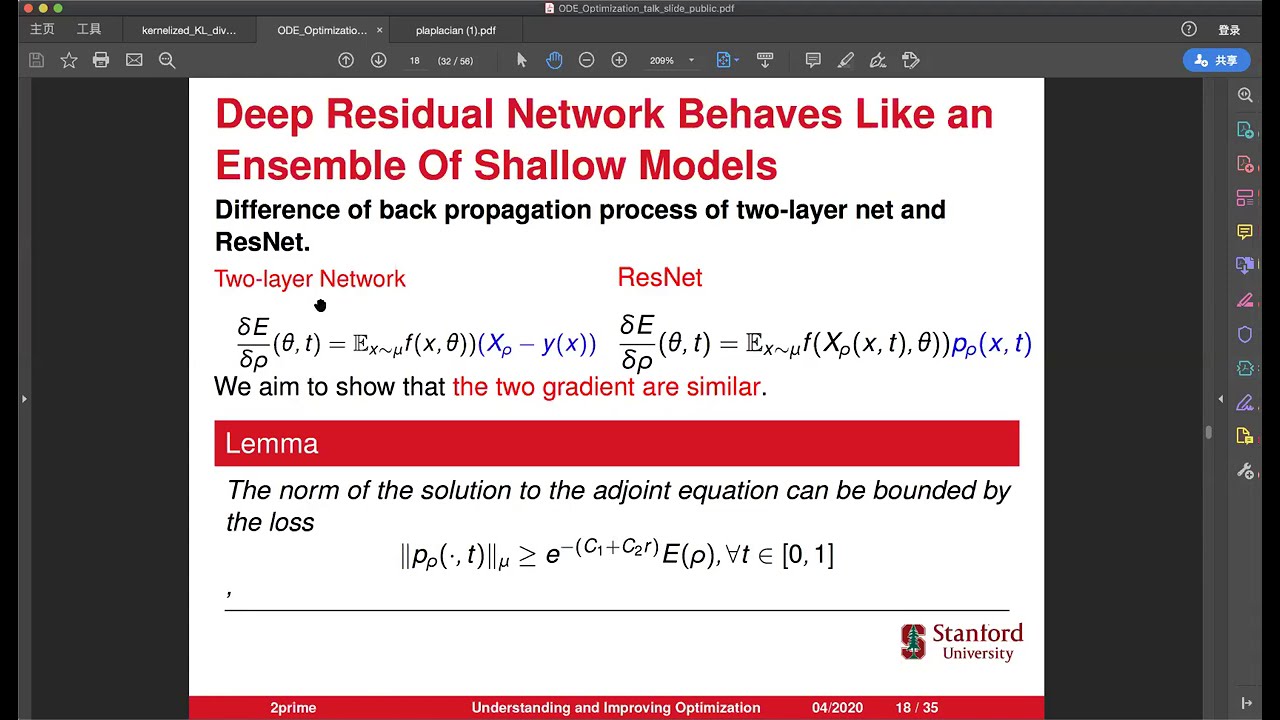
Показать описание
Presentation given by Yiping Lu on August 14th at the "Thematic Day on Continuous ResNets" of the one world seminar on the mathematics of machine learning on the topic "A Continuous Depth Limit Point Of View And Beyond".
Abstract: To understand the mystery of neural networks, we introduce a brand new perspective on the understanding of deep architectures which consider ODE as the continuum limits of deep neural networks (neural networks with infinite layers). In this framework, optimizing a deep neural network becomes an optimal control problem and from this perspective, the optimality condition (i.e. the Pontryagin’s Maximum Principle) can derive the original backpropagation algorithm. We adopt this view both on theoretical understanding and empirical algorithm design for neural networks. Theoretically, we propose a new limiting ODE model of ResNets using mean-field analysis, which enjoys a good landscape in the sense that every local minimizer is global. Empirically, we adopt this frame to design algorithms to design fast adversarial training. This talk is based on our publication at Neurips2019 and ICML2020, joint work with Bin Dong, Zhanxing Zhu, Lexing Ying, Jianfeng Lu and et al. Here is a summarizing video.
Abstract: To understand the mystery of neural networks, we introduce a brand new perspective on the understanding of deep architectures which consider ODE as the continuum limits of deep neural networks (neural networks with infinite layers). In this framework, optimizing a deep neural network becomes an optimal control problem and from this perspective, the optimality condition (i.e. the Pontryagin’s Maximum Principle) can derive the original backpropagation algorithm. We adopt this view both on theoretical understanding and empirical algorithm design for neural networks. Theoretically, we propose a new limiting ODE model of ResNets using mean-field analysis, which enjoys a good landscape in the sense that every local minimizer is global. Empirically, we adopt this frame to design algorithms to design fast adversarial training. This talk is based on our publication at Neurips2019 and ICML2020, joint work with Bin Dong, Zhanxing Zhu, Lexing Ying, Jianfeng Lu and et al. Here is a summarizing video.