filmov
tv
SANE 2015: Pablo Sprechmann (NYU) on Deep Learning for Solving Inverse Problems
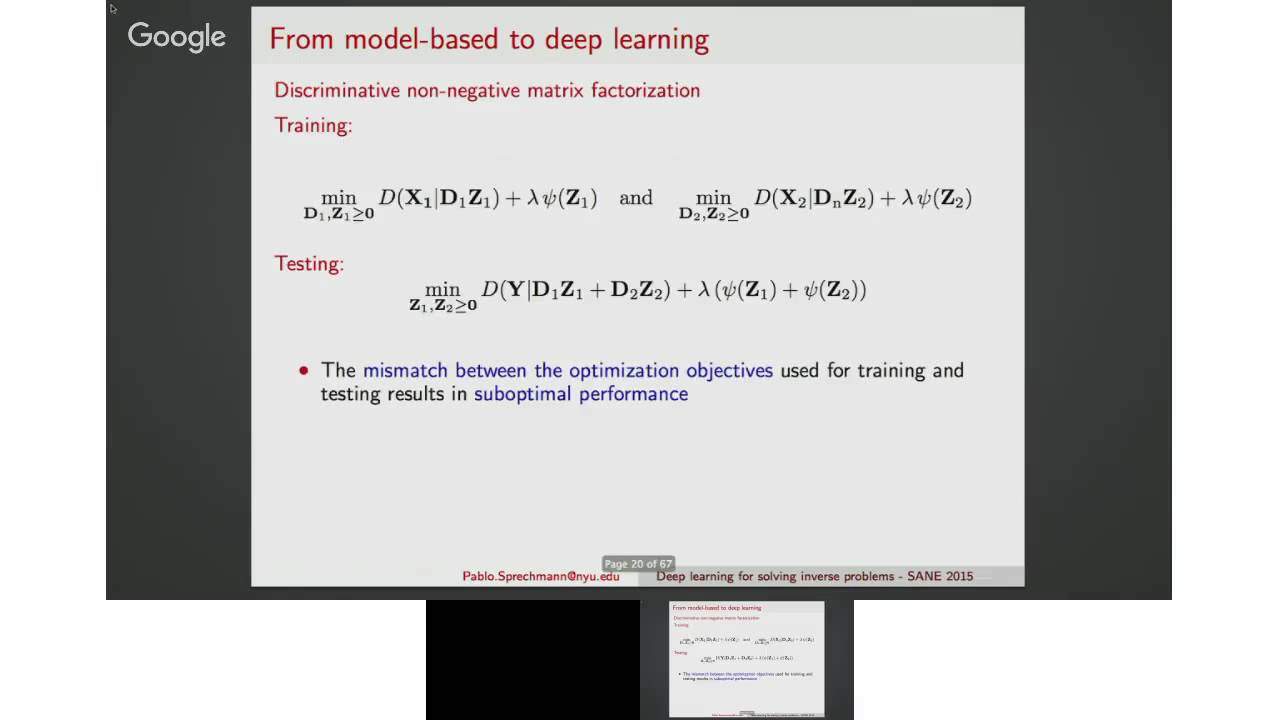
Показать описание
raditionally, approaches to solve inverse problems in image and signal processing construct models with appropriate priors to regularize the signal estimation. Motivated by the advance of deep learning techniques in classification and recognition tasks, in recent years there has been a shift from this traditional setting towards approaching inverse problems as data-driven non-linear regressions. Given a set of pairs of observed and target signals, these approaches aim at learning a parametric mapping given by a generic neural network architecture with enough capacity to perform the regression. In the first part of the talk I will review this transition in the context of single channel source separation and enhancement. A natural connection between model-based approaches (based on non-negative matrix factorization) and regression-based approaches using deep neural networks will be presented. Some reasons that might explain why this method is particularly successful in this setting will be exposed and, based on this observations, an approach using multi-scale convolutional networks will be discussed. Despite their great success, these techniques face some limitations when the inverse problem at hand becomes more ill-conditioned. For instance, these limitations emerge when the goal is not just to the enhance the observed signal, but to produce a high fidelity version of it, which oftentimes involves multi-modality and uncertainty. The second part of the talk provides an analysis of these limitations and presents a possible alternative to mitigate them by establishing connections with recent work in computer vision.