filmov
tv
Difference-in-differences | Synthetic Control | Causal Inference in Data Science Part 2
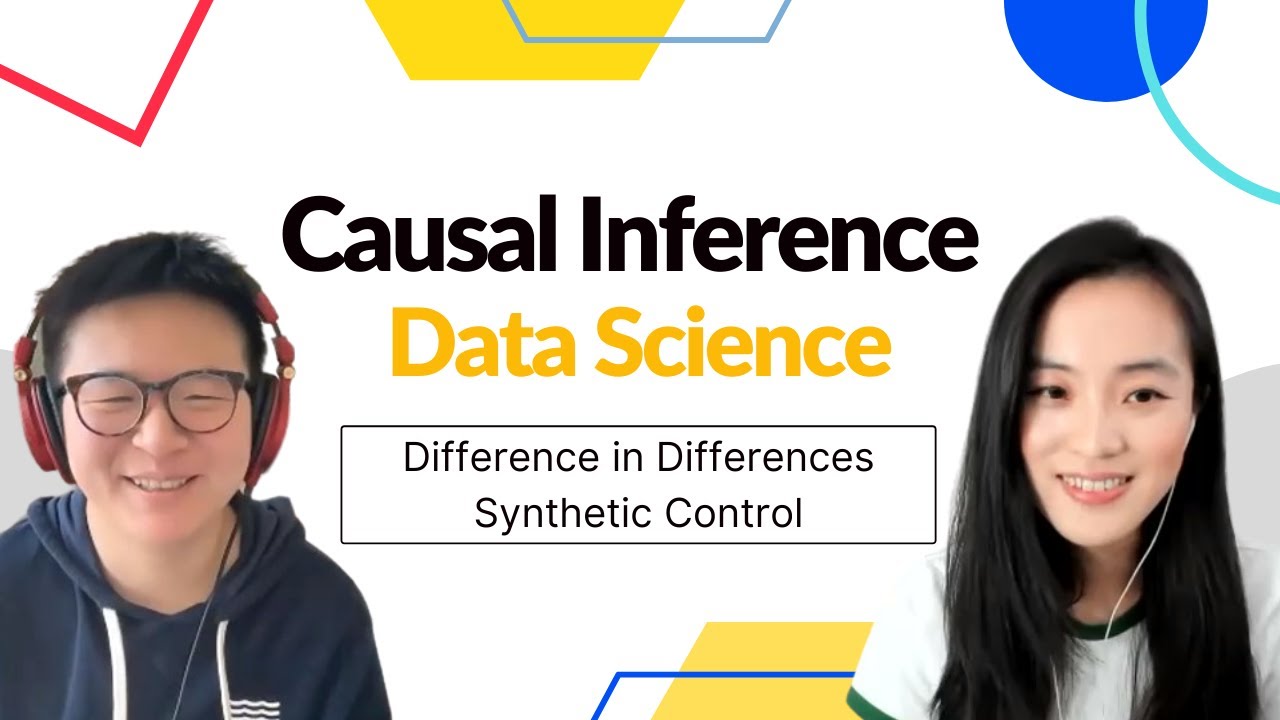
Показать описание
This video is the second part of our mini course on application of Causal Inference in data science. We are going to discuss what kind of methods you can use to do Causal Inference with just a few treated units. Two methods are introduced: difference-in-differences and synthetic control.
📚 Resources recommended by Yuan
- Abadie, A. (2021). Using synthetic controls: Feasibility, data requirements, and methodological aspects. Journal of Economic Literature, 59(2), 391-425.
🟢Get all my free data science interview resources
// Comment
Got any questions? Something to add?
Write a comment below to chat.
// Let's connect on LinkedIn:
====================
Contents of this video:
====================
00:00 How to measure COVID's Impact on the Economy
08:13 Difference-in-Differences
14:47 Synthetic Control
24:17 Summary
📚 Resources recommended by Yuan
- Abadie, A. (2021). Using synthetic controls: Feasibility, data requirements, and methodological aspects. Journal of Economic Literature, 59(2), 391-425.
🟢Get all my free data science interview resources
// Comment
Got any questions? Something to add?
Write a comment below to chat.
// Let's connect on LinkedIn:
====================
Contents of this video:
====================
00:00 How to measure COVID's Impact on the Economy
08:13 Difference-in-Differences
14:47 Synthetic Control
24:17 Summary
Difference-in-differences | Synthetic Control | Causal Inference in Data Science Part 2
Synthetic Control: Math Explained
Susan Athey: Synthetic Difference in Differences
Synthetic Control
Synthetic Control Explained
Synthetic control methods: Introduction & overview of recent developments - Dr Carl Bonander
Staggered Treatment in Difference-in-Differences (The Effects, Videos on Causality, Ep 56)
Lecture 14: Canonical Research Designs II: Event Studies, Synthetic Control + Synthetic DinD
Università degli studi di Firenze
Synthetic Controls: A Tool That's Changing Economics
Basic Difference-in-Differences Method (DID) | Estimation Methods | Stata Tutorials Topic 42
Difference-in-differences methods
Andrew Gelman: Better than difference-in-differences
10-3: Synthetic Control Method
[1/2] Synthetic Control approach to measuring economic spillovers
9.1 - Difference-in-Differences Motivation and Preliminaries
9.4 - Problems with Difference-in-Differences
Nick Jones, Sam Barrows: Uber's Synthetic Control | PyData Amsterdam 2019
Econ 480 - Lecture 8: Difference in Differences
Synthetic Controls for Causal Inference in Investing
Causal Inference: A Simple Difference-in-Difference Model
2021, Methods Lecture, Alberto Abadie 'Synthetic Controls: Methods and Practice'
Episode 27. Causality (2): Synthetic Control
Data Science Research Methods: Differences-in-differences estimation
Комментарии