filmov
tv
Machine Learning | The Vapnik-Chervonenkis Dimension
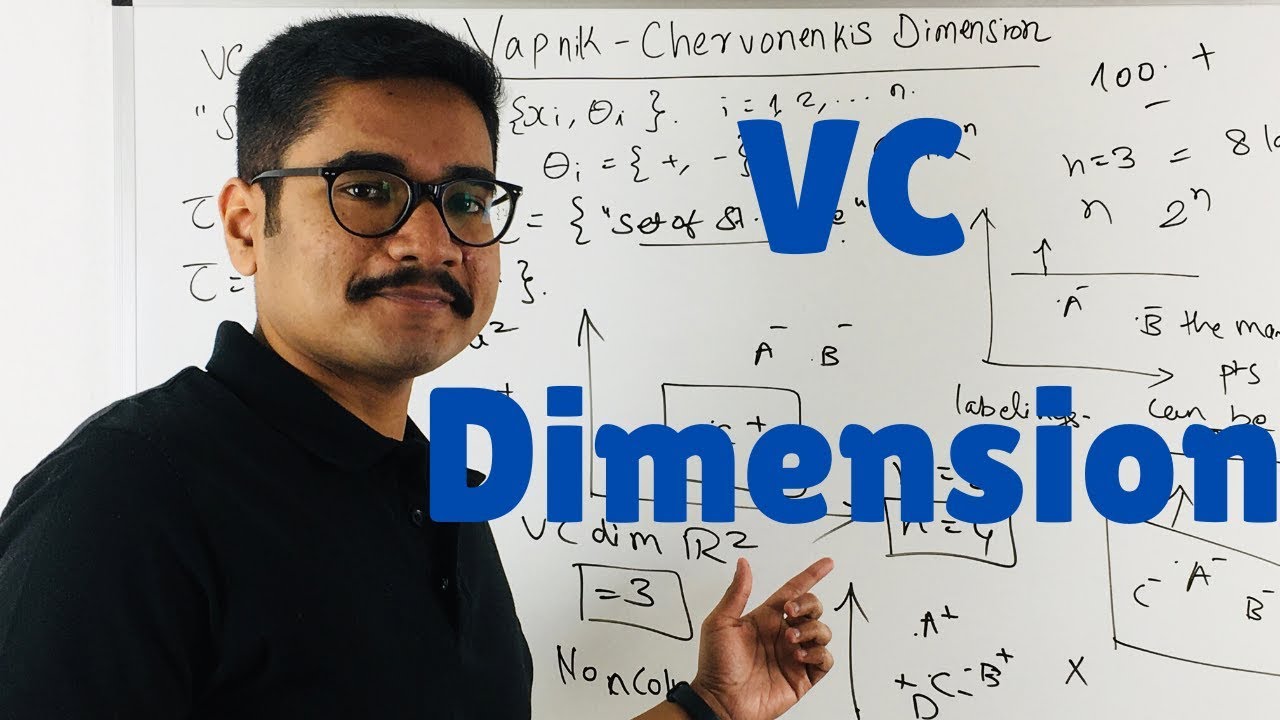
Показать описание
In Vapnik–Chervonenkis theory, the Vapnik–Chervonenkis (VC) dimension is a measure of the capacity (complexity, expressive power, richness, or flexibility) of a space of functions that can be learned by a statistical classification algorithm. #MachineLearning #VCDimension
Machine Learning | The Vapnik-Chervonenkis Dimension
Vapnik-Chervonenkis Dimension: How it Impacts Model Performance | Machine Learning | ThinkUnderstand
Vapnik Chervonenkis Dimension | VC Dimension | Solved Example in Machine Learning by Mahesh Huddar
VC Dimension - Model Building and Validation
What is VC Dimension | Example vc dimension of Line Hypothesis class
Understanding VC Dimension(Vapnik Chervonenkis Dimension) - Simplified Explanation for Beginners
Vc dimension and PAC
Vapnik - Chervonenkis (VC) Dimension-Supervised Learning-Machine Learning -Unit-1- 15A05706
VC dimension
Model Complexity and VC Dimension
The Roots of AI: VC Dimension (1971)
Complete Statistical Theory of Learning (Vladimir Vapnik) | MIT Deep Learning Series
Vladimir Vapnik: Statistical Learning | Lex Fridman Podcast #5
Machine Learning Class: VC Dimension: Part I
Vapnik–Chervonenkis(VC) Dimension | Productionization and Deployment of ML Models | EP #9
The Machine Learning Maverick: Cracking the Code with Vladimir Vapnik
06-e LFD: The Vapnik-Chervonenkis (VC) error bound.
PAC Learning and VC Dimension
module-1-Vapnik-Chervonenkis (VC)dimension
Probably Approximately Correct Learning-PAC Learning-Supervised Learning-Machine Learning-15A05706
ee53 lec60 VC dimension
A simple and gentle tutorial on Vapnik-Chervonenkis dimension part 2 of 2
VC of Finite H - Georgia Tech - Machine Learning
[W6-1] PAC learning and VC dimension
Комментарии