filmov
tv
A Finite Game of Infinite Rounds #SoME2
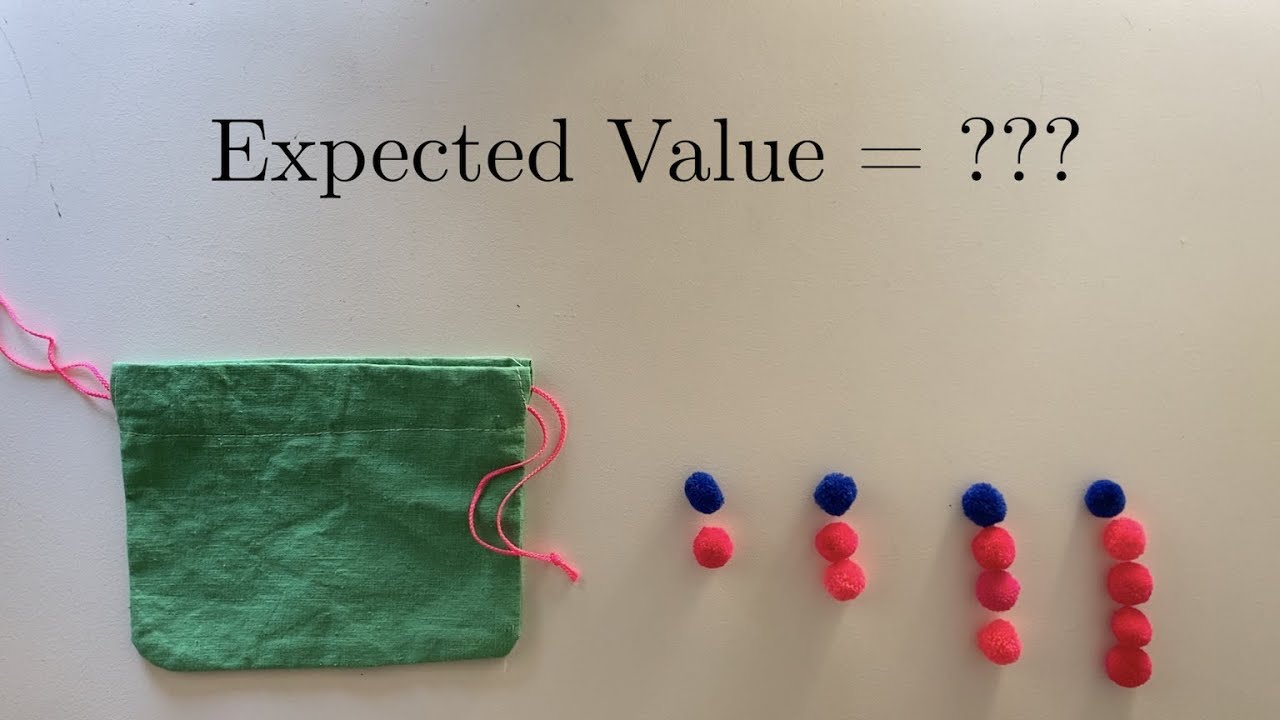
Показать описание
A short video about a random variable with no expected value. Made for the Summer of Maths Exposition 2.
0:00 Let's play a game
2:33 A better-behaved example
4:49 Working through the maths
7:08 Does the game always finish?
9:34 Discussion, and another example
11:04 A challenge problem
Correction: the sum at 4:32 should be P(1)+2P(2)+3P(3)+…
Some extra details I found later after some discussion in the comments:
The Cauchy distribution has a cool property that intuitively explains why it behaves so weirdly: the probability distribution of the average of a number independent Cauchy-distributed variables is the exact same Cauchy distribution! No matter how many runs you average, the distribution doesn't narrow, making the Cauchy distribution a counterexample to the central limit theorem.
Why do the simulations at the start of the video seem to go up logarithmically? Let's say you run N games and average the results. The mean is infinite, but we could shoddily get around this by assuming no game runs for K rounds or longer, the probability of which is (1-1/K)^N. Intuitively K should vary with N, so let's fix this to some chosen threshold probability p (e.g. p=0.05) and rearrange to get K=1/(1-p^(1/N)). We can now calculate the expected value only summing up to the K-th term, and after approximating the harmonic numbers with a logarithm and using a small Taylor expansion I got an expected value of ln(N) - ln(-ln(p)) + γ - 1. So yes, by some weird metric it is logarithmic with the number of games! However I should mention that I actually rerecorded the programs a couple of times before I got results that fit with the flow of the video; in reality the average tends to jump around a lot more.
0:00 Let's play a game
2:33 A better-behaved example
4:49 Working through the maths
7:08 Does the game always finish?
9:34 Discussion, and another example
11:04 A challenge problem
Correction: the sum at 4:32 should be P(1)+2P(2)+3P(3)+…
Some extra details I found later after some discussion in the comments:
The Cauchy distribution has a cool property that intuitively explains why it behaves so weirdly: the probability distribution of the average of a number independent Cauchy-distributed variables is the exact same Cauchy distribution! No matter how many runs you average, the distribution doesn't narrow, making the Cauchy distribution a counterexample to the central limit theorem.
Why do the simulations at the start of the video seem to go up logarithmically? Let's say you run N games and average the results. The mean is infinite, but we could shoddily get around this by assuming no game runs for K rounds or longer, the probability of which is (1-1/K)^N. Intuitively K should vary with N, so let's fix this to some chosen threshold probability p (e.g. p=0.05) and rearrange to get K=1/(1-p^(1/N)). We can now calculate the expected value only summing up to the K-th term, and after approximating the harmonic numbers with a logarithm and using a small Taylor expansion I got an expected value of ln(N) - ln(-ln(p)) + γ - 1. So yes, by some weird metric it is logarithmic with the number of games! However I should mention that I actually rerecorded the programs a couple of times before I got results that fit with the flow of the video; in reality the average tends to jump around a lot more.
Комментарии