filmov
tv
MLOps Coffee Sessions #6 // Continuous Integration for ML // Featuring Elle O'Brien
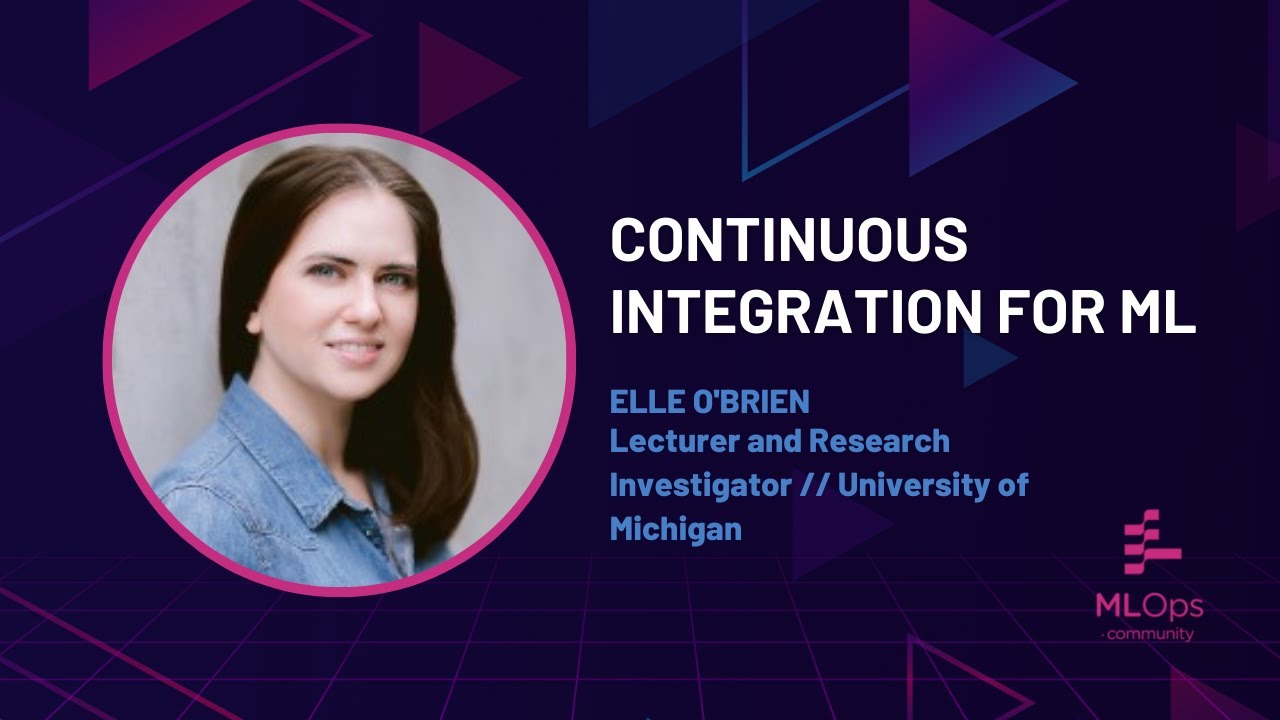
Показать описание
David & Elle talk about how one of the staples of DevOps, the practice of continuous integration, can work for machine learning. Continuous integration is a tried-and-true method for speeding up development cycles and rapidly releasing software- an area where data science and ML could use some help. Making continuous integration work for ML has been challenging in the past, and we chat about new open-source tools and approaches in the Git ecosystem for leveling up development processes with big models and datasets.
|| Highlights ||
What is continuous integration and why should ML/data science teams know about it?
Why ML projects tend to fall short of DevOps best practices, like frequent check-ins and testing
How we're dealing with obstacles to get continuous integration working for ML
Also, some fun chat about how data science roles are changing and how MLOps skills fit into the data science toolkit!
--------------- ✌️Connect With Us ✌️ -------------
Follow us on Twitter: @mlopscommunity
Timestamps:
[00:00] Intro to the discussion and to Elle O'Brien
[01:13] AI comedy writing collective
[02:32] How did Elle get into ML?
[04:43] Transition from academe to industry
[07:30] Good foundation in statistics goes a long way in data science
[09:24] Hard to quantify the value of research
[11:35] Great place for opportunities
[14:00] What is continuous integration?
[17:29] What is continuous delivery?
[21:23] Useful tools for CICD
[27:12] Integration of tools
[31:39] Why is CICD hard in ML?
[34:18] CICD in models system
[37:43] Complexity in ML codes
[39:18] What is CML?
[41:32] Data remote
[43:16] Challenge of CI in ML
[45:05] CI metrics in ML
[46:46] Visualization in CML
[50:07] CICD video series
[52:15] Starting in ML
[58:45] Takeaway from Elle
|| Highlights ||
What is continuous integration and why should ML/data science teams know about it?
Why ML projects tend to fall short of DevOps best practices, like frequent check-ins and testing
How we're dealing with obstacles to get continuous integration working for ML
Also, some fun chat about how data science roles are changing and how MLOps skills fit into the data science toolkit!
--------------- ✌️Connect With Us ✌️ -------------
Follow us on Twitter: @mlopscommunity
Timestamps:
[00:00] Intro to the discussion and to Elle O'Brien
[01:13] AI comedy writing collective
[02:32] How did Elle get into ML?
[04:43] Transition from academe to industry
[07:30] Good foundation in statistics goes a long way in data science
[09:24] Hard to quantify the value of research
[11:35] Great place for opportunities
[14:00] What is continuous integration?
[17:29] What is continuous delivery?
[21:23] Useful tools for CICD
[27:12] Integration of tools
[31:39] Why is CICD hard in ML?
[34:18] CICD in models system
[37:43] Complexity in ML codes
[39:18] What is CML?
[41:32] Data remote
[43:16] Challenge of CI in ML
[45:05] CI metrics in ML
[46:46] Visualization in CML
[50:07] CICD video series
[52:15] Starting in ML
[58:45] Takeaway from Elle
Комментарии