filmov
tv
Landing an ML Job- secrets from technical recruiters
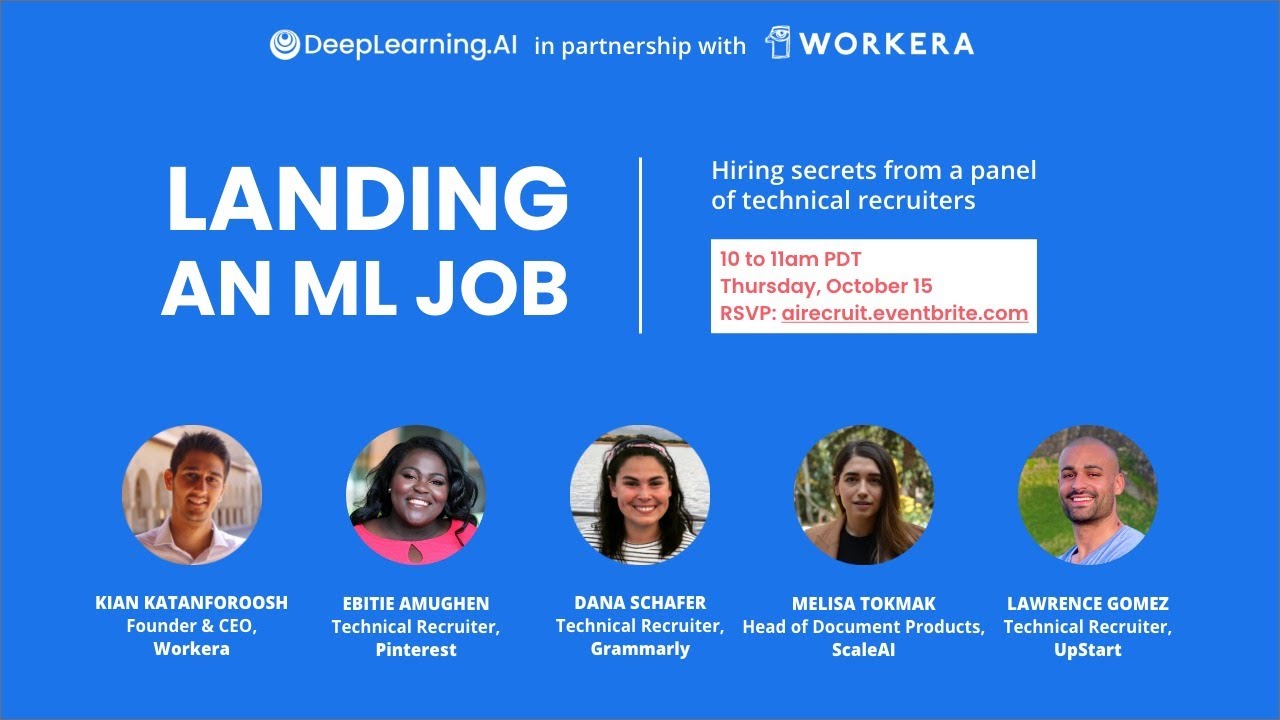
Показать описание
Welcome to Landing an ML Job- a virtual Learner Community Event hosted by DeepLearning.AI and Workera! A panel of technical recruiters will be sharing suggestions on potential career paths as well as tips and best practices for interview prep.
Agenda: PDT (*subject to change)
MC: Sandhya Simhan, Director of Marketing, DeepLearning.AI
5mins: Opening speech: Kian Katanforoosh, Founder & CEO, Workera
40mins: Panel discussion:
Moderator: Kian Katanforoosh, Founder & CEO, Workera
Panelists:
-Ebitie Amughan, Technical Recruiter, Pinterest
-Lawrence Gomez, Technical Recruiter, Upstart
-Dana Schafer, Technical Recruiter, Grammarly
-Melisa Tokmak, Head of Document Products, ScaleAI
15mins: Q&A. We will be taking questions from our learners in the Slack community. If you’re currently taking the AI for Medicine, NLP or GANs Specializations, check your Coursera platform classroom for the invitation link if you haven’t joined the respective Slack workspaces.
To learn more about us,
Agenda: PDT (*subject to change)
MC: Sandhya Simhan, Director of Marketing, DeepLearning.AI
5mins: Opening speech: Kian Katanforoosh, Founder & CEO, Workera
40mins: Panel discussion:
Moderator: Kian Katanforoosh, Founder & CEO, Workera
Panelists:
-Ebitie Amughan, Technical Recruiter, Pinterest
-Lawrence Gomez, Technical Recruiter, Upstart
-Dana Schafer, Technical Recruiter, Grammarly
-Melisa Tokmak, Head of Document Products, ScaleAI
15mins: Q&A. We will be taking questions from our learners in the Slack community. If you’re currently taking the AI for Medicine, NLP or GANs Specializations, check your Coursera platform classroom for the invitation link if you haven’t joined the respective Slack workspaces.
To learn more about us,
Landing an ML Job- secrets from technical recruiters
Secret to Landing a Software Engineering Job
Landing a Job in AI: Hiring Secrets from Technical Recruiters
Mastering Your Job Search Secrets to Landing AI and ML Jobs
Journey to Landing a Machine Learning Job in the US
Crack the Code: Insider Secrets to Landing Your Dream Data Science Job | Newton School
Landing the Perfect AI Engineering Role
FASTEST Way to Learn Data Science and ACTUALLY Get a Job
Forget about Applying for Jobs on LinkedIn or Indeed and Do This Instead
Landing interviews can be hard - Learn How To Land Your Dream Data Scientist Job In Top MNCs 🏆
Ever wonder how deep a tattoo penetrates your skin?!😱 #mommymakeover #liposuction #tummytuck
what it’s like to work at GOOGLE…
Air hostesses trying to close door 😅 #shorts
Unlocking New Job Opportunities: The Secret to Landing Your Dream Job
How to break into quant trading (as a trader)
Mr. Robot Sucks
Breaking Into Data Science : Secrets to Landing Your Dream Job! | Rosha Pokharel, PhD
How I Taught Myself How To Code And Landed A Job In Six Weeks
Best Internship Job Search Strategies That Landed At Apple
Pilot shows off the impact of flying at 9.5G 😅 #shorts #funny #gforce
How to NOT Fail a Technical Interview
Pavel Pleskov: Tips on landing a remote DSML job at a startup
2-Minute Rule to Learn Coding - Atomic Habits
ANYONE can Crack Coding Interviews by Doing THIS
Комментарии