filmov
tv
tinyML Asia 2021 Video Poster: Bird Hotspots: A tinyML acoustic classification system for...
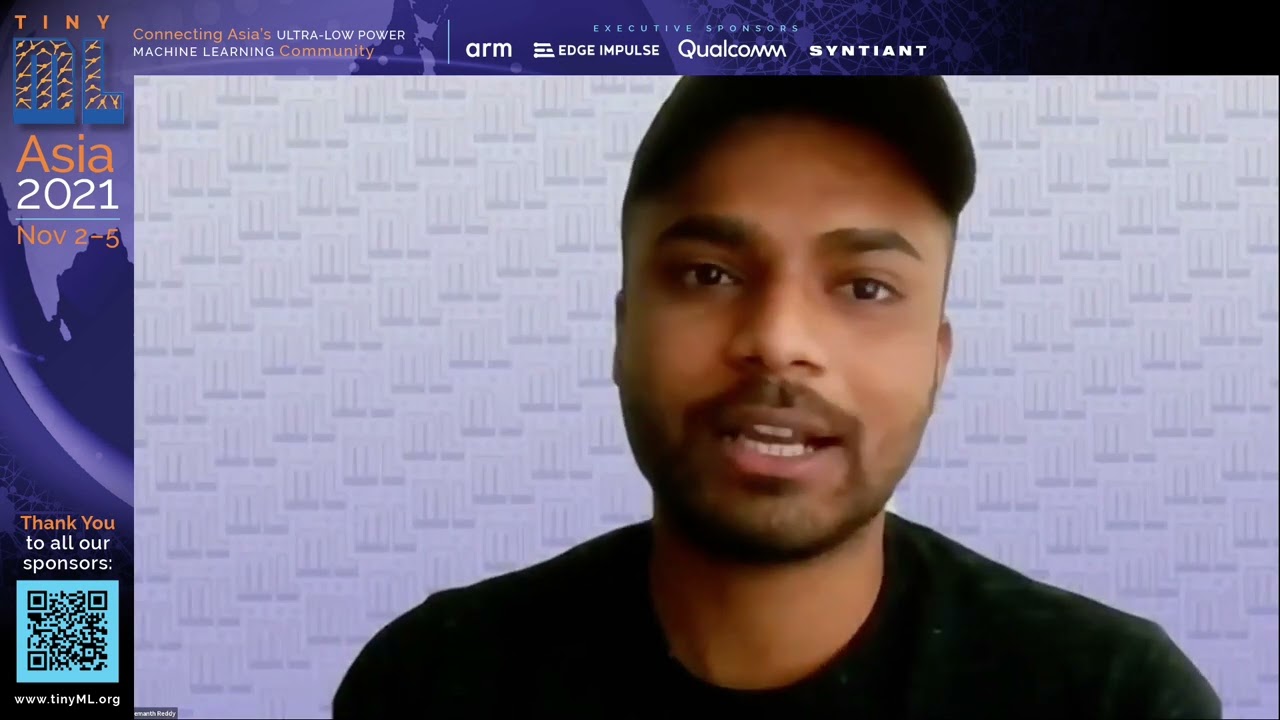
Показать описание
tinyML Asia 2021
Video Poster
Bird Hotspots: A tinyML acoustic classification system for ecological insights
Hemanth Reddy SABBELLA, Research Assistant at NeuRonICS lab, Indian Institute of Science (IISc)
The global bird distribution and abundance are reported by birdwatchers manually going from place to place to e-bird community using a smartphone application. Creating chronological hotspots across landscapes can help to automate the identification of birds and map relevant spaces for birds. In this video poster, we present an in-house developed, low-power neuromorphic acoustic classifier system that uses a novel in-filter computing framework leveraging neuromorphic cochlea as an audio frontend. The framework uses an acoustic feature extractor based on Cascade of Asymmetric Resonators with Inner Hair Cells (CAR-IHC) that can act as SVM (Support Vector Machine) kernel, resulting in template-based SVM. The template SVM classifier is designed to be computationally efficient and can run on resource-constrained hardware. For hardware integration, we developed an easy-to-deploy framework to port this classifier into battery-powered, low-power microcontrollers. The complete system is demonstrated in real-time to detect and log bird species and their occurrences running on ARM Cortex M4 processor consuming 1.6mA of mean current. It is estimated to last for at least 2 months for detecting multiple bird species on 3 AA batteries and could be optimized to achieve backup durations up to 1-year. The logged data is used to make chronological hotspots of bird occurrences on google maps which could help understand valuable information towards species conservation.
Video Poster
Bird Hotspots: A tinyML acoustic classification system for ecological insights
Hemanth Reddy SABBELLA, Research Assistant at NeuRonICS lab, Indian Institute of Science (IISc)
The global bird distribution and abundance are reported by birdwatchers manually going from place to place to e-bird community using a smartphone application. Creating chronological hotspots across landscapes can help to automate the identification of birds and map relevant spaces for birds. In this video poster, we present an in-house developed, low-power neuromorphic acoustic classifier system that uses a novel in-filter computing framework leveraging neuromorphic cochlea as an audio frontend. The framework uses an acoustic feature extractor based on Cascade of Asymmetric Resonators with Inner Hair Cells (CAR-IHC) that can act as SVM (Support Vector Machine) kernel, resulting in template-based SVM. The template SVM classifier is designed to be computationally efficient and can run on resource-constrained hardware. For hardware integration, we developed an easy-to-deploy framework to port this classifier into battery-powered, low-power microcontrollers. The complete system is demonstrated in real-time to detect and log bird species and their occurrences running on ARM Cortex M4 processor consuming 1.6mA of mean current. It is estimated to last for at least 2 months for detecting multiple bird species on 3 AA batteries and could be optimized to achieve backup durations up to 1-year. The logged data is used to make chronological hotspots of bird occurrences on google maps which could help understand valuable information towards species conservation.