filmov
tv
'Algorithmic Discrimination' 💻#BigData #AI #Algorithms #Equality#CathyONeil #WMD #Shorts #foryou
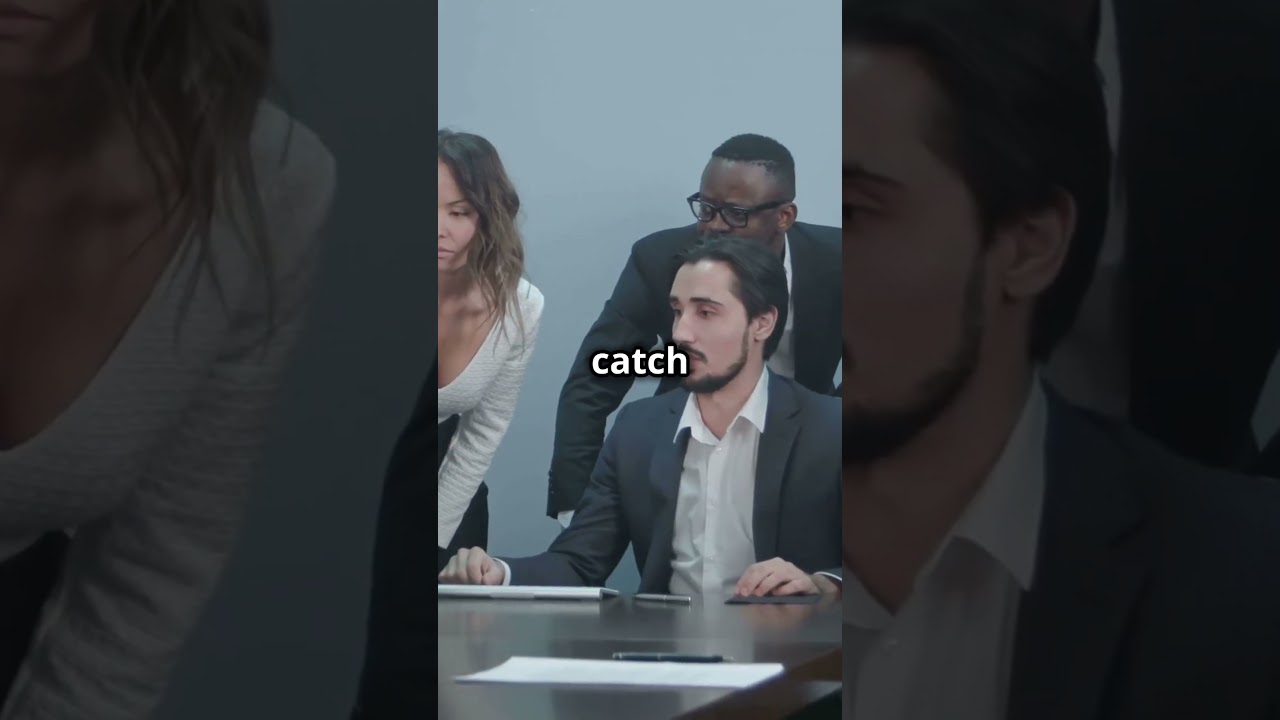
Показать описание
📄 Chapter 2: “Hiring by Algorithm”
In this chapter, Cathy O'Neil uncovers how algorithms are widely used in hiring processes but are often far from fair. Companies use these data-driven models to screen applicants, but rather than being neutral, these algorithms can reinforce biases and discrimination.
🔍 Bias in Data: Algorithms often use historical data, which can reflect past inequalities. For instance, if a company’s previous hires were predominantly from elite schools or specific backgrounds, the algorithm will favor similar profiles, perpetuating the same biases.
🚫 No Accountability: If a person is rejected due to an algorithm, they rarely know why. The system is opaque—applicants aren’t given feedback or a chance to improve, and companies rarely check the fairness of their algorithms.
📉 Cycle of Disadvantage: The chapter explains how these algorithms can create a cycle of disadvantage, where marginalized groups are continually overlooked, worsening inequality.
💼 Impact on Jobs: This system can particularly harm those who don’t fit the “ideal” profile—like people from less prestigious universities or those with gaps in their résumés. The result? Qualified candidates are often excluded for reasons unrelated to their actual ability to perform the job.
🤖 The Illusion of Objectivity: O'Neil stresses that just because an algorithm is mathematical doesn’t mean it’s free from human bias. In fact, algorithms can often hide the deepest inequalities under the guise of objectivity.
👉 Curious how these algorithms shape your future? Watch the full breakdown and see how data might be holding you back.
#BigData #AI #Algorithms #Equality #WMD #CathyONeil #WeaponsOfMathDestruction #YouTubeShorts #TechForGood #shorts #ytshorts #foryou #fyp #facts #youtube #motivation #youtuber
In this chapter, Cathy O'Neil uncovers how algorithms are widely used in hiring processes but are often far from fair. Companies use these data-driven models to screen applicants, but rather than being neutral, these algorithms can reinforce biases and discrimination.
🔍 Bias in Data: Algorithms often use historical data, which can reflect past inequalities. For instance, if a company’s previous hires were predominantly from elite schools or specific backgrounds, the algorithm will favor similar profiles, perpetuating the same biases.
🚫 No Accountability: If a person is rejected due to an algorithm, they rarely know why. The system is opaque—applicants aren’t given feedback or a chance to improve, and companies rarely check the fairness of their algorithms.
📉 Cycle of Disadvantage: The chapter explains how these algorithms can create a cycle of disadvantage, where marginalized groups are continually overlooked, worsening inequality.
💼 Impact on Jobs: This system can particularly harm those who don’t fit the “ideal” profile—like people from less prestigious universities or those with gaps in their résumés. The result? Qualified candidates are often excluded for reasons unrelated to their actual ability to perform the job.
🤖 The Illusion of Objectivity: O'Neil stresses that just because an algorithm is mathematical doesn’t mean it’s free from human bias. In fact, algorithms can often hide the deepest inequalities under the guise of objectivity.
👉 Curious how these algorithms shape your future? Watch the full breakdown and see how data might be holding you back.
#BigData #AI #Algorithms #Equality #WMD #CathyONeil #WeaponsOfMathDestruction #YouTubeShorts #TechForGood #shorts #ytshorts #foryou #fyp #facts #youtube #motivation #youtuber