filmov
tv
Creating correct and capable classifiers - Ian Ozsvald
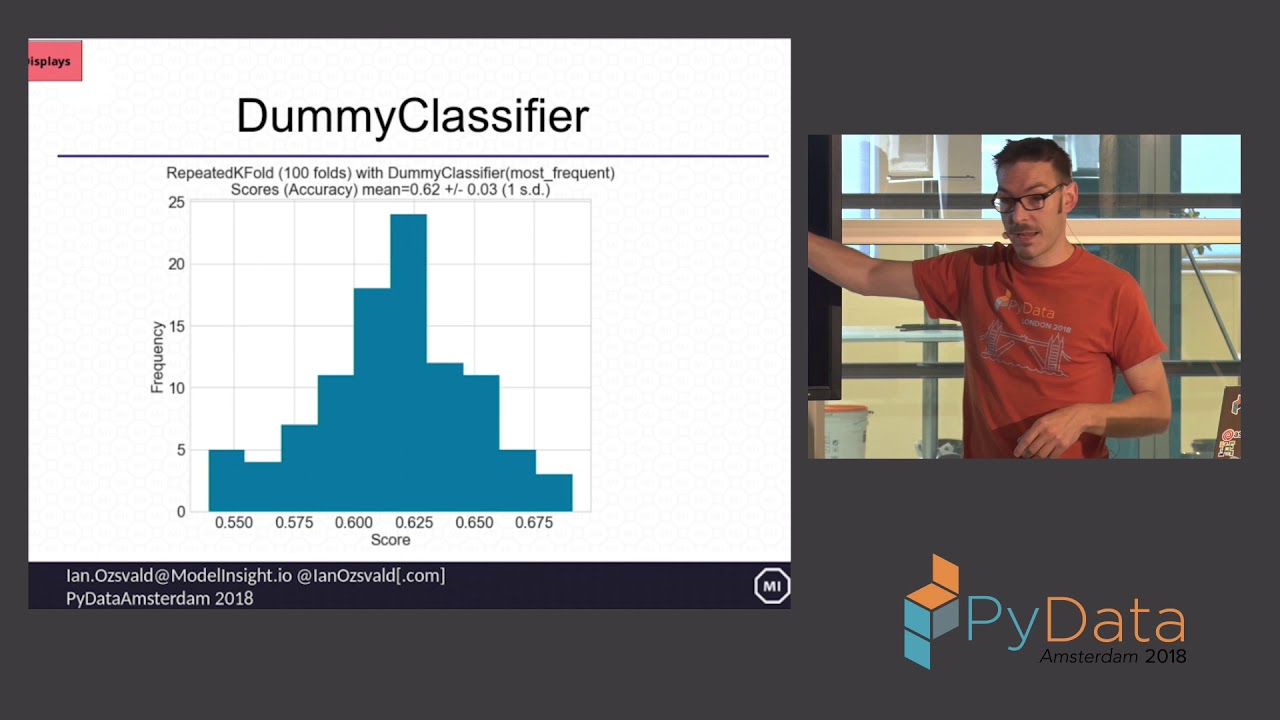
Показать описание
PyData Amsterdam 2018
Iteratively building a classifier requires a mix of skill, diagnostic ability and guesswork. I'll lay out a framework that helps you build reliable classifiers with greater confidence and less random guesswork. Tools demonstrated will include sklearn, YellowBrick, Shapley and pandas_profiling.
--
PyData is an educational program of NumFOCUS, a 501(c)3 non-profit organization in the United States. PyData provides a forum for the international community of users and developers of data analysis tools to share ideas and learn from each other. The global PyData network promotes discussion of best practices, new approaches, and emerging technologies for data management, processing, analytics, and visualization. PyData communities approach data science using many languages, including (but not limited to) Python, Julia, and R.
PyData conferences aim to be accessible and community-driven, with novice to advanced level presentations. PyData tutorials and talks bring attendees the latest project features along with cutting-edge use cases. 00:00 Welcome!
00:10 Help us add time stamps or captions to this video! See the description for details.
Iteratively building a classifier requires a mix of skill, diagnostic ability and guesswork. I'll lay out a framework that helps you build reliable classifiers with greater confidence and less random guesswork. Tools demonstrated will include sklearn, YellowBrick, Shapley and pandas_profiling.
--
PyData is an educational program of NumFOCUS, a 501(c)3 non-profit organization in the United States. PyData provides a forum for the international community of users and developers of data analysis tools to share ideas and learn from each other. The global PyData network promotes discussion of best practices, new approaches, and emerging technologies for data management, processing, analytics, and visualization. PyData communities approach data science using many languages, including (but not limited to) Python, Julia, and R.
PyData conferences aim to be accessible and community-driven, with novice to advanced level presentations. PyData tutorials and talks bring attendees the latest project features along with cutting-edge use cases. 00:00 Welcome!
00:10 Help us add time stamps or captions to this video! See the description for details.
Комментарии