filmov
tv
Anomaly Detection in Highly Imbalanced Multivariate Data Using Gaussian Mixture Model
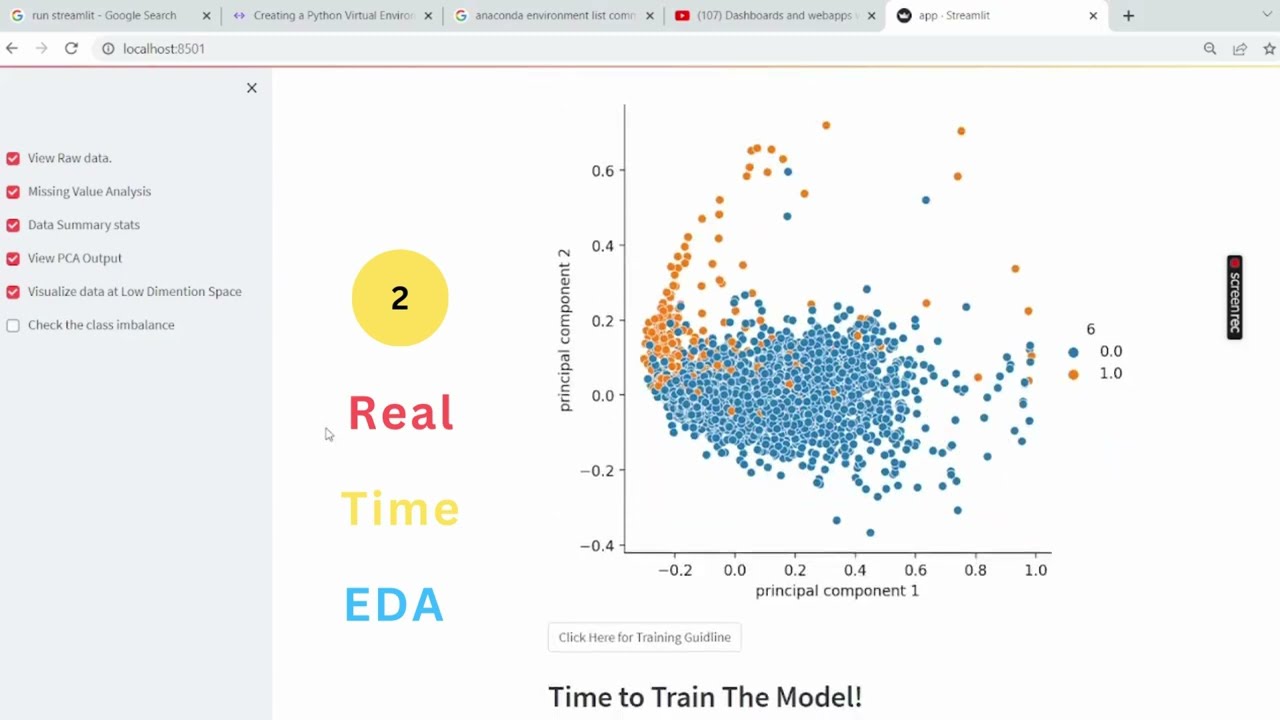
Показать описание
Project Description:
This web application is designed to detect anomalies within highly imbalanced multivariate data using the Gaussian Mixture Model, a powerful probabilistic approach. It offers an array of functionalities aimed at providing users with an interactive and intuitive experience, including:
Real-Time Data Exploration (EDA): Users can explore and analyze their data in real-time, gaining immediate insights and understanding of the dataset’s structure and key features.
Hyperparameter Tuning: The application includes tools for tuning hyperparameters, enabling users to optimize the Gaussian Mixture Model for their specific data and detection needs.
Data Visualization: Comprehensive data visualization capabilities are integrated, allowing users to visualize both the data and the model’s outputs, enhancing interpretability and decision-making.
By combining these features, the application supports users in effectively identifying and understanding anomalies in complex, imbalanced data environments.
This web application is designed to detect anomalies within highly imbalanced multivariate data using the Gaussian Mixture Model, a powerful probabilistic approach. It offers an array of functionalities aimed at providing users with an interactive and intuitive experience, including:
Real-Time Data Exploration (EDA): Users can explore and analyze their data in real-time, gaining immediate insights and understanding of the dataset’s structure and key features.
Hyperparameter Tuning: The application includes tools for tuning hyperparameters, enabling users to optimize the Gaussian Mixture Model for their specific data and detection needs.
Data Visualization: Comprehensive data visualization capabilities are integrated, allowing users to visualize both the data and the model’s outputs, enhancing interpretability and decision-making.
By combining these features, the application supports users in effectively identifying and understanding anomalies in complex, imbalanced data environments.