filmov
tv
En3D: An Enhanced Generative Model for Sculpting 3D Humans from 2D Synthetic Data
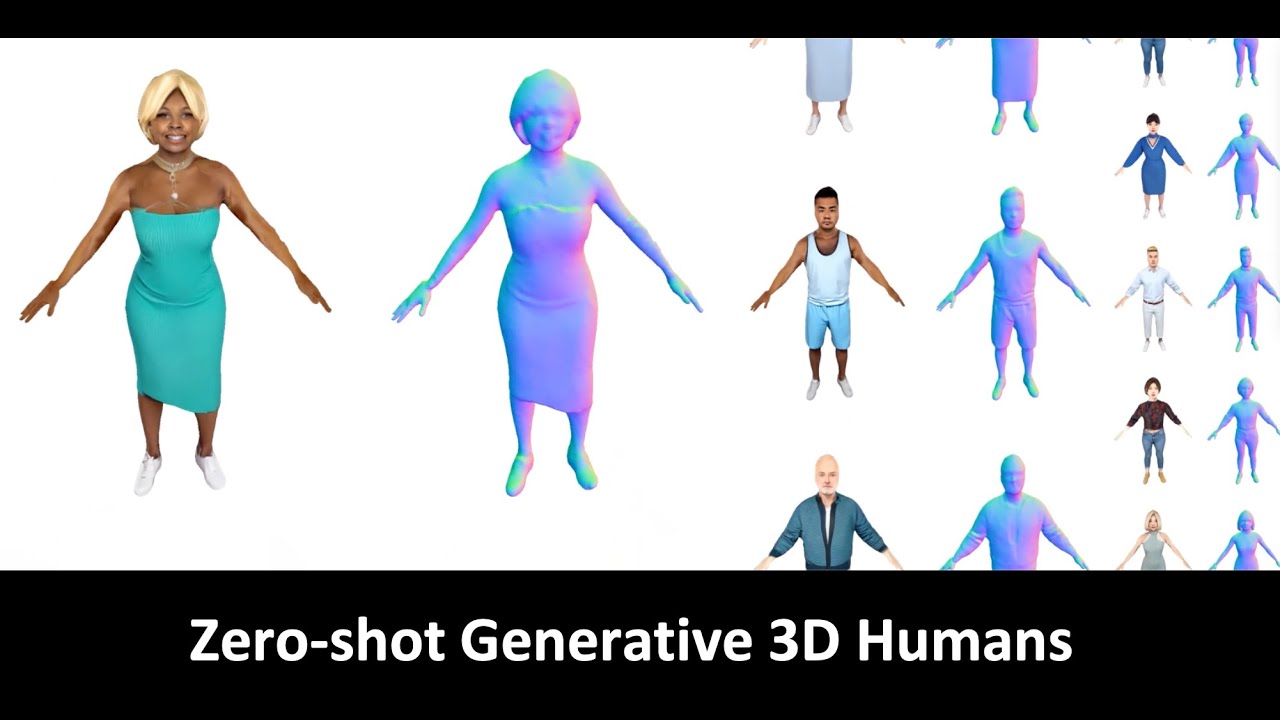
Показать описание
En3D: An Enhanced Generative Model for Sculpting 3D Humans from 2D Synthetic Data
Yifang Men, Biwen Lei, Yuan Yao, Miaomiao Cui, Zhouhui Lian, and Xuansong Xie
Abstract: We present En3D, an enhanced generative scheme for sculpting high-quality 3D human avatars. Unlike previous works that rely on scarce 3D datasets or limited 2D collections with imbalanced viewing angles and imprecise pose priors, our approach aims to develop a zero-shot 3D generative scheme capable of producing visually realistic, geometrically accurate and content-wise diverse 3D humans without relying on pre-existing 3D or 2D assets. To address this challenge, we introduce a meticulously crafted workflow that implements accurate physical modeling to learn the enhanced 3D generative model from synthetic 2D data. During inference, we integrate optimization modules to bridge the gap between realistic appearances and coarse 3D shapes. Experimental results show that our approach significantly outperforms prior works in terms of image quality, geometry accuracy and content diversity.
With the trained model, our scheme can generate a 360-degree view of a person with a consistent, high-resolution appearance from a text prompt or a single image. We also showcase the applicability of our generated avatars for animation and editing, as well as the scalability of our approach for content-style free adaptation.
Compared baseline methods:
- EG3D: Efficient Geometry-aware 3D Generative Adversarial Networks, CVPR 2022
- EVA3D: Compositional 3D Human Generation
from 2D Image Collections, ICLR 2023
- AG3D: Learning to Generate 3D Avatars from 2D Image Collections, ICCV 2023
Yifang Men, Biwen Lei, Yuan Yao, Miaomiao Cui, Zhouhui Lian, and Xuansong Xie
Abstract: We present En3D, an enhanced generative scheme for sculpting high-quality 3D human avatars. Unlike previous works that rely on scarce 3D datasets or limited 2D collections with imbalanced viewing angles and imprecise pose priors, our approach aims to develop a zero-shot 3D generative scheme capable of producing visually realistic, geometrically accurate and content-wise diverse 3D humans without relying on pre-existing 3D or 2D assets. To address this challenge, we introduce a meticulously crafted workflow that implements accurate physical modeling to learn the enhanced 3D generative model from synthetic 2D data. During inference, we integrate optimization modules to bridge the gap between realistic appearances and coarse 3D shapes. Experimental results show that our approach significantly outperforms prior works in terms of image quality, geometry accuracy and content diversity.
With the trained model, our scheme can generate a 360-degree view of a person with a consistent, high-resolution appearance from a text prompt or a single image. We also showcase the applicability of our generated avatars for animation and editing, as well as the scalability of our approach for content-style free adaptation.
Compared baseline methods:
- EG3D: Efficient Geometry-aware 3D Generative Adversarial Networks, CVPR 2022
- EVA3D: Compositional 3D Human Generation
from 2D Image Collections, ICLR 2023
- AG3D: Learning to Generate 3D Avatars from 2D Image Collections, ICCV 2023
Комментарии