filmov
tv
Accessing JSON Annotation Data in TensorFlow's tf.data.Dataset Using tf.string
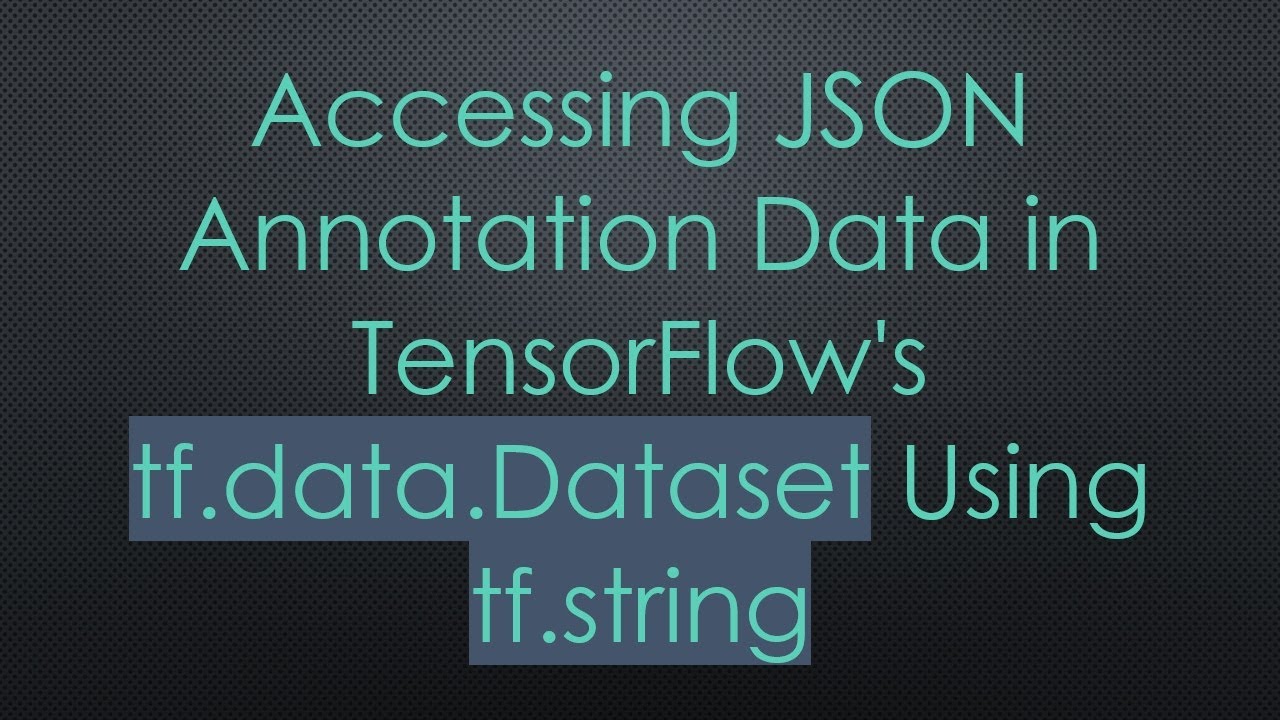
Показать описание
---
If anything seems off to you, please feel free to write me at vlogize [AT] gmail [DOT] com.
---
Problem Overview
Solution Explanation
Step 1: Reading the JSON File
[[See Video to Reveal this Text or Code Snippet]]
Step 2: Extracting Image Name from Path
In your dataset creation function, you can split the image path to get the image name. This name will serve as a key to retrieve the corresponding annotations from your JSON data.
Here's how you can do it in your specific case:
[[See Video to Reveal this Text or Code Snippet]]
Within the create_bbox function, you can decode the values and retrieve your annotations easily:
[[See Video to Reveal this Text or Code Snippet]]
Step 4: Updating the Dataset Creation Logic
Once the setup is complete, you can seamlessly integrate this logic into your existing dataset creation methods. This integration allows your TensorFlow pipeline to both preprocess images and access their annotations effectively.
Wrapping Up
Utilizing these techniques, you can enhance your data processing pipeline and ensure that your model trains on well-defined, accurate datasets.
If anything seems off to you, please feel free to write me at vlogize [AT] gmail [DOT] com.
---
Problem Overview
Solution Explanation
Step 1: Reading the JSON File
[[See Video to Reveal this Text or Code Snippet]]
Step 2: Extracting Image Name from Path
In your dataset creation function, you can split the image path to get the image name. This name will serve as a key to retrieve the corresponding annotations from your JSON data.
Here's how you can do it in your specific case:
[[See Video to Reveal this Text or Code Snippet]]
Within the create_bbox function, you can decode the values and retrieve your annotations easily:
[[See Video to Reveal this Text or Code Snippet]]
Step 4: Updating the Dataset Creation Logic
Once the setup is complete, you can seamlessly integrate this logic into your existing dataset creation methods. This integration allows your TensorFlow pipeline to both preprocess images and access their annotations effectively.
Wrapping Up
Utilizing these techniques, you can enhance your data processing pipeline and ensure that your model trains on well-defined, accurate datasets.