filmov
tv
Randall Balestriero - The Fair Language Paradox
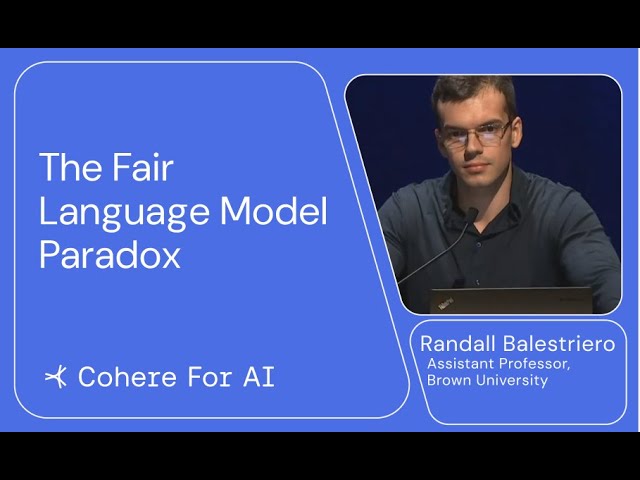
Показать описание
The Fair Language Paradox
Large Language Models (LLMs) are widely deployed in real-world applications, yet little is known about their training dynamics at the token level. Evaluation typically relies on aggregated training loss, measured at the batch or dataset level, which overlooks subtle per-token biases arising from (i) varying token-level dynamics and (ii) structural biases introduced by hyperparameters. While weight decay is commonly used to stabilize training, we reveal that it silently introduces strong biases--easily measured through token-level metrics. That bias seems to emerge for varying dataset and model sizes: as weight decay increases as low-frequency tokens are disproportionately disregarded by the model. This finding is concerning as these neglected low-frequency tokens altogether represent the vast majority of the token distribution in most languages. We conclude by proving how the current LLM pre-training strategy--classification task on highly imbalance classes--is misaligned with producing fair generative models.
Dr. Randall Balestriero is an Assistant Professor at Brown University. He has been doing research in learnable signal processing since 2013, in particular with learnable parametrized wavelets which have then been extended for deep wavelet transforms. The latter has found many applications, e.g., in the NASA's Mars rover for marsquake detection. In 2016 when joining Rice University for a PhD with Prof. Richard Baraniuk, He broadened my scope to explore Deep Networks from a theoretical persepective by employing affine spline operators. This led him to revisit and improve state-of-the-art methods, e.g., batch-normalization or generative networks. In 2021 when joining Meta AI Research (FAIR) for a postdoc with Prof. Yann LeCun, He further enlarged his research interests e.g. to include self-supervised learning or biases emerging from data-augmentation and regularization leading to many publications and conference tutorials. In 2023, he have joined GQS, Citadel, to work on highly noisy and nonstationnary financial time-series and to provide AI solutions for prediction and representation learning. Such industry exposure is driving my research agenda to provide practical solutions from first principles which he has been pursuing every day for the last 10 years.
Large Language Models (LLMs) are widely deployed in real-world applications, yet little is known about their training dynamics at the token level. Evaluation typically relies on aggregated training loss, measured at the batch or dataset level, which overlooks subtle per-token biases arising from (i) varying token-level dynamics and (ii) structural biases introduced by hyperparameters. While weight decay is commonly used to stabilize training, we reveal that it silently introduces strong biases--easily measured through token-level metrics. That bias seems to emerge for varying dataset and model sizes: as weight decay increases as low-frequency tokens are disproportionately disregarded by the model. This finding is concerning as these neglected low-frequency tokens altogether represent the vast majority of the token distribution in most languages. We conclude by proving how the current LLM pre-training strategy--classification task on highly imbalance classes--is misaligned with producing fair generative models.
Dr. Randall Balestriero is an Assistant Professor at Brown University. He has been doing research in learnable signal processing since 2013, in particular with learnable parametrized wavelets which have then been extended for deep wavelet transforms. The latter has found many applications, e.g., in the NASA's Mars rover for marsquake detection. In 2016 when joining Rice University for a PhD with Prof. Richard Baraniuk, He broadened my scope to explore Deep Networks from a theoretical persepective by employing affine spline operators. This led him to revisit and improve state-of-the-art methods, e.g., batch-normalization or generative networks. In 2021 when joining Meta AI Research (FAIR) for a postdoc with Prof. Yann LeCun, He further enlarged his research interests e.g. to include self-supervised learning or biases emerging from data-augmentation and regularization leading to many publications and conference tutorials. In 2023, he have joined GQS, Citadel, to work on highly noisy and nonstationnary financial time-series and to provide AI solutions for prediction and representation learning. Such industry exposure is driving my research agenda to provide practical solutions from first principles which he has been pursuing every day for the last 10 years.