filmov
tv
Convolutional Neural Network for Malware Classification Based on API Call Sequence
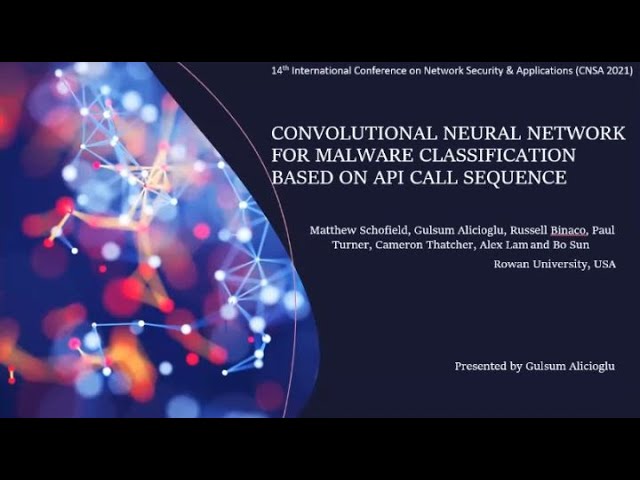
Показать описание
Title
=======
Convolutional Neural Network for Malware Classification Based on API Call Sequence
Authors
=======
Matthew Schofield, Gulsum Alicioglu, Russell Binaco, Paul Turner, Cameron Thatcher, Alex Lam and Bo Sun, Rowan University, USA
Abstract
=======
Malicious software is constantly being developed and improved, so detection and classification of malicious applications is an ever-evolving problem. Since traditional malware detection techniques fail to detect new or unknown malware, machine learning algorithms have been used to overcome this disadvantage. We present a Convolutional Neural Network (CNN) for malware type classification based on the Windows system API (Application Program Interface) calls. This research uses a database of 5385 instances of API call streams labeled with eight types of malware of the source malicious application. We use a 1-Dimensional CNN by mapping API call streams as categorical and term frequency-inverse document frequency (TF-IDF) vectors respectively. We achieved accuracy scores of 98.17% using TF-IDF vector and 95.40% via categorical vector. The proposed 1-D CNN outperformed other traditional classification techniques with overall accuracy score of 91.0%.
Keywords
=====
Convolutional Neural Network, Malware Classification, Windows API Calls, Term Frequency Inverse Document Frequency Vectors.
------------------------------------------------------------------------------
Full Text
---------------
Abstract URL
----------------------
Volume URL
--------------------
Time Stamps
00:00 Outline
00:30 Intro
03:57 Related work
05:22 Windows Malware API Call Dataset
06:16 Methodology
09:49 Results
11:58 Visualization
13:56 Conclusion
#machinelearning #Convolutionalneuralnetwork #Malware #Classification #WindowsAPIcalls #Term Frequency #security #Deepreinforcementlearning
=======
Convolutional Neural Network for Malware Classification Based on API Call Sequence
Authors
=======
Matthew Schofield, Gulsum Alicioglu, Russell Binaco, Paul Turner, Cameron Thatcher, Alex Lam and Bo Sun, Rowan University, USA
Abstract
=======
Malicious software is constantly being developed and improved, so detection and classification of malicious applications is an ever-evolving problem. Since traditional malware detection techniques fail to detect new or unknown malware, machine learning algorithms have been used to overcome this disadvantage. We present a Convolutional Neural Network (CNN) for malware type classification based on the Windows system API (Application Program Interface) calls. This research uses a database of 5385 instances of API call streams labeled with eight types of malware of the source malicious application. We use a 1-Dimensional CNN by mapping API call streams as categorical and term frequency-inverse document frequency (TF-IDF) vectors respectively. We achieved accuracy scores of 98.17% using TF-IDF vector and 95.40% via categorical vector. The proposed 1-D CNN outperformed other traditional classification techniques with overall accuracy score of 91.0%.
Keywords
=====
Convolutional Neural Network, Malware Classification, Windows API Calls, Term Frequency Inverse Document Frequency Vectors.
------------------------------------------------------------------------------
Full Text
---------------
Abstract URL
----------------------
Volume URL
--------------------
Time Stamps
00:00 Outline
00:30 Intro
03:57 Related work
05:22 Windows Malware API Call Dataset
06:16 Methodology
09:49 Results
11:58 Visualization
13:56 Conclusion
#machinelearning #Convolutionalneuralnetwork #Malware #Classification #WindowsAPIcalls #Term Frequency #security #Deepreinforcementlearning