filmov
tv
Learning for Active 3D Mapping
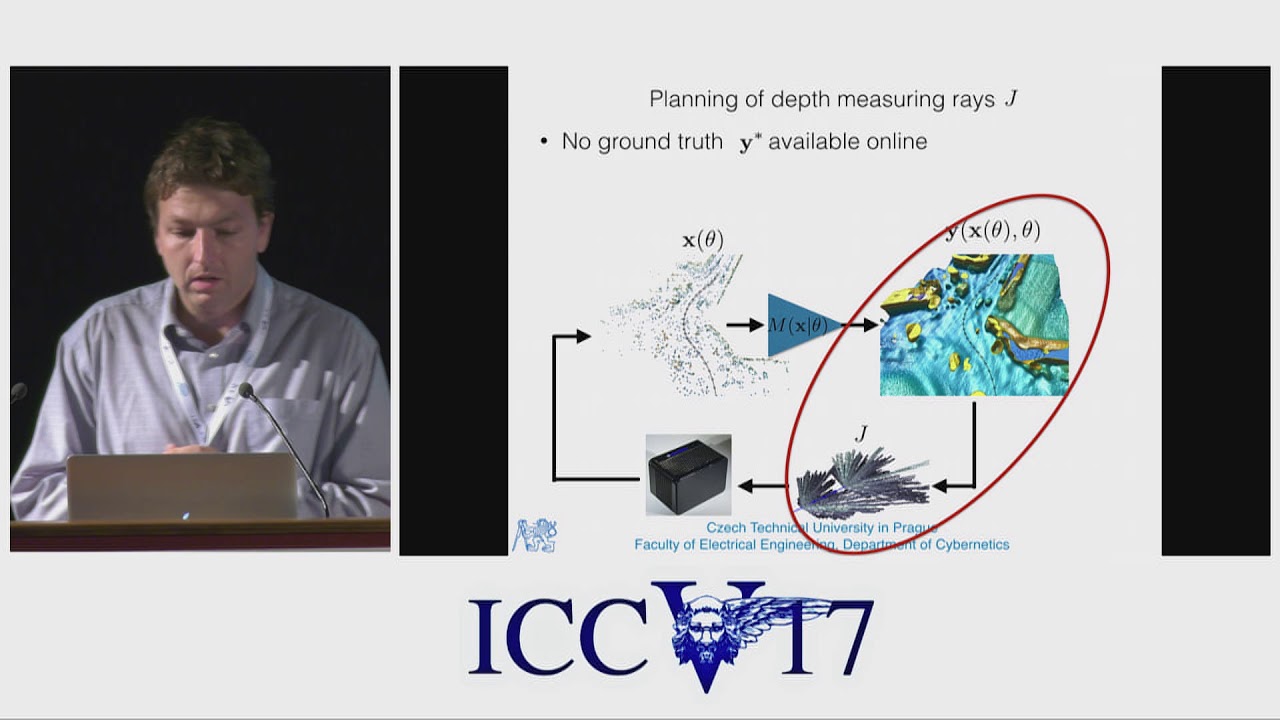
Показать описание
ICCV17 | 1812 | Learning for Active 3D Mapping
Karel Zimmermann (Czech Technical University in Prague), Tomas Petricek (Czech Technical University in Prague), Vojtech Salansky (Czech Technical University in Prague), Tomas Svoboda (Czech Technical University in Prague)
We propose an active 3D mapping method for depth sensors, which allow individual control of depth-measuring rays, such as the newly emerging Solid State Lidars. The method simultaneously (i) learns to reconstruct a dense 3D voxel-map from sparse depth measurements, and (ii) optimizes the reactive control of depth-measuring rays. To make the first step towards the online control optimization, we propose a fast greedy algorithm, which needs to update its cost function in only a small fraction of possible rays. The approximation ratio of the greedy algorithm is derived. Experimental evaluation on the subset of the Kitti dataset demonstrates significant improvement in the 3D map accuracy when learning-to-reconstruct from sparse measurements is coupled with the optimization where-to-measure.
Karel Zimmermann (Czech Technical University in Prague), Tomas Petricek (Czech Technical University in Prague), Vojtech Salansky (Czech Technical University in Prague), Tomas Svoboda (Czech Technical University in Prague)
We propose an active 3D mapping method for depth sensors, which allow individual control of depth-measuring rays, such as the newly emerging Solid State Lidars. The method simultaneously (i) learns to reconstruct a dense 3D voxel-map from sparse depth measurements, and (ii) optimizes the reactive control of depth-measuring rays. To make the first step towards the online control optimization, we propose a fast greedy algorithm, which needs to update its cost function in only a small fraction of possible rays. The approximation ratio of the greedy algorithm is derived. Experimental evaluation on the subset of the Kitti dataset demonstrates significant improvement in the 3D map accuracy when learning-to-reconstruct from sparse measurements is coupled with the optimization where-to-measure.