filmov
tv
Safe and Efficient Reinforcement Learning for Surgical Robots
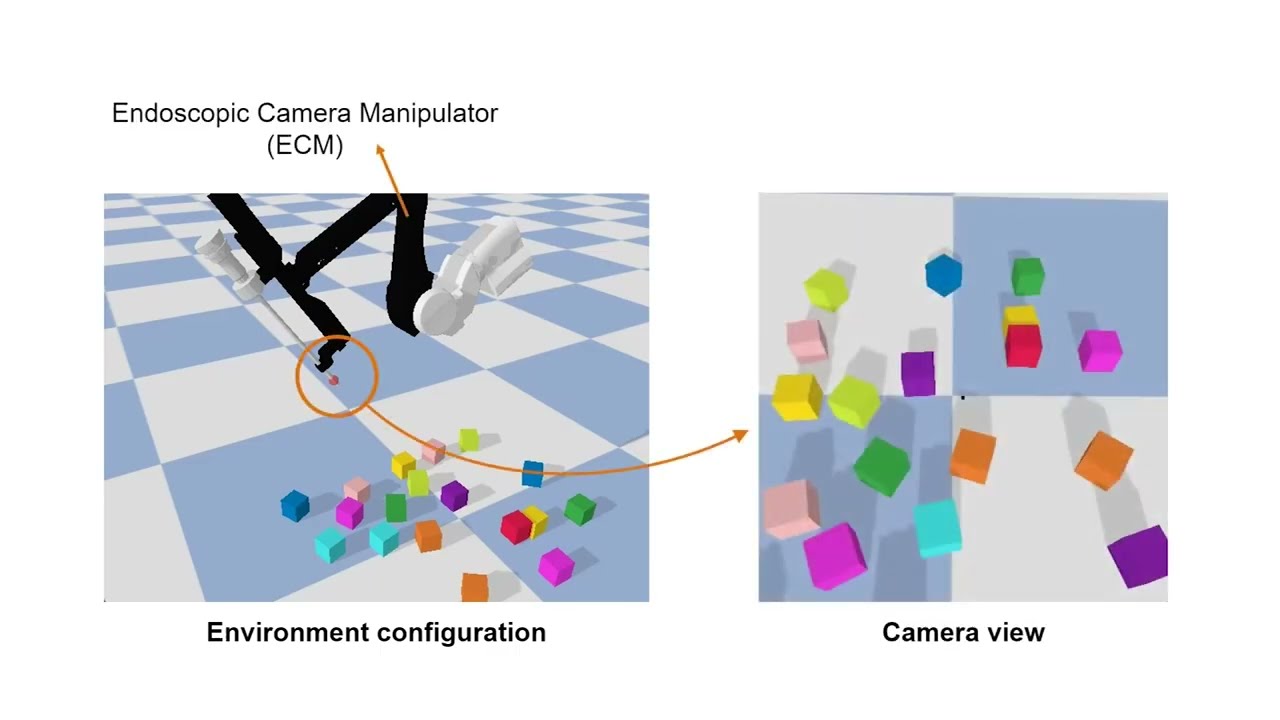
Показать описание
Indirect simultaneous positioning (ISP), where internal
tissue points are placed at desired locations indirectly
through the manipulation of boundary points, is a type of
subtask frequently performed in robotic surgeries. Although
challenging due to complex tissue dynamics, automating the
task can potentially reduce the workload of surgeons. This
paper presents a sim-to-real framework for learning to automate
the task without interacting with a real environment, and for
planning preoperatively to find the grasping points that minimize
local tissue deformation. A control policy is learned using deep
reinforcement learning (DRL) in the FEM-based simulation
environment and transferred to real-world situation. Grasping
points are planned in the simulator by utilizing the trained
policy using Bayesian optimization (BO). Inconsistent simulation
performance is overcome by formulating the problem as a state
augmented Markov decision process (MDP). Experimental results
show that the learned policy places the internal tissue points
accurately, and that the planned grasping points yield small
tissue deformation among the trials. The proposed learning
and planning scheme is able to automate internal tissue point
manipulation in surgeries and has the potential to be generalized
to complex surgical scenarios.
tissue points are placed at desired locations indirectly
through the manipulation of boundary points, is a type of
subtask frequently performed in robotic surgeries. Although
challenging due to complex tissue dynamics, automating the
task can potentially reduce the workload of surgeons. This
paper presents a sim-to-real framework for learning to automate
the task without interacting with a real environment, and for
planning preoperatively to find the grasping points that minimize
local tissue deformation. A control policy is learned using deep
reinforcement learning (DRL) in the FEM-based simulation
environment and transferred to real-world situation. Grasping
points are planned in the simulator by utilizing the trained
policy using Bayesian optimization (BO). Inconsistent simulation
performance is overcome by formulating the problem as a state
augmented Markov decision process (MDP). Experimental results
show that the learned policy places the internal tissue points
accurately, and that the planned grasping points yield small
tissue deformation among the trials. The proposed learning
and planning scheme is able to automate internal tissue point
manipulation in surgeries and has the potential to be generalized
to complex surgical scenarios.