filmov
tv
A Deep Learning Approach to Traffic Accident Prediction on Heterogeneous Spatio-Temporal Data
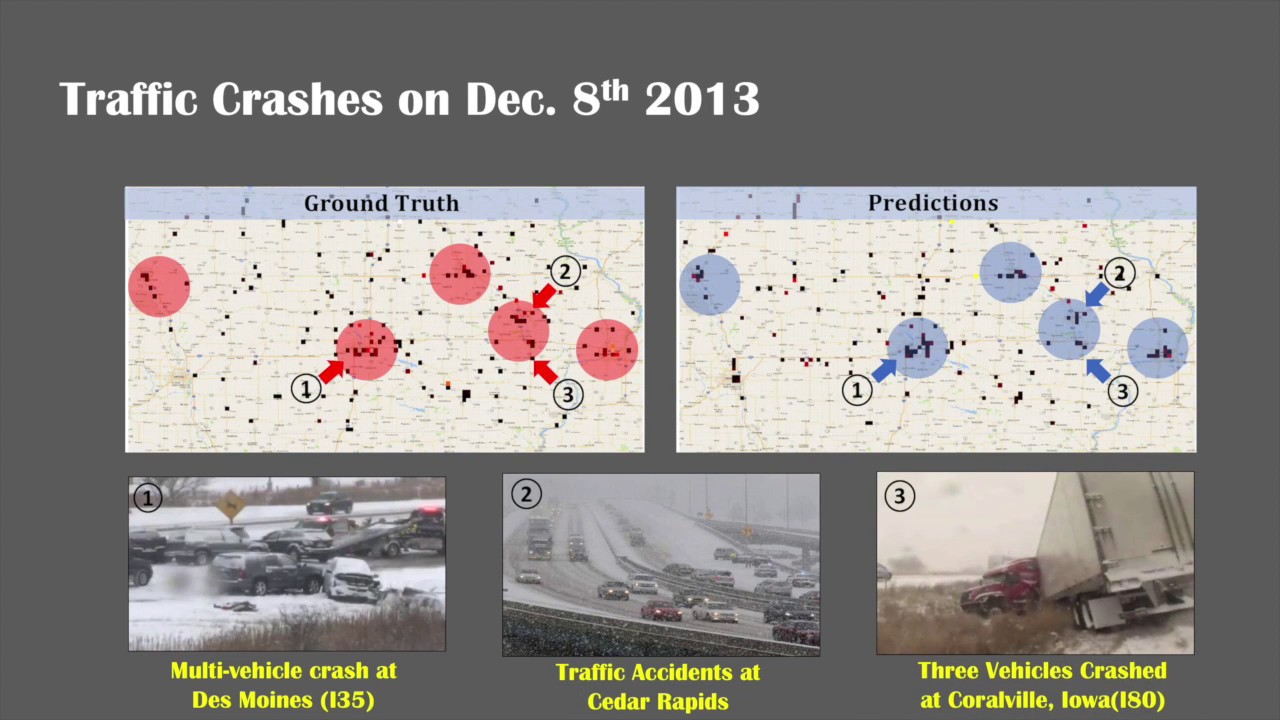
Показать описание
Hetero-ConvLSTM: A Deep Learning Approach to Traffic Accident Prediction on Heterogeneous Spatio-Temporal Data
Authors:
Zhuoning Yuan (University of Iowa); Xun Zhou (University of Iowa); Tianbao Yang (University of Iowa)
Abstract:
Predicting traffic accidents is a crucial problem to improving transportation and public safety as well as safe routing. The problem is also challenging due to the rareness of accidents in space and time and spatial heterogeneity of the environment (e.g., urban vs. rural). Most previous research on traffic accident prediction conducted by domain researchers simply applied classical prediction models on limited data without addressing the above challenges properly, thus leading to unsatisfactory performance. A small number of recent works have attempted to use deep learning for traffic accident prediction. However, they either ignore time information or use only data from a small and homogeneous study area (a city), without handling spatial heterogeneity and temporal auto-correlation properly at the same time.
In this paper we perform a comprehensive study on the traffic accident prediction problem using the Convolutional Long Short-Term Memory (ConvLSTM) neural network model. A number of detailed features such as weather, environment, road condition, and traffic volume are extracted from big datasets over the state of Iowa across 8 years. To address the spatial heterogeneity challenge in the data, we propose a Hetero-ConvLSTM framework, where a few novel ideas are implemented on top of the basic ConvLSTM model, such as incorporating spatial graph features and spatial model ensemble. Extensive experiments on the 8-year data over the entire state of Iowa show that the proposed framework makes reasonably accurate predictions and significantly improves the prediction accuracy over baseline approaches.
Authors:
Zhuoning Yuan (University of Iowa); Xun Zhou (University of Iowa); Tianbao Yang (University of Iowa)
Abstract:
Predicting traffic accidents is a crucial problem to improving transportation and public safety as well as safe routing. The problem is also challenging due to the rareness of accidents in space and time and spatial heterogeneity of the environment (e.g., urban vs. rural). Most previous research on traffic accident prediction conducted by domain researchers simply applied classical prediction models on limited data without addressing the above challenges properly, thus leading to unsatisfactory performance. A small number of recent works have attempted to use deep learning for traffic accident prediction. However, they either ignore time information or use only data from a small and homogeneous study area (a city), without handling spatial heterogeneity and temporal auto-correlation properly at the same time.
In this paper we perform a comprehensive study on the traffic accident prediction problem using the Convolutional Long Short-Term Memory (ConvLSTM) neural network model. A number of detailed features such as weather, environment, road condition, and traffic volume are extracted from big datasets over the state of Iowa across 8 years. To address the spatial heterogeneity challenge in the data, we propose a Hetero-ConvLSTM framework, where a few novel ideas are implemented on top of the basic ConvLSTM model, such as incorporating spatial graph features and spatial model ensemble. Extensive experiments on the 8-year data over the entire state of Iowa show that the proposed framework makes reasonably accurate predictions and significantly improves the prediction accuracy over baseline approaches.
Комментарии