filmov
tv
Hybrid Representations for 3D Understanding -- Qixing Huang
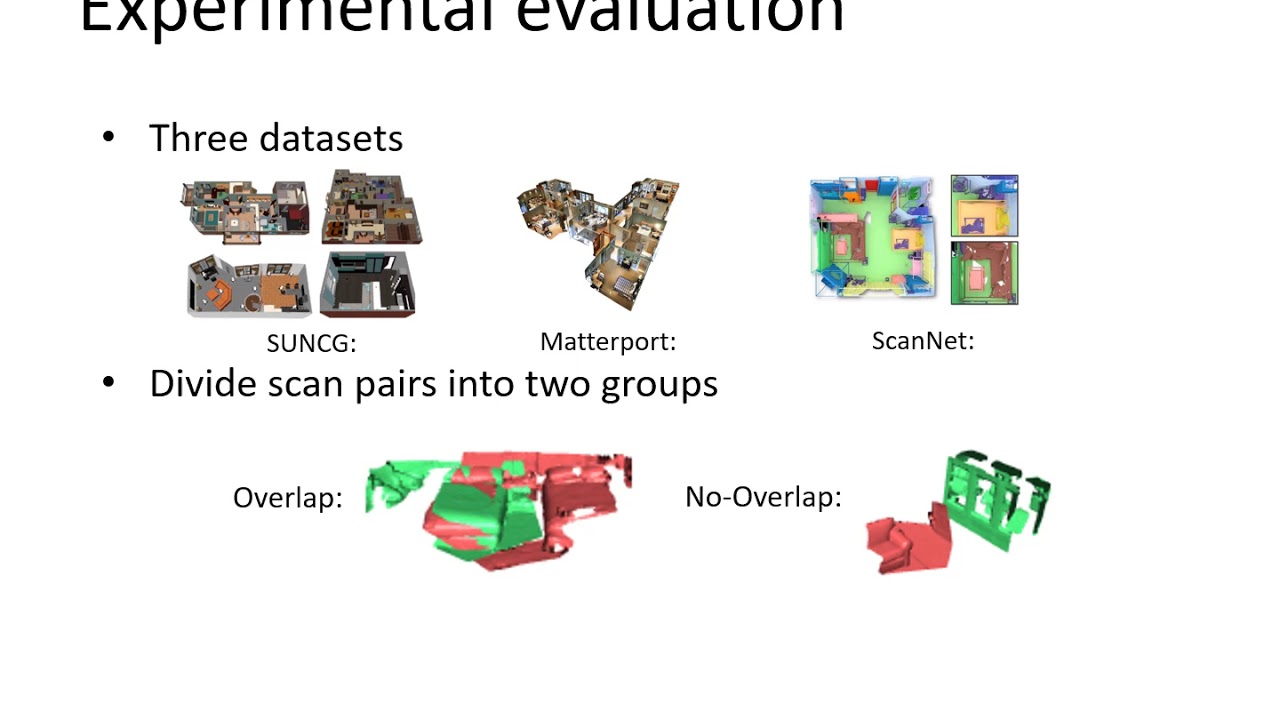
Показать описание
CVPR 2020 Workshop on Deep Learning Foundations of Geometric Shape Modeling and Reconstruction
Speaker: Qixing Huang is an assistant professor of Computer Science at the University of Texas at Austin. He obtained his PhD in Computer Science from Stanford University. He was a research assistant professor at Toyota Technological Institute at Chicago before joining UT Austin. Dr. Huang's research spans the fields of computer vision, computer graphics, and machine learning, and publishes extensively in venues such as SIGGRAPH, CVPR, ICCV, ECCV, NeuriPS, ICML, and etc. In particular, his recent focus is on developing machine learning algorithms (particularly deep learning) that leverage Big Data to solve core problems in computer vision, computer graphics and computational biology. He is also interested in statistical data analysis, compressive sensing, low-rank matrix recovery, and large-scale optimization, which provides theoretical foundation for his research.
Talk abstract: Choosing suitable data representations is one of the most critical topics when installing machine learning on 3D data. Instead of exploring a single representation, there is great potential in integrating multiple representations. Such hybrid representations exhibit advantages in uncertainty reduction, adaptative feature selection, and self-supervision, among others. This talk introduces several recent results in pose estimation, object detection, and 3D reconstruction.
Speaker: Qixing Huang is an assistant professor of Computer Science at the University of Texas at Austin. He obtained his PhD in Computer Science from Stanford University. He was a research assistant professor at Toyota Technological Institute at Chicago before joining UT Austin. Dr. Huang's research spans the fields of computer vision, computer graphics, and machine learning, and publishes extensively in venues such as SIGGRAPH, CVPR, ICCV, ECCV, NeuriPS, ICML, and etc. In particular, his recent focus is on developing machine learning algorithms (particularly deep learning) that leverage Big Data to solve core problems in computer vision, computer graphics and computational biology. He is also interested in statistical data analysis, compressive sensing, low-rank matrix recovery, and large-scale optimization, which provides theoretical foundation for his research.
Talk abstract: Choosing suitable data representations is one of the most critical topics when installing machine learning on 3D data. Instead of exploring a single representation, there is great potential in integrating multiple representations. Such hybrid representations exhibit advantages in uncertainty reduction, adaptative feature selection, and self-supervision, among others. This talk introduces several recent results in pose estimation, object detection, and 3D reconstruction.