filmov
tv
Mini-Course: Reconstruction methods for sparse-data X-ray tomography - Class 01
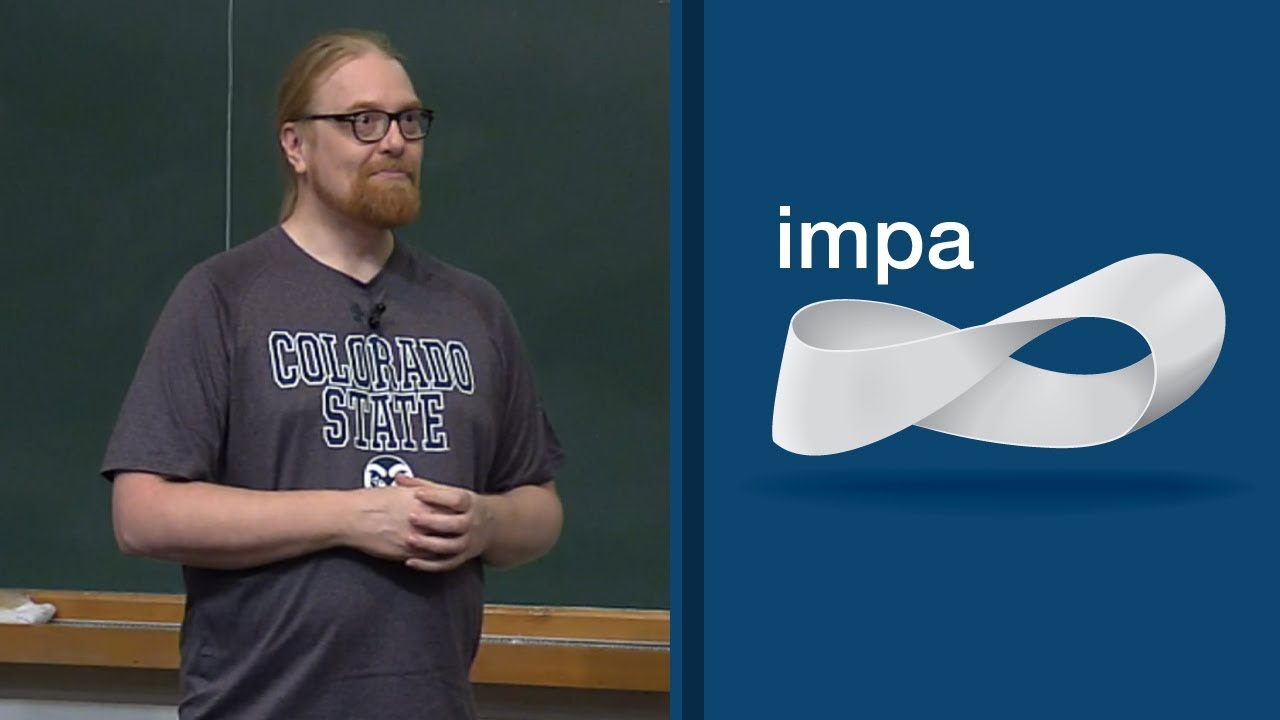
Показать описание
Mini-Course: Reconstruction methods for sparse-data X-ray tomography - Class 01
Mini-Course: Samuli Siltanen (University of Helsinki, Finland)
Title: Reconstruction methods for sparse-data X-ray tomography
Abstract:
X-ray tomography is an imaging method where an unknown physical body is photographed from many directions using X-rays. The X-rays passing through the object lose their intensity exponentially in proportion to the density of the material along the ray according to the Beer-Lambert law. After a calibration step one arrives at the following mathematical problem: can one recover a non-negative,
compactly supported function from the knowledge of integrals of that function along lines? Johann Radon showed in his seminal 1917 article how to do that in dimension two when all possible line integrals are known. Radon’s geometric reconstruction formula serves as the foundation of today’s Computerized Tomography (CT) scanners in hospitals in the form of the Filtered Back-Projection (FBP) algorithm. FBP is based on inverting the so-called Radon transform. Recently, there is growing interest in X-ray tomography imaging based on limited data. The main reason for this is the need to limit the harmful radiation dose to the patient. Mathematically, the problem of recovering a function from an incomplete set of line integrals is an example of a linear ill-posed inverse problem. Illposedness means that the reconstruction problem is extremely
sensitive to measurement noise and modelling errors. In such situations the FBP algorithm is not optimal. This course discusses variational regularisation methods for limited-data X-ray tomography, including classical Tikhonov regularisation and modern sparsitypromoting algorithms such as Total Variation regularization. The core idea behind these methods is complementing the insufficient measurement data by additional information about the unknown
function. The methods presented in the course are applicable to any linear illposed inverse problems. Also, they can be extended to nonlinear
cases.
IMPA - Instituto de Matemática Pura e Aplicada ©
Os direitos sobre todo o material deste canal pertencem ao Instituto de Matemática Pura e Aplicada, sendo vedada a utilização total ou parcial do conteúdo sem autorização prévia e por escrito do referido titular, salvo nas hipóteses previstas na legislação vigente.
The rights over all the material in this channel belong to the Instituto de Matemática Pura e Aplicada, and it is forbidden to use all or part of it without prior written authorization from the above mentioned holder, except in the cases prescribed in the current legislation.
Mini-Course: Samuli Siltanen (University of Helsinki, Finland)
Title: Reconstruction methods for sparse-data X-ray tomography
Abstract:
X-ray tomography is an imaging method where an unknown physical body is photographed from many directions using X-rays. The X-rays passing through the object lose their intensity exponentially in proportion to the density of the material along the ray according to the Beer-Lambert law. After a calibration step one arrives at the following mathematical problem: can one recover a non-negative,
compactly supported function from the knowledge of integrals of that function along lines? Johann Radon showed in his seminal 1917 article how to do that in dimension two when all possible line integrals are known. Radon’s geometric reconstruction formula serves as the foundation of today’s Computerized Tomography (CT) scanners in hospitals in the form of the Filtered Back-Projection (FBP) algorithm. FBP is based on inverting the so-called Radon transform. Recently, there is growing interest in X-ray tomography imaging based on limited data. The main reason for this is the need to limit the harmful radiation dose to the patient. Mathematically, the problem of recovering a function from an incomplete set of line integrals is an example of a linear ill-posed inverse problem. Illposedness means that the reconstruction problem is extremely
sensitive to measurement noise and modelling errors. In such situations the FBP algorithm is not optimal. This course discusses variational regularisation methods for limited-data X-ray tomography, including classical Tikhonov regularisation and modern sparsitypromoting algorithms such as Total Variation regularization. The core idea behind these methods is complementing the insufficient measurement data by additional information about the unknown
function. The methods presented in the course are applicable to any linear illposed inverse problems. Also, they can be extended to nonlinear
cases.
IMPA - Instituto de Matemática Pura e Aplicada ©
Os direitos sobre todo o material deste canal pertencem ao Instituto de Matemática Pura e Aplicada, sendo vedada a utilização total ou parcial do conteúdo sem autorização prévia e por escrito do referido titular, salvo nas hipóteses previstas na legislação vigente.
The rights over all the material in this channel belong to the Instituto de Matemática Pura e Aplicada, and it is forbidden to use all or part of it without prior written authorization from the above mentioned holder, except in the cases prescribed in the current legislation.