filmov
tv
Interpreting P and q values in the results of genomic data analysis
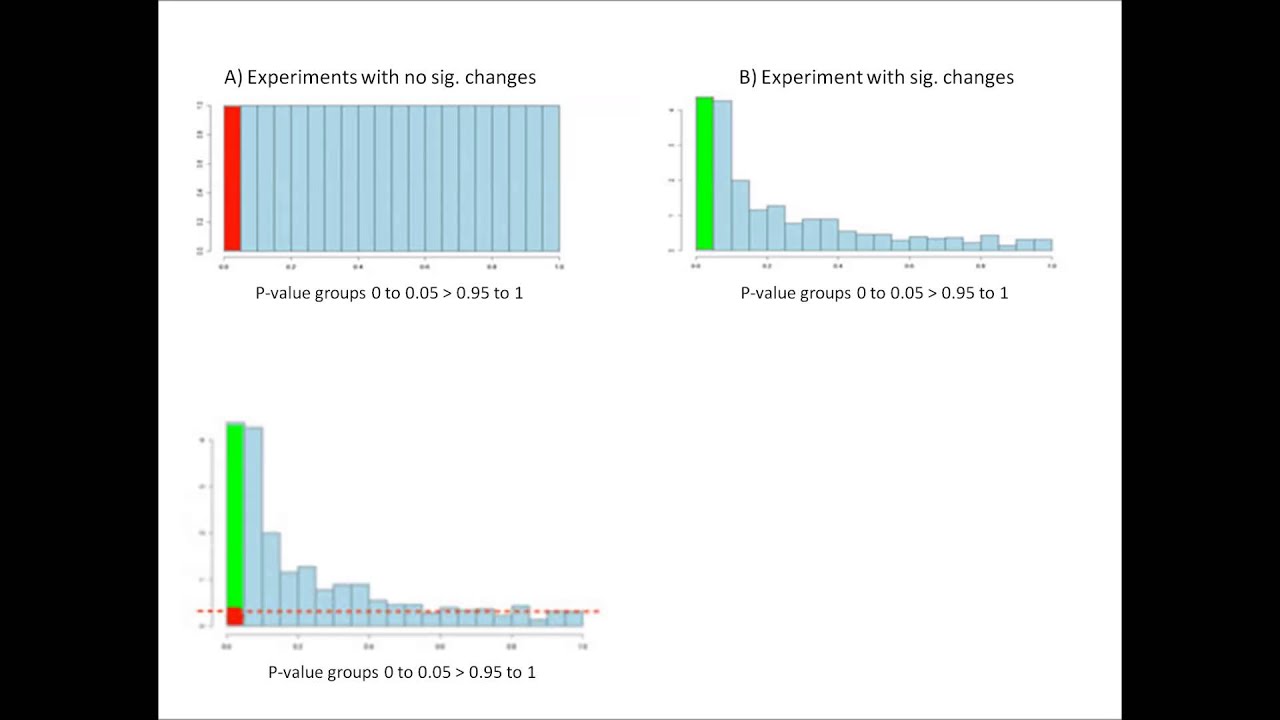
Показать описание
If this was helpful this please give a "thumb up". Otherwise, leave a comment so I can improve the content - thanks!
This video has been made a primer to the use of P and q values that are used to indicate the significance of results in genomic data analysis and elsewhere.
It is based upon an article presented by the company Nonlinear on their web site:
[EDIT May 2015]
The above URL now redirects to:
If you have any comments please let me know.
This video has been made a primer to the use of P and q values that are used to indicate the significance of results in genomic data analysis and elsewhere.
It is based upon an article presented by the company Nonlinear on their web site:
[EDIT May 2015]
The above URL now redirects to:
If you have any comments please let me know.
Interpreting P and q values in the results of genomic data analysis
FDR, q-values vs p-values: multiple testing simply explained!
p-Value (Statistics made simple)
FDR - Storey's method and q values
p-values: What they are and how to interpret them
Statistical Significance, the Null Hypothesis and P-Values Defined & Explained in One Minute
Conditional Statements: if p then q
What Is A P-Value? - Clearly Explained
8.17: How to pick the value of p in ARIMA models using ACF & PACF?
P-values and significance tests | AP Statistics | Khan Academy
02417 Lecture 6 part B: Identifying order of ARIMA models
How to Find Optimal ARIMA Model Parameters (p,d,q) | ACF, PACF, and AIC Explained
ARIMA Model | How to Choose p,d,q Value in ARIMA Model Along with Output Interpretation #arima
8.22: Choosing P & Q in SARIMA models using ACF and PACF
8.16: Choosing AR(p) and MA(q) in ARIMA models using ACF and PACF
8.18: How to pick the value of q in ARIMA models using ACF & PACF?
P,Q,R,S,T waves in the EKG
Vertex form- p and q value
P-Value Method For Hypothesis Testing
Statistics made easy ! ! ! Learn about the t-test, the chi square test, the p value and more
Interpreting ACF PACF Plots in Time Series Forecasting - order of AR and MA Model - TeKnowledGeek
Q value
Intro to Truth Tables | Negation, Conjunction, and Disjunction
Time Series | What is ACF, PACF, P, D, Q and How to Find it | ARIMA, AR, I, MA | #4
Комментарии