filmov
tv
MLOps Roadmap: A Step-by-Step Guide for 2024
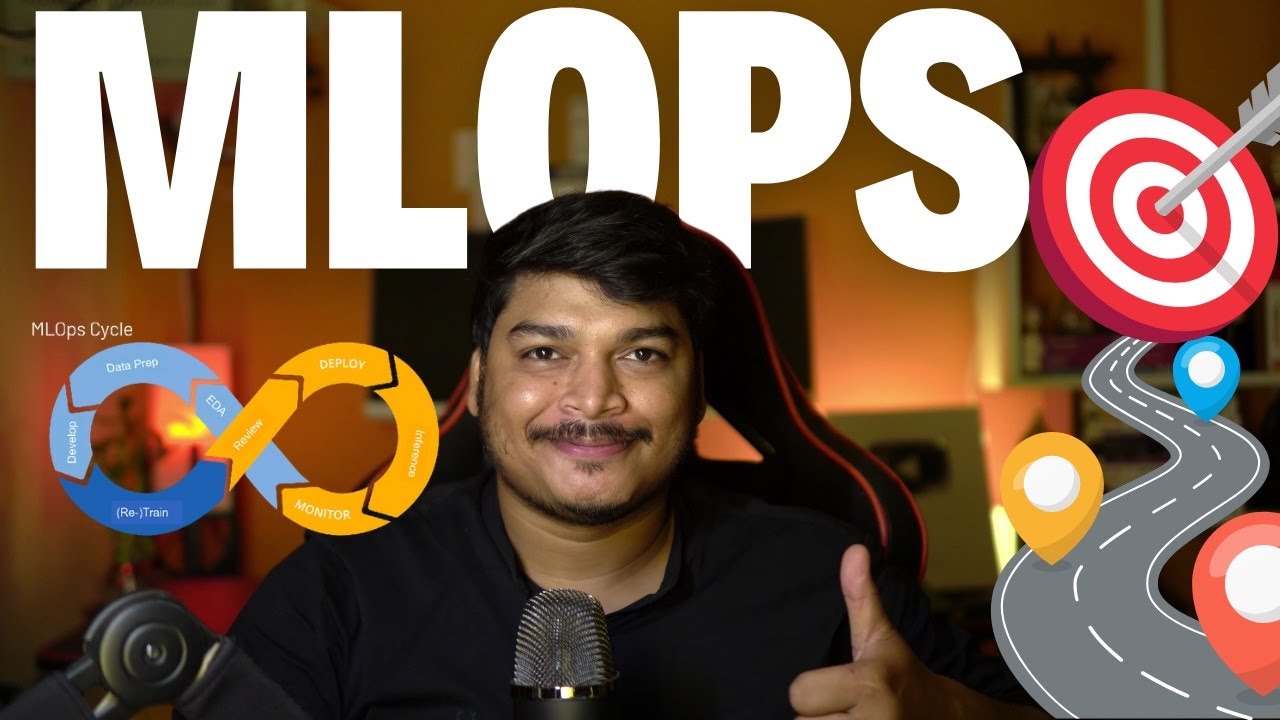
Показать описание
Welcome to my latest video where I take you through the essential roadmap for mastering MLOps in 2024! Whether you're a beginner or an experienced professional, this comprehensive guide will help you navigate the world of Machine Learning Operations, combining ML and DevOps to streamline your workflow and enhance your projects.
🚀 In This Video, You Will Learn:
ML Foundations: Understand supervised, unsupervised, and reinforcement learning.
ML Algorithms: Dive into regression, classification, clustering, and dimensionality reduction.
ML Workflows: Learn about data collection, model selection, training, evaluation, and deployment.
Key Tools and Platforms: Git, Jenkins, Docker, Kubernetes, TensorFlow, PyTorch, and more.
Model Development: Best practices for training, hyperparameter tuning, and serving models.
Data Engineering: Explore ETL pipelines, data storage solutions, and processing frameworks.
Scaling and Automation: Utilize cloud services and distributed training for efficient workflows.
Security and Compliance: Ensure data privacy and model governance.
💬 Leave a Comment:
Got questions or feedback? Drop them in the comments section below. I’d love to hear your thoughts and help you on your MLOps journey!
#mlops #devops #roadmap
MLOps, Machine Learning Operations, ML, DevOps, Data Science, TensorFlow, PyTorch, Docker, Kubernetes, AI, Cloud Computing, MLOps Roadmap, 2024 Guide, ML Workflow, Model Deployment, Data Engineering, Hyperparameter Tuning, Kubeflow
🚀 In This Video, You Will Learn:
ML Foundations: Understand supervised, unsupervised, and reinforcement learning.
ML Algorithms: Dive into regression, classification, clustering, and dimensionality reduction.
ML Workflows: Learn about data collection, model selection, training, evaluation, and deployment.
Key Tools and Platforms: Git, Jenkins, Docker, Kubernetes, TensorFlow, PyTorch, and more.
Model Development: Best practices for training, hyperparameter tuning, and serving models.
Data Engineering: Explore ETL pipelines, data storage solutions, and processing frameworks.
Scaling and Automation: Utilize cloud services and distributed training for efficient workflows.
Security and Compliance: Ensure data privacy and model governance.
💬 Leave a Comment:
Got questions or feedback? Drop them in the comments section below. I’d love to hear your thoughts and help you on your MLOps journey!
#mlops #devops #roadmap
MLOps, Machine Learning Operations, ML, DevOps, Data Science, TensorFlow, PyTorch, Docker, Kubernetes, AI, Cloud Computing, MLOps Roadmap, 2024 Guide, ML Workflow, Model Deployment, Data Engineering, Hyperparameter Tuning, Kubeflow
Комментарии