filmov
tv
#94 - ALAN CHAN - AI Alignment and Governance #NEURIPS
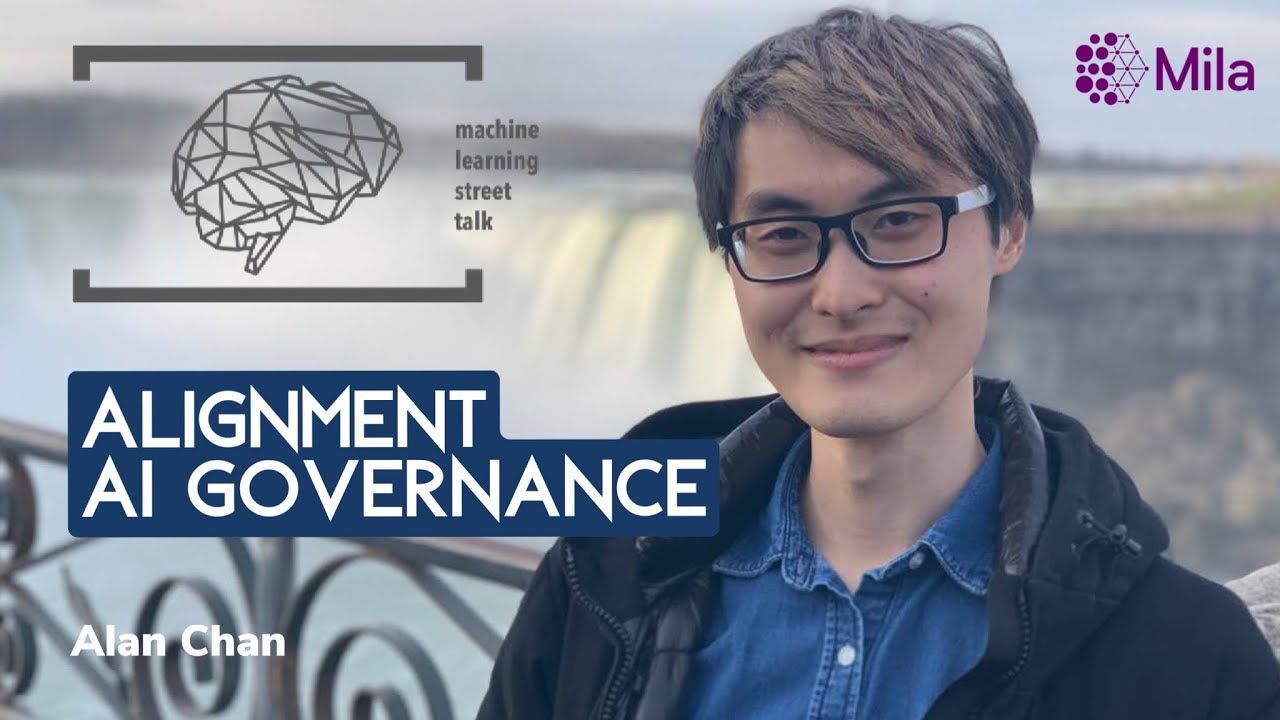
Показать описание
Alan Chan is a PhD student at Mila, the Montreal Institute for Learning Algorithms, supervised by Nicolas Le Roux. Before joining Mila, Alan was a Masters student at the Alberta Machine Intelligence Institute and the University of Alberta, where he worked with Martha White. Alan's expertise and research interests encompass value alignment and AI governance. He is currently exploring the measurement of harms from language models and the incentives that agents have to impact the world. Alan's research focuses on understanding and controlling the values expressed by machine learning models. His projects have examined the regulation of explainability in algorithmic systems, scoring rules for performative binary prediction, the effects of global exclusion in AI development, and the role of a graduate student in approaching ethical impacts in AI research. In addition, Alan has conducted research into inverse policy evaluation for value-based sequential decision-making, and the concept of "normal accidents" and AI systems. Alan's research is motivated by the need to align AI systems with human values, and his passion for scientific and governance work in this field. Alan's energy and enthusiasm for his field is infectious.
In this conversation, Alan and Tim discussed their respective views on the concept of alignment, particularly in regard to artificial intelligence (AI). Tim began by expressing his intuitive skepticism of alignment, citing the difficulty of scaling large systems, such as Google, and the limitations of the AI-XI conception of intelligence. Alan then argued that AI might be able to escape these bottlenecks, and that it is an open question as to how far we need to go in order to build pure utility maximizers in order to achieve some level of danger.
Tim then raised the issue of the reward function being too complicated to capture the dynamics of a macroscopic complex system. Alan agreed, and went on to say that he was pessimistic about alignment due to the potential for misgeneralization and power-seeking. He argued that the best way forward was to take a slower, more thoughtful approach to AI development and to respect norms, values, and rights.
Overall, this conversation highlighted the complexity of the concept of alignment and the need for careful consideration and further research when it comes to AI development.
References:
The Rationalist's Guide to the Galaxy: Superintelligent AI and the Geeks Who Are Trying to Save Humanity's Future [Tim Chivers]
The implausibility of intelligence explosion [Chollet]
Superintelligence: Paths, Dangers, Strategies [Bostrom]
A Theory of Universal Artificial Intelligence based on Algorithmic Complexity [Hutter]
Комментарии