filmov
tv
Bigger Chips = Better AI? Nvidia's Blackwell vs. Cerebras Wafer Scale Engine
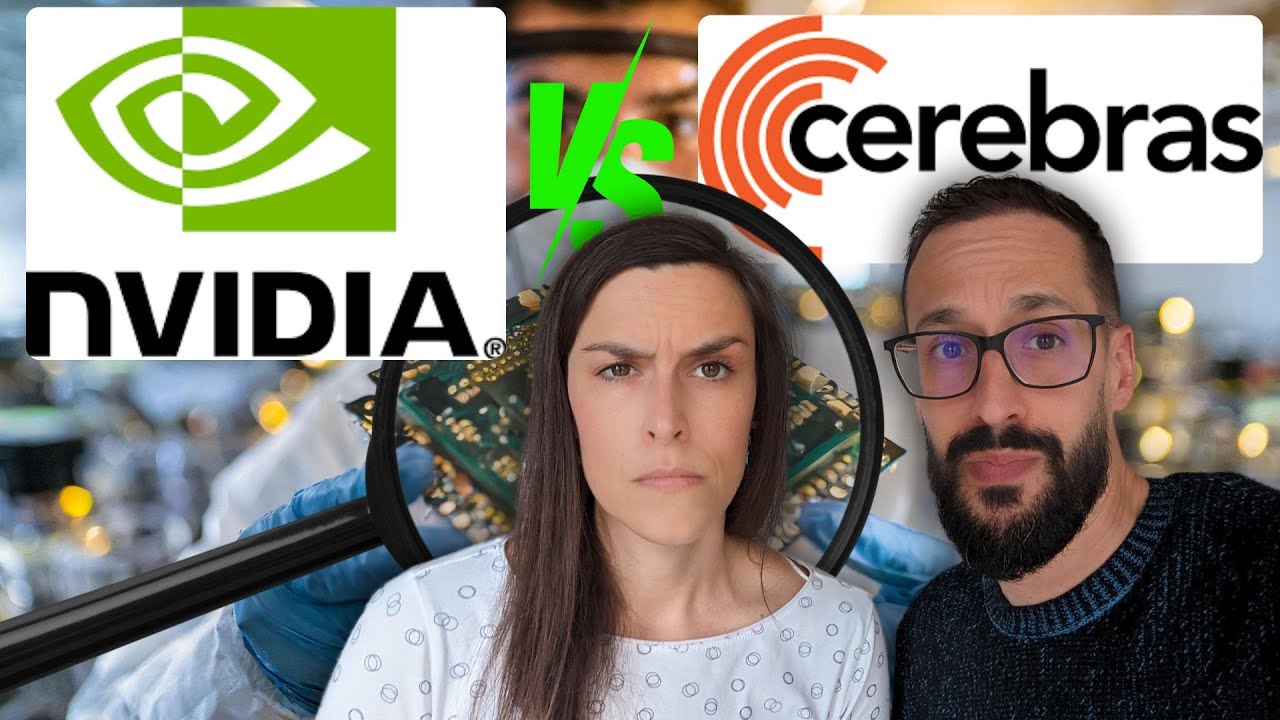
Показать описание
Nvidia's new Blackwell GPU is HUGE, literally! If you’re looking to be an Nvidia AI chip competitor, why not just make physically bigger chips? In this video, we explore the physics and economics behind AI chip design. We'll cover Nvidia's Blackwell packaging secrets, rival Cerebras Systems' wafer-scale chips, and the critical role of fab equipment makers in the race for AI system dominance.
Other vids to check out:
**************************************************************************************
Affiliate links that are sprinkled in throughout this video. If something catches your eye and you decide to buy it, we might earn a little coffee money. Thanks for helping us (Kasey) fuel our caffeine addiction!
Chapters:
00:00 Introduction to Megachips: Why It's Not Simple
00:29 Exploring NVIDIA's Blackwell GPU and Cerebras' Monster Chip
01:22 Diving Deep into Chip Manufacturing Challenges
03:53 Advanced Packaging Techniques: Chiplets and Heterogeneous Integration
10:25 Cerebras' Wafer Scale Engine: A Game Changer?
12:13 The Five Major Challenges of Megachip Manufacturing
16:41 Economic Constraints and the Future of Chip Manufacturing
18:44 Investment Opportunities in the Semiconductor Industry
Content in this video is for general information or entertainment only and is not specific or individual investment advice. Forecasts and information presented may not develop as predicted and there is no guarantee any strategies presented will be successful. All investing involves risk, and you could lose some or all of your principal.
#cerebras #nvidia #semiconductors #chips #investing #stocks #finance #financeeducation #silicon #artificialintelligence #ai #financeeducation #chipstocks #finance #stocks #investing #investor #financeeducation #stockmarket #chipstockinvestor #fablesschipdesign #chipmanufacturing #semiconductormanufacturing #semiconductorstocks
Nick and Kasey own shares of Nvidia, Cadence Design Systems, AMD, and Synopsys
Комментарии