filmov
tv
How Bioinformatics and AI Transforming Drug Discovery globally
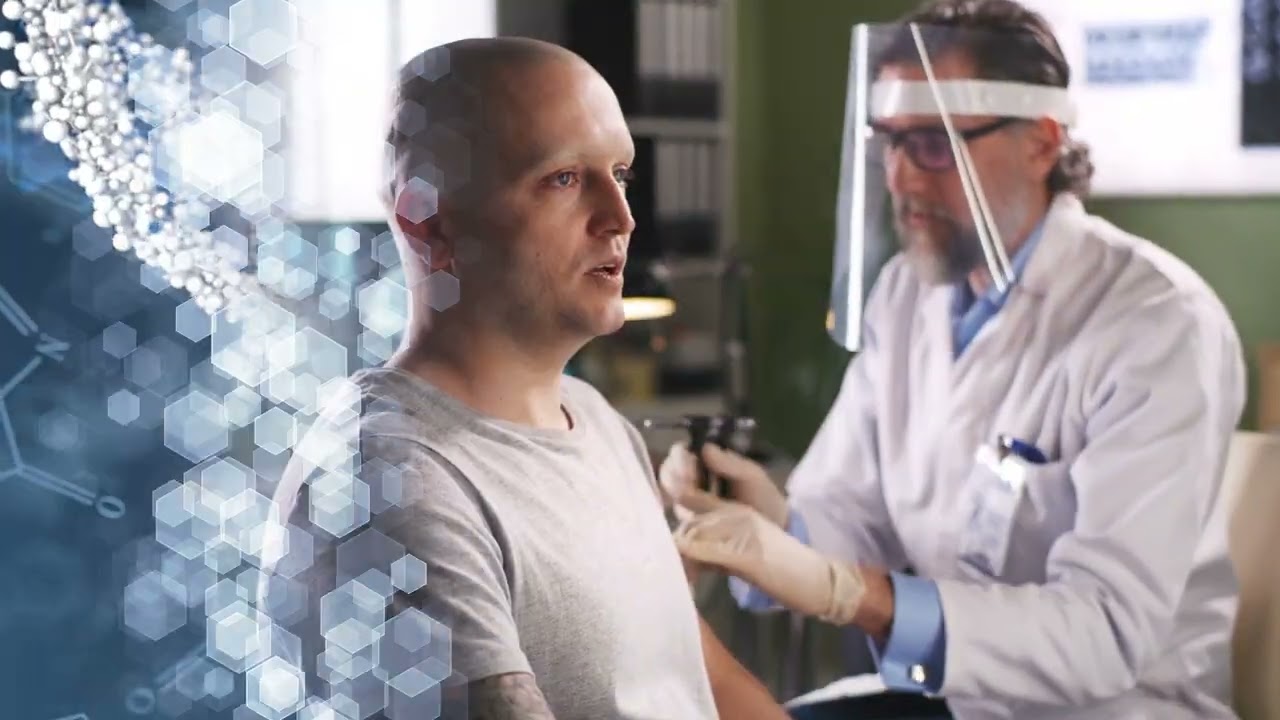
Показать описание
Role of Bioinformatics in Drug Discovery and Development
Bioinformatics plays a crucial role in drug discovery and development by leveraging computational tools and biological data to identify potential drug targets, understand cancer mechanisms, and improve the efficiency of clinical trials. Below are key areas where bioinformatics contributes significantly:
1. Identification of Cancer-Related Biomarkers
Bioinformatics aids in the identification of novel biomarkers for cancer by analyzing large datasets, such as gene expression profiles, genomic sequences, and proteomics data. These biomarkers are essential for the early detection, diagnosis, and monitoring of cancer progression, enabling the development of targeted therapies that are specific to cancer subtypes.
2. Genomic and Transcriptomic Data Analysis
Advanced bioinformatics tools are used to analyze genomic data (e.g., from DNA sequencing, next-generation sequencing, or genome-wide association studies) and transcriptomic data (e.g., RNA sequencing). By studying mutations, gene fusions, expression patterns, and alternative splicing events, bioinformaticians can identify key genes and pathways involved in cancer development and progression. These insights help researchers identify potential drug targets and optimize therapeutic strategies.
3. Target Discovery and Validation
Bioinformatics techniques such as pathway analysis, network modeling, and systems biology help researchers identify and validate new drug targets by analyzing molecular pathways involved in cancer. This includes the study of oncogenes, tumor suppressor genes, and signaling networks that are dysregulated in cancer cells. Bioinformatics tools facilitate the selection of high-confidence drug targets that are involved in key processes like cell cycle regulation, apoptosis, and metastasis.
4. Drug Repurposing and Virtual Screening
Bioinformatics tools can be used to accelerate drug discovery through virtual screening of large chemical libraries to identify compounds that can interact with cancer-related targets. This can lead to the identification of potential drug candidates for repurposing, where existing drugs may be found to be effective against certain cancer types. Virtual screening minimizes the need for labor-intensive wet-lab experiments, making the drug discovery process faster and more cost-effective.
6. Preclinical and Clinical Data Integration
Bioinformatics tools enable the integration and analysis of preclinical and clinical data, which can improve the understanding of how potential drugs behave in different biological environments. This includes modeling drug interactions, predicting side effects, and assessing the pharmacokinetics and pharmacodynamics of drug candidates. The analysis of clinical trial data allows for the optimization of dosing regimens and better prediction of patient responses.
7. Drug Resistance Mechanisms
Cancer drug resistance is a significant challenge in treatment. Bioinformatics helps identify molecular changes, such as mutations or epigenetic modifications, that may lead to resistance against specific drugs. By understanding the mechanisms of resistance, researchers can design combination therapies or develop second-line drugs to overcome resistance and improve patient outcomes.
8. Biological Data Sharing and Collaboration
Bioinformatics supports data sharing through public repositories (e.g., TCGA, GEO, or COSMIC), allowing researchers from around the world to access large datasets on cancer genomics, drug responses, and clinical outcomes. This fosters collaboration and accelerates the discovery of novel cancer therapies by enabling the comparison of results across multiple studies and facilitating meta-analysis.
9. Simulation and Modeling of Drug-Target Interactions
Computational methods, such as molecular docking, molecular dynamics simulations, and quantitative systems pharmacology, are essential in understanding how small molecules or biologics interact with cancer targets. These approaches predict how drugs will bind to their targets, providing insights into the drug’s efficacy, binding affinity, and potential side effects before clinical testing.
10. Precision in Clinical Trial Design
Bioinformatics can optimize the design of clinical trials by using predictive modeling and biomarkers to identify the most appropriate patient populations, reducing trial costs and improving success rates. By selecting patients who are more likely to benefit from a drug based on their genetic or molecular profiles, clinical trials can be more targeted and efficient.
Bioinformatics plays a crucial role in drug discovery and development by leveraging computational tools and biological data to identify potential drug targets, understand cancer mechanisms, and improve the efficiency of clinical trials. Below are key areas where bioinformatics contributes significantly:
1. Identification of Cancer-Related Biomarkers
Bioinformatics aids in the identification of novel biomarkers for cancer by analyzing large datasets, such as gene expression profiles, genomic sequences, and proteomics data. These biomarkers are essential for the early detection, diagnosis, and monitoring of cancer progression, enabling the development of targeted therapies that are specific to cancer subtypes.
2. Genomic and Transcriptomic Data Analysis
Advanced bioinformatics tools are used to analyze genomic data (e.g., from DNA sequencing, next-generation sequencing, or genome-wide association studies) and transcriptomic data (e.g., RNA sequencing). By studying mutations, gene fusions, expression patterns, and alternative splicing events, bioinformaticians can identify key genes and pathways involved in cancer development and progression. These insights help researchers identify potential drug targets and optimize therapeutic strategies.
3. Target Discovery and Validation
Bioinformatics techniques such as pathway analysis, network modeling, and systems biology help researchers identify and validate new drug targets by analyzing molecular pathways involved in cancer. This includes the study of oncogenes, tumor suppressor genes, and signaling networks that are dysregulated in cancer cells. Bioinformatics tools facilitate the selection of high-confidence drug targets that are involved in key processes like cell cycle regulation, apoptosis, and metastasis.
4. Drug Repurposing and Virtual Screening
Bioinformatics tools can be used to accelerate drug discovery through virtual screening of large chemical libraries to identify compounds that can interact with cancer-related targets. This can lead to the identification of potential drug candidates for repurposing, where existing drugs may be found to be effective against certain cancer types. Virtual screening minimizes the need for labor-intensive wet-lab experiments, making the drug discovery process faster and more cost-effective.
6. Preclinical and Clinical Data Integration
Bioinformatics tools enable the integration and analysis of preclinical and clinical data, which can improve the understanding of how potential drugs behave in different biological environments. This includes modeling drug interactions, predicting side effects, and assessing the pharmacokinetics and pharmacodynamics of drug candidates. The analysis of clinical trial data allows for the optimization of dosing regimens and better prediction of patient responses.
7. Drug Resistance Mechanisms
Cancer drug resistance is a significant challenge in treatment. Bioinformatics helps identify molecular changes, such as mutations or epigenetic modifications, that may lead to resistance against specific drugs. By understanding the mechanisms of resistance, researchers can design combination therapies or develop second-line drugs to overcome resistance and improve patient outcomes.
8. Biological Data Sharing and Collaboration
Bioinformatics supports data sharing through public repositories (e.g., TCGA, GEO, or COSMIC), allowing researchers from around the world to access large datasets on cancer genomics, drug responses, and clinical outcomes. This fosters collaboration and accelerates the discovery of novel cancer therapies by enabling the comparison of results across multiple studies and facilitating meta-analysis.
9. Simulation and Modeling of Drug-Target Interactions
Computational methods, such as molecular docking, molecular dynamics simulations, and quantitative systems pharmacology, are essential in understanding how small molecules or biologics interact with cancer targets. These approaches predict how drugs will bind to their targets, providing insights into the drug’s efficacy, binding affinity, and potential side effects before clinical testing.
10. Precision in Clinical Trial Design
Bioinformatics can optimize the design of clinical trials by using predictive modeling and biomarkers to identify the most appropriate patient populations, reducing trial costs and improving success rates. By selecting patients who are more likely to benefit from a drug based on their genetic or molecular profiles, clinical trials can be more targeted and efficient.