filmov
tv
Synthetic Data vs. Original Data [Comparison]
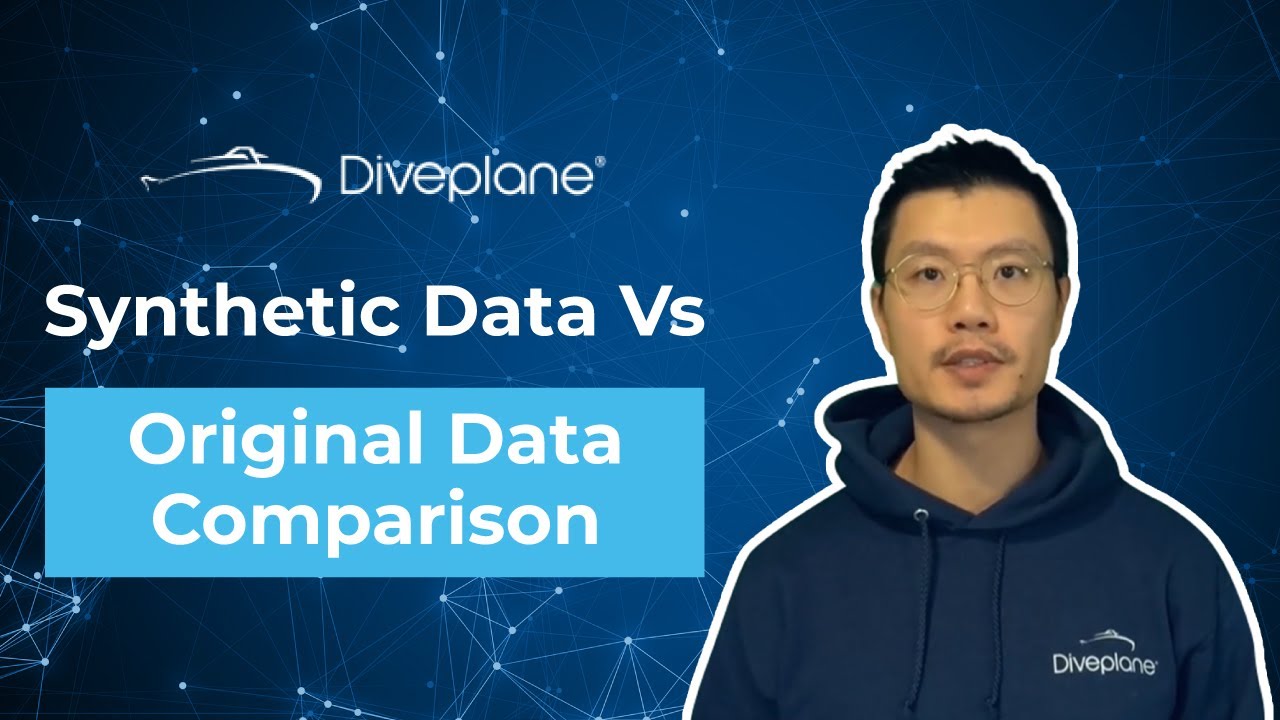
Показать описание
When using synthetic data, it is important to have as accurate data as possible. This is important to ensure that your output data is precise and true. Watch this video as Jack explains why the Data Quality Tool is your strongest ally.
Timestamps
0:00 Intro
0:17 Synthetic vs. Original Data
***************************************
At synthesis, GeminAI's Data Quality Tool reports on the synthetic data across a suite of accuracy, privacy, and machine learning viability measures. Having access to these measures grants your team confidence in the synthetic dataʼs resistance to attacks on privacy and the enduring statistical value of the data. Along with this detailed feedback, Data Quality Tool offers an overall desirability index to ensure the synthetic data is suitable for the use case.
Let Diveplane guide you on your path to success. When it comes to protecting your data and data research, it starts with us. We will find what works for your organization, and you can even try our products out. Contact us today and get started.
Video Transcript
Hi, my name is Jack Shu, Director of Sales Engineering here at Diveplane. In the last video, we talked about using the Data Quality Tool for privacy evaluation, and in this video, we're going to be talking about the Data Quality Tool for utility evaluation.
0:17 Synthetic vs. Original Data
At Diveplane, we take a very unique approach for utility evaluation. For example, if you consider just model comparison, what typically one would do is build a model using the synthetic data, build a model using the original data, and then compare the performance difference between the two.
At Diveplane, what we do is we actually open up the underlying understandable AI platform, and we build a model using the synthetic data, build a model using the original data set, and with our platform, instead of making a prediction of just one single target feature, you can actually make a prediction across every feature that exists in your data set. From there on, we can then compare the performance difference between the synthetic versus the original data across every feature.
This approach is actually closer to what Gemini does behind the scenes in that we preserve and maintain the accuracy across every feature that exists in the data set. This is important because it gives our users the assurance that they can use the synthetic data for whichever use case they may have in mind in the future.
Do you have any questions about synthetic data vs. original data? Let us know in the comments section below!
***************************************
Be sure to subscribe for more actionable entity resolution tips and tutorials.
FOLLOW US ON:
#diveplane #ai #syntheticdata #artificialintelligence #datamanagement
Timestamps
0:00 Intro
0:17 Synthetic vs. Original Data
***************************************
At synthesis, GeminAI's Data Quality Tool reports on the synthetic data across a suite of accuracy, privacy, and machine learning viability measures. Having access to these measures grants your team confidence in the synthetic dataʼs resistance to attacks on privacy and the enduring statistical value of the data. Along with this detailed feedback, Data Quality Tool offers an overall desirability index to ensure the synthetic data is suitable for the use case.
Let Diveplane guide you on your path to success. When it comes to protecting your data and data research, it starts with us. We will find what works for your organization, and you can even try our products out. Contact us today and get started.
Video Transcript
Hi, my name is Jack Shu, Director of Sales Engineering here at Diveplane. In the last video, we talked about using the Data Quality Tool for privacy evaluation, and in this video, we're going to be talking about the Data Quality Tool for utility evaluation.
0:17 Synthetic vs. Original Data
At Diveplane, we take a very unique approach for utility evaluation. For example, if you consider just model comparison, what typically one would do is build a model using the synthetic data, build a model using the original data, and then compare the performance difference between the two.
At Diveplane, what we do is we actually open up the underlying understandable AI platform, and we build a model using the synthetic data, build a model using the original data set, and with our platform, instead of making a prediction of just one single target feature, you can actually make a prediction across every feature that exists in your data set. From there on, we can then compare the performance difference between the synthetic versus the original data across every feature.
This approach is actually closer to what Gemini does behind the scenes in that we preserve and maintain the accuracy across every feature that exists in the data set. This is important because it gives our users the assurance that they can use the synthetic data for whichever use case they may have in mind in the future.
Do you have any questions about synthetic data vs. original data? Let us know in the comments section below!
***************************************
Be sure to subscribe for more actionable entity resolution tips and tutorials.
FOLLOW US ON:
#diveplane #ai #syntheticdata #artificialintelligence #datamanagement