filmov
tv
PSW 2445 The First Serious Problem Solved by AI | John Moult
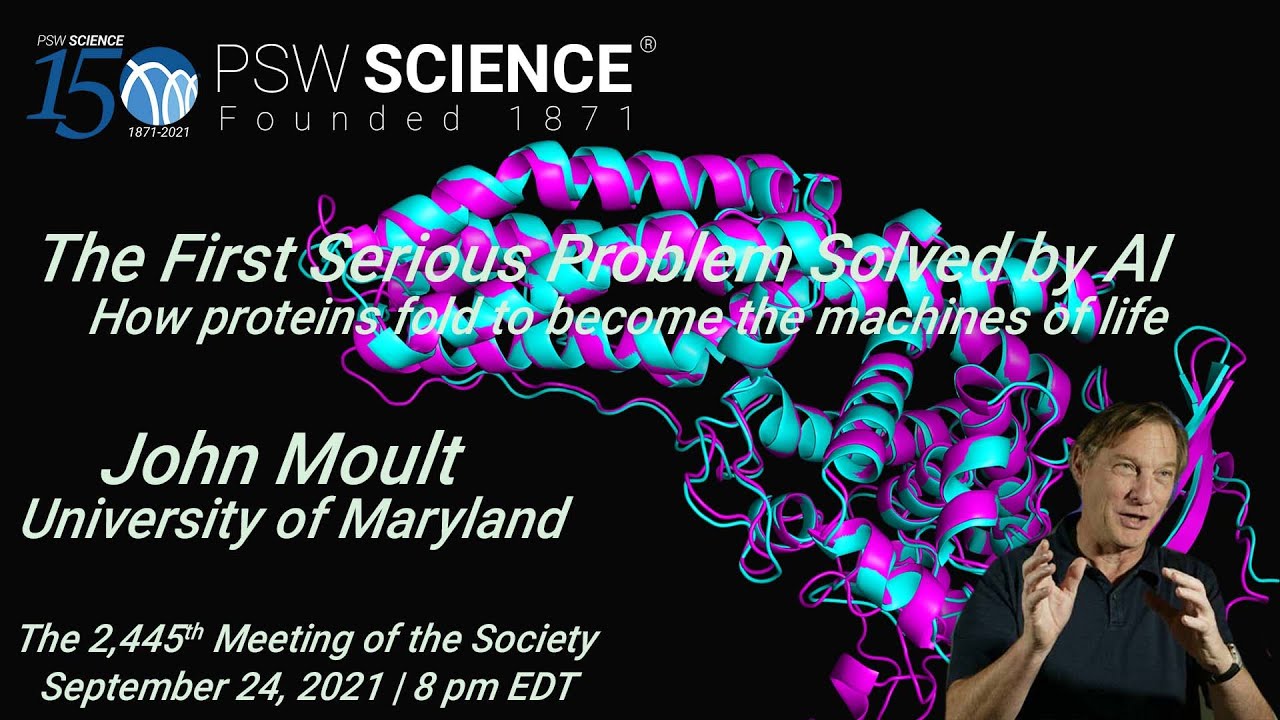
Показать описание
Lecture Starts at 10:59
PSW 2445
September 24, 2021
The First Serious Problem Solved by AI: How proteins fold to become the machines of life
John Moult, Institute for Bioscience and Biotechnology Research, University of Maryland
Artificial Intelligence is beginning to have an impact on our lives. So far, the impact is small. This talk will discuss the first serious problem to be solved with this technology – how protein molecules fold into their functional shapes. Genes code for proteins, and each gene’s sequence of DNA bases is translated into a polymer of 20 different sorts of amino acids. The sequence of amino acids is such that most proteins fold into a compact shape, in which each of its 1000s of atoms has an ordered position relative to all the other atoms. The number of possible structures (shapes) these extraordinary objects can adopt is super-astronomical, and the problem of computing structure from amino acid sequence has been one of the unsolved grand challenges of biochemistry for more than half a century.
Progress in the field has been advanced by a series of community experiments, aimed at maximizing rigor, transparency, and communication. Many methods have been developed, tested and discussed. However, while progress had been made and the field advanced, none of the approaches that have been developed has had more than partial success. At the most recent community experiment dramatic results were obtained showing that the program has largely been solved – by a branch of AI called Deep Learning. The calculated structures obtained by the Deep Learning methods are often comparable, and likely sometimes better, than representations to those obtained with state-of-the-art experimental techniques of crystallography and cryo-electron microscopy. The Deep Leaning protein models have already demonstrated an ability to solve previously intransigent experimental problems, and the results suggest the methods will be successfully applied to other areas of structural biology and more generally.
This lecture will describe the intellectual journey to this solution, the nature of the methods used, and some implications for the future of AI and its applications and effects on sciences and other areas of human endeavor.
John Moult is a Fellow at the Institute for Bioscience and Biotechnology Research and a Professor in the Department of Cell Biology and Molecular Genetics at Maryland. Previously he was a Founding Fellow of the Center for Advanced Research in Biotechnology at the University of Maryland. John did postdoctoral work at the Weizmann Institute of Science and at the University of Edinburgh, and he was then on the faculty at the University of Alberta, Canada before coming to the University of Maryland.
John is a leader in the development of new approaches to community science, aimed at maximizing rigor, transparency and communication, particularly in computational biology. He founded the Critical Assessment of Structure Prediction (CASP) to advance methods for computing protein structure, and he co-founded the Critical Assessment of Genome Interpretation Assessment (CAGI). He has also been instrumental in establishing several other community science programs, including DREAM (systems biology), CAPRI (protein-protein docking), SBV (quality assurance in industry research pipelines), and CACHE (drug design and discovery).
John’s early research was in the use of X-ray crystallography of proteins, notably he obtained the first structure of a beta-lactamase, an enzyme that breaks down penicillin class antibiotics and is a primary mechanism of resistance to those drugs. His work in computational studies of protein structure and function began in earnest after taking up his position in Maryland, and has included proofs that protein folding is formally chaotic and an NP hard problem, and the development of Monte Carlo molecular dynamics, genetic algorithms and other methods for studying protein structure and folding. More recently, he has developed machine learning and related methods for the analysis of the relationship between human genetic variation and disease, including deep learning and graph-based approaches for representing disease mechanisms.
He is an author on more than 150 scientific publications.
John earned his BS in Physics at the University of London and his PhD in Molecular Biophysics at the University of Oxford.
PSW 2445
September 24, 2021
The First Serious Problem Solved by AI: How proteins fold to become the machines of life
John Moult, Institute for Bioscience and Biotechnology Research, University of Maryland
Artificial Intelligence is beginning to have an impact on our lives. So far, the impact is small. This talk will discuss the first serious problem to be solved with this technology – how protein molecules fold into their functional shapes. Genes code for proteins, and each gene’s sequence of DNA bases is translated into a polymer of 20 different sorts of amino acids. The sequence of amino acids is such that most proteins fold into a compact shape, in which each of its 1000s of atoms has an ordered position relative to all the other atoms. The number of possible structures (shapes) these extraordinary objects can adopt is super-astronomical, and the problem of computing structure from amino acid sequence has been one of the unsolved grand challenges of biochemistry for more than half a century.
Progress in the field has been advanced by a series of community experiments, aimed at maximizing rigor, transparency, and communication. Many methods have been developed, tested and discussed. However, while progress had been made and the field advanced, none of the approaches that have been developed has had more than partial success. At the most recent community experiment dramatic results were obtained showing that the program has largely been solved – by a branch of AI called Deep Learning. The calculated structures obtained by the Deep Learning methods are often comparable, and likely sometimes better, than representations to those obtained with state-of-the-art experimental techniques of crystallography and cryo-electron microscopy. The Deep Leaning protein models have already demonstrated an ability to solve previously intransigent experimental problems, and the results suggest the methods will be successfully applied to other areas of structural biology and more generally.
This lecture will describe the intellectual journey to this solution, the nature of the methods used, and some implications for the future of AI and its applications and effects on sciences and other areas of human endeavor.
John Moult is a Fellow at the Institute for Bioscience and Biotechnology Research and a Professor in the Department of Cell Biology and Molecular Genetics at Maryland. Previously he was a Founding Fellow of the Center for Advanced Research in Biotechnology at the University of Maryland. John did postdoctoral work at the Weizmann Institute of Science and at the University of Edinburgh, and he was then on the faculty at the University of Alberta, Canada before coming to the University of Maryland.
John is a leader in the development of new approaches to community science, aimed at maximizing rigor, transparency and communication, particularly in computational biology. He founded the Critical Assessment of Structure Prediction (CASP) to advance methods for computing protein structure, and he co-founded the Critical Assessment of Genome Interpretation Assessment (CAGI). He has also been instrumental in establishing several other community science programs, including DREAM (systems biology), CAPRI (protein-protein docking), SBV (quality assurance in industry research pipelines), and CACHE (drug design and discovery).
John’s early research was in the use of X-ray crystallography of proteins, notably he obtained the first structure of a beta-lactamase, an enzyme that breaks down penicillin class antibiotics and is a primary mechanism of resistance to those drugs. His work in computational studies of protein structure and function began in earnest after taking up his position in Maryland, and has included proofs that protein folding is formally chaotic and an NP hard problem, and the development of Monte Carlo molecular dynamics, genetic algorithms and other methods for studying protein structure and folding. More recently, he has developed machine learning and related methods for the analysis of the relationship between human genetic variation and disease, including deep learning and graph-based approaches for representing disease mechanisms.
He is an author on more than 150 scientific publications.
John earned his BS in Physics at the University of London and his PhD in Molecular Biophysics at the University of Oxford.
Комментарии