filmov
tv
Weaving together machine learning, theoretical physics, and neuroscience
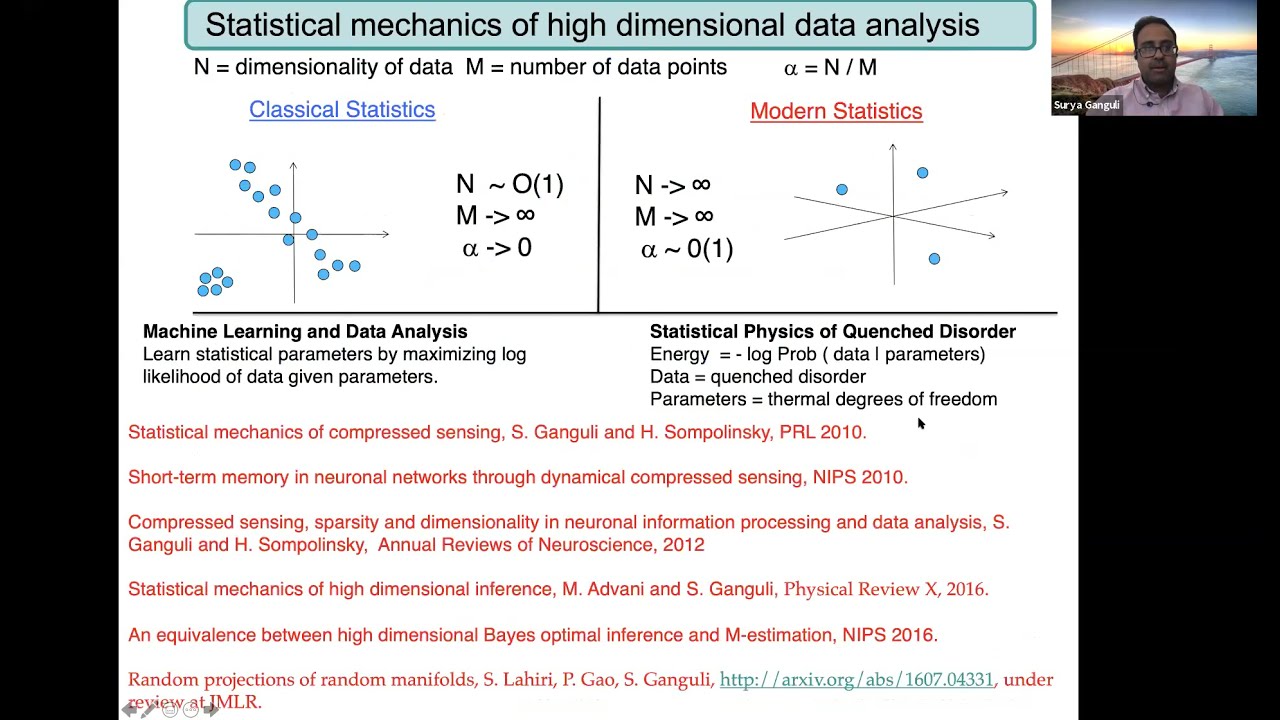
Показать описание
Surya Ganguli, Stanford University
Machine Learning Advances and Applications Seminar
Date and Time: Monday, April 5, 2021 - 3:00pm to 4:00pm
Abstract: An exciting area of intellectual activity in this century may well revolve around a synthesis of machine learning, theoretical physics, and neuroscience. The unification of these fields will likely enable us to exploit the power of complex systems analysis, developed in theoretical physics and applied mathematics, to elucidate the design principles governing neural systems, both biological and artificial, and deploy these principles to develop better algorithms in machine learning. We will give several vignettes in this direction, including: (1) determining the best optimization problem to solve in order to perform regression in high dimensions; (2) finding exact solutions to the dynamics of generalization error in deep linear networks; (3) derving the detailed structure of the primate retina by analyzing optimal convolutional auto-encoders of natural movies; (4) analyzing and explaining the origins of hexagonal firing patterns in recurrent neural networks trained to path-integrate; (5) understanding the geometry and dynamics of high dimensional optimization in the classical limit of dissipative many-body quantum optimizers.
Machine Learning Advances and Applications Seminar
Date and Time: Monday, April 5, 2021 - 3:00pm to 4:00pm
Abstract: An exciting area of intellectual activity in this century may well revolve around a synthesis of machine learning, theoretical physics, and neuroscience. The unification of these fields will likely enable us to exploit the power of complex systems analysis, developed in theoretical physics and applied mathematics, to elucidate the design principles governing neural systems, both biological and artificial, and deploy these principles to develop better algorithms in machine learning. We will give several vignettes in this direction, including: (1) determining the best optimization problem to solve in order to perform regression in high dimensions; (2) finding exact solutions to the dynamics of generalization error in deep linear networks; (3) derving the detailed structure of the primate retina by analyzing optimal convolutional auto-encoders of natural movies; (4) analyzing and explaining the origins of hexagonal firing patterns in recurrent neural networks trained to path-integrate; (5) understanding the geometry and dynamics of high dimensional optimization in the classical limit of dissipative many-body quantum optimizers.