filmov
tv
Lecture 13: Laws of large numbers.
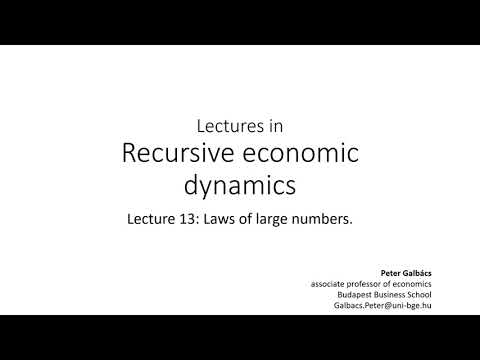
Показать описание
In this lecture we lay the foundations for comparisons between models and reality. In the last few lectures, we surveyed the convergence concepts of Markov processes, and paid special attention to the conditions under which we can expect a model describing a Markov process to converge to a probability measure. Speaking in broad terms, we set up a model to describe the optimal behavior of any economic agent, then we just let the agent operate by letting the model work. The result is a sort of dynamics: What path for capital is implied by the optimal consumption and investment decisions of agents in an economy, for instance. In a stochastic environment we work with probability distributions. The agents respond to changes in both endogenous and exogenous state variables, the latter including the stochastic and uncontrollable shocks, so the optimal path can best be described with a probability distribution. In any case, however, when we want to check if our model is true, then we need to turn to data. At this point our question for this lecture emerges: When and on what ground can we say that a model is consistent with the data? A systematic and generally applicable approach is needed. First and foremost, we distinguish two convergence concepts—convergence almost everywhere, and convergence in probability. The former is the stronger of the two, and hence establishes the strong law of large numbers.
Title page:(00:00)
Introduction:(00:10)
Random variables and expectations:(16:27)
The strong law of large numbers:(28:42)
Exercise 14.1:(35:00)
Exercise 14.2:(48:34)
Linear functionals:(63:44)
An example from p. 432:(69:12)
Linear operators:(88:46)
Exercise 14.4:(91:17)
Title page:(00:00)
Introduction:(00:10)
Random variables and expectations:(16:27)
The strong law of large numbers:(28:42)
Exercise 14.1:(35:00)
Exercise 14.2:(48:34)
Linear functionals:(63:44)
An example from p. 432:(69:12)
Linear operators:(88:46)
Exercise 14.4:(91:17)