filmov
tv
1-Bit LLM INSTALLATION| 7B LOCAL LLMs in 1-Bit + Test Demo #ai #llm
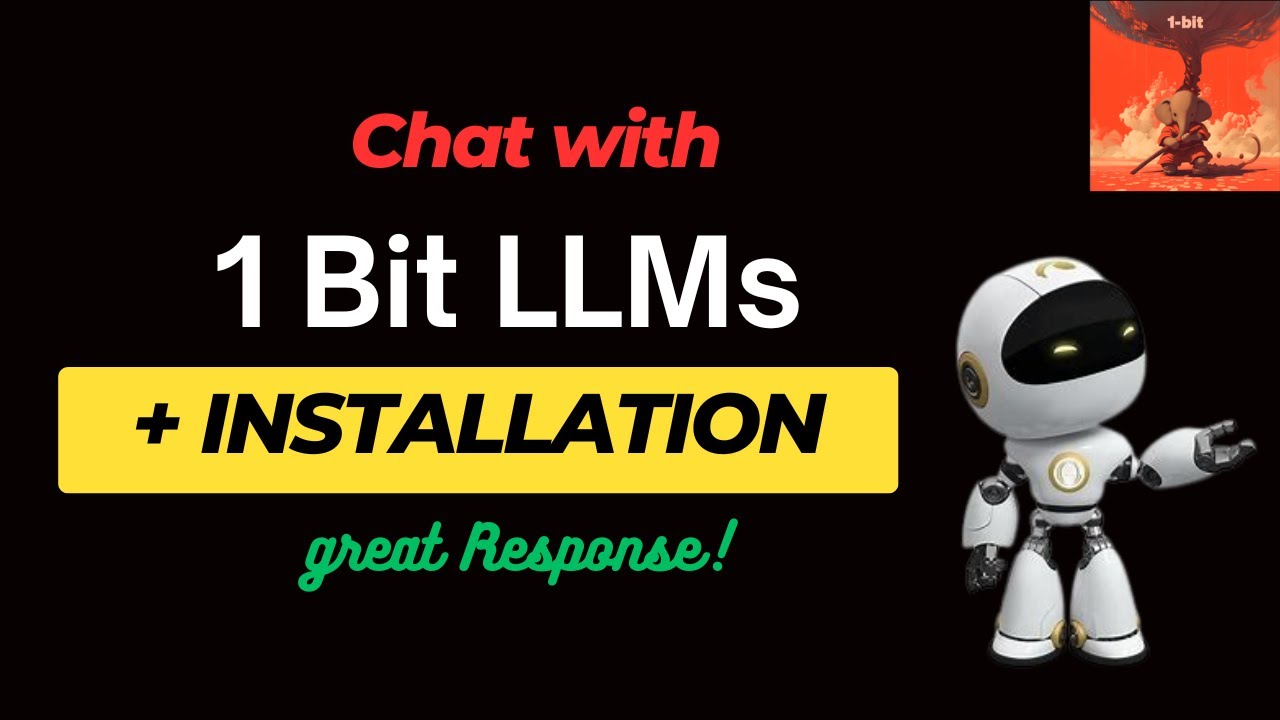
Показать описание
In recent developments, the machine learning community is diving deep into extreme low-bit quantization techniques such as BitNet and 1.58 bit, aiming to redefine compute efficiency by enabling matrix multiplication with quantized weights without actual multiplications. However, existing methods often entail training models from scratch, which can be both computationally expensive and less accessible.
To address this challenge, Mobius Labs GmbH presents a groundbreaking approach: direct quantization of pre-trained models with extreme settings, including binary weights (0s and 1s), through their adaptation called HQQ+. HQQ+ leverages a low-rank adapter to enhance its performance, allowing for fine-tuning of only a fraction of the weights on top of an HQQ-quantized model. This results in significant quality improvements even at 1-bit, surpassing smaller full-precision models in output quality.
HQQ (Half Quadratic Quantization) serves as a fast and accurate model quantizer that eliminates the need for calibration data. Implementation is straightforward, requiring just a few lines of code for the optimizer, and it can quantize models like Llama2-70B in a mere 4 minutes.
This method rethinks the dequantization step to directly exploit extreme low-bit matrix multiplication, leveraging efficient matrix operations and low-rank adapters to enhance quantization results. Benchmarking against full-precision and other quantization methods, experiments showcase remarkable improvements in output quality for both 1-bit and 2-bit models. Notably, the HQQ+ 1-bit model achieves comparable performance to the 2-bit Quip# model, highlighting the effectiveness of this approach.
These findings pave a promising path for making larger machine learning models more accessible by significantly reducing memory and compute requirements through extreme low-bit quantization.
Join us for a demo as we explore the implementation of a 1-bit model (Llama2) from Hugging Face, installed locally, to build a chatbot and test its capabilities. Dive into the future of machine learning with HQQ+!
#ai #llm #localllms #opensourcellm #opensourcecommunity #largelanguagemodels
LINKS:
To address this challenge, Mobius Labs GmbH presents a groundbreaking approach: direct quantization of pre-trained models with extreme settings, including binary weights (0s and 1s), through their adaptation called HQQ+. HQQ+ leverages a low-rank adapter to enhance its performance, allowing for fine-tuning of only a fraction of the weights on top of an HQQ-quantized model. This results in significant quality improvements even at 1-bit, surpassing smaller full-precision models in output quality.
HQQ (Half Quadratic Quantization) serves as a fast and accurate model quantizer that eliminates the need for calibration data. Implementation is straightforward, requiring just a few lines of code for the optimizer, and it can quantize models like Llama2-70B in a mere 4 minutes.
This method rethinks the dequantization step to directly exploit extreme low-bit matrix multiplication, leveraging efficient matrix operations and low-rank adapters to enhance quantization results. Benchmarking against full-precision and other quantization methods, experiments showcase remarkable improvements in output quality for both 1-bit and 2-bit models. Notably, the HQQ+ 1-bit model achieves comparable performance to the 2-bit Quip# model, highlighting the effectiveness of this approach.
These findings pave a promising path for making larger machine learning models more accessible by significantly reducing memory and compute requirements through extreme low-bit quantization.
Join us for a demo as we explore the implementation of a 1-bit model (Llama2) from Hugging Face, installed locally, to build a chatbot and test its capabilities. Dive into the future of machine learning with HQQ+!
#ai #llm #localllms #opensourcellm #opensourcecommunity #largelanguagemodels
LINKS:
Комментарии