filmov
tv
Liquid Neural Networks
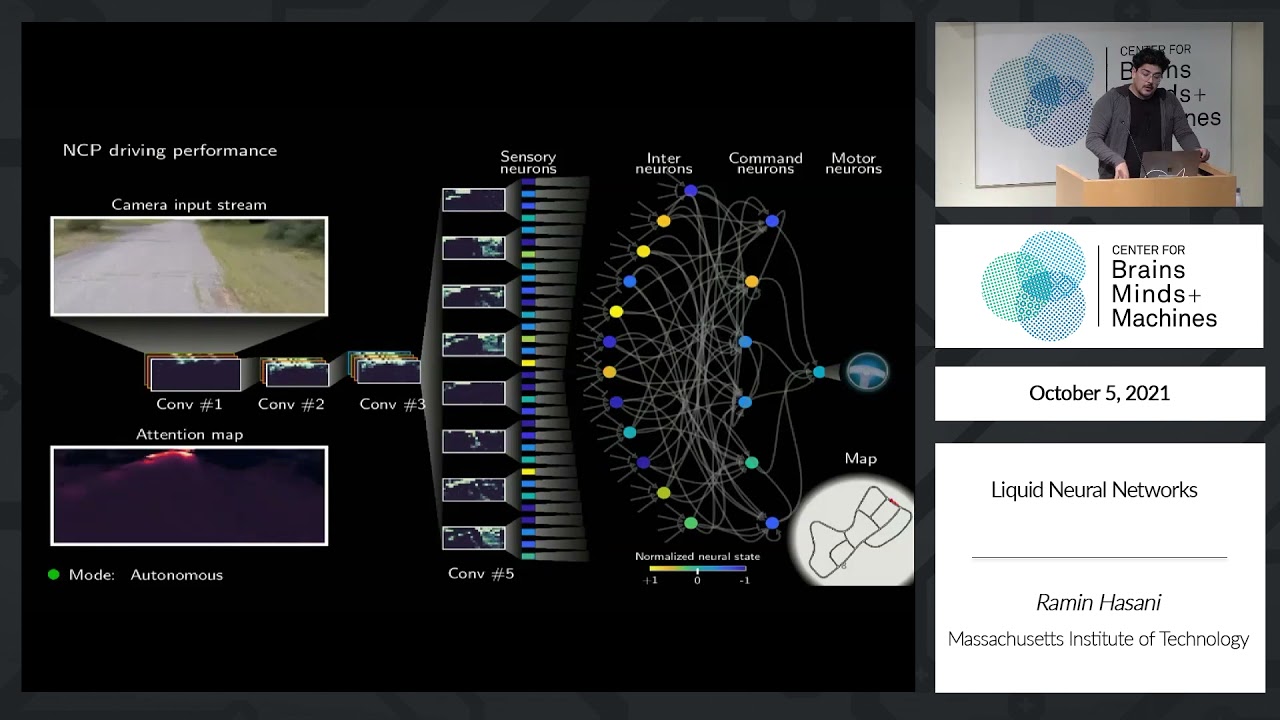
Показать описание
Ramin Hasani, MIT - intro by Daniela Rus, MIT
Abstract: In this talk, we will discuss the nuts and bolts of the novel continuous-time neural network models: Liquid Time-Constant (LTC) Networks. Instead of declaring a learning system's dynamics by implicit nonlinearities, LTCs construct networks of linear first-order dynamical systems modulated via nonlinear interlinked gates. LTCs represent dynamical systems with varying (i.e., liquid) time-constants, with outputs being computed by numerical differential equation solvers. These neural networks exhibit stable and bounded behavior, yield superior expressivity within the family of neural ordinary differential equations, and give rise to improved performance on time-series prediction tasks compared to advance recurrent network models.
Speaker Biographies:
Dr. Daniela Rus is the Andrew (1956) and Erna Viterbi Professor of Electrical Engineering and Computer Science and Director of the Computer Science and Artificial Intelligence Laboratory (CSAIL) at MIT. Rus’s research interests are in robotics, mobile computing, and data science. Rus is a Class of 2002 MacArthur Fellow, a fellow of ACM, AAAI and IEEE, and a member of the National Academy of Engineers, and the American Academy of Arts and Sciences. She earned her PhD in Computer Science from Cornell University. Prior to joining MIT, Rus was a professor in the Computer Science Department at Dartmouth College.
Dr. Ramin Hasani is a postdoctoral associate and a machine learning scientist at MIT CSAIL. His primary research focus is on the development of interpretable deep learning and decision-making algorithms for robots. Ramin received his Ph.D. with honors in Computer Science at TU Wien, Austria. His dissertation on liquid neural networks was co-advised by Prof. Radu Grosu (TU Wien) and Prof. Daniela Rus (MIT). Ramin is a frequent TEDx speaker. He has completed an M.Sc. in Electronic Engineering at Politecnico di Milano (2015), Italy, and has got his B.Sc. in Electrical Engineering – Electronics at Ferdowsi University of Mashhad, Iran (2012).
Abstract: In this talk, we will discuss the nuts and bolts of the novel continuous-time neural network models: Liquid Time-Constant (LTC) Networks. Instead of declaring a learning system's dynamics by implicit nonlinearities, LTCs construct networks of linear first-order dynamical systems modulated via nonlinear interlinked gates. LTCs represent dynamical systems with varying (i.e., liquid) time-constants, with outputs being computed by numerical differential equation solvers. These neural networks exhibit stable and bounded behavior, yield superior expressivity within the family of neural ordinary differential equations, and give rise to improved performance on time-series prediction tasks compared to advance recurrent network models.
Speaker Biographies:
Dr. Daniela Rus is the Andrew (1956) and Erna Viterbi Professor of Electrical Engineering and Computer Science and Director of the Computer Science and Artificial Intelligence Laboratory (CSAIL) at MIT. Rus’s research interests are in robotics, mobile computing, and data science. Rus is a Class of 2002 MacArthur Fellow, a fellow of ACM, AAAI and IEEE, and a member of the National Academy of Engineers, and the American Academy of Arts and Sciences. She earned her PhD in Computer Science from Cornell University. Prior to joining MIT, Rus was a professor in the Computer Science Department at Dartmouth College.
Dr. Ramin Hasani is a postdoctoral associate and a machine learning scientist at MIT CSAIL. His primary research focus is on the development of interpretable deep learning and decision-making algorithms for robots. Ramin received his Ph.D. with honors in Computer Science at TU Wien, Austria. His dissertation on liquid neural networks was co-advised by Prof. Radu Grosu (TU Wien) and Prof. Daniela Rus (MIT). Ramin is a frequent TEDx speaker. He has completed an M.Sc. in Electronic Engineering at Politecnico di Milano (2015), Italy, and has got his B.Sc. in Electrical Engineering – Electronics at Ferdowsi University of Mashhad, Iran (2012).
Комментарии