filmov
tv
GPT-4o Mini Arrives In Global IT Outage, But How ‘Mini’ Is Its Intelligence?
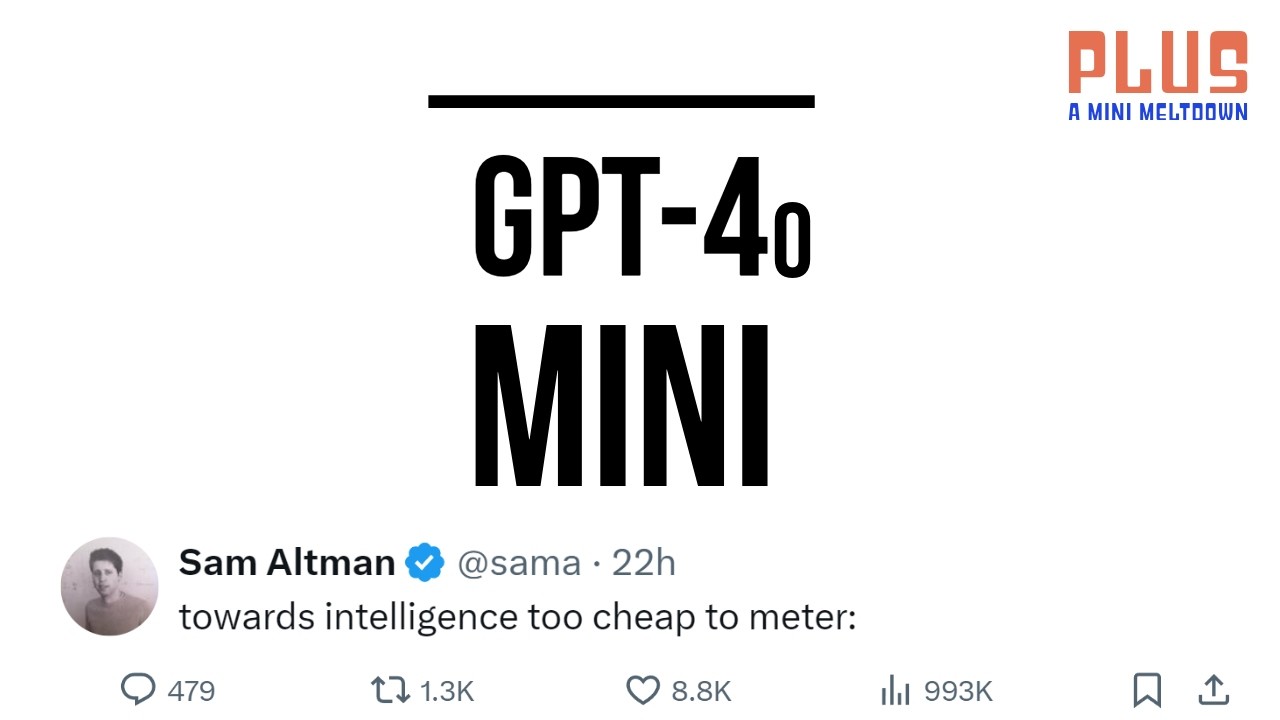
Показать описание
GPT 4o Mini from OpenAI is here, and Windows melts down. Coincidence? Definitely. But 100 million free users might soon be using it, so I’ve been scrutinising the model relentlessly since last night and will explain why OpenAI might need to be a bit more honest about the tradeoffs involved, and where they might head next, with reasoning, physical intelligence and more. Plus Fei-Fei Li, USMLE, and Roon.
GPT-4o Mini Arrives In Global IT Outage, But How ‘Mini’ Is Its Intelligence?
New GPT-4o Mini is Here & More AI Use Cases
OpenAI Unveils Game-Changing GPT-4o Mini
How to use ChatGPT 4o mini for free: New ChatGPT released by Open AI | Use ChatGPT 4o mini
GPT-4o - Full Breakdown + Bonus Details
26 Incredible Use Cases for the New GPT-4o
OpenAI GPT-4o MINI Jailbreak, Mauvaise Nouvelle pour l'UE, Plusieurs IA Mistral et Apple..- Act...
Last Week in AI #175 - GPT-4o Mini, OpenAI's Strawberry, of A Million Experts
Sparks of AGI: early experiments with GPT-4
Llama 3.1 is WAY better than we (or most people) expected
But what is a GPT? Visual intro to transformers | Chapter 5, Deep Learning
Llama 405b: Full 92 page Analysis, and Uncontaminated SIMPLE Benchmark Results
Open WebUI, Ollama, GPT-4o, RAG, Tool Use, Agent-Mastering Building Your Own AI
I Installed Chat GPT In Home Assistant And The Results Were Amazing!
Google CEO Sundar Pichai and the Future of AI | The Circuit
Microsoft's INSANE NEW Open Source AI Model 'Orca' RIVALS GPT-4!
Orca - ChatGPT performance in a much smaller model
This is the dangerous AI that got Sam Altman fired. Elon Musk, Ilya Sutskever.
old 1969 LIFE magazine: Apollo 11 LEM, retro ads, chatGPT (GPT-4) | ASMR ramble
Insane New AI Model - ORCA - That Finally Beats GPT-4
LLM Explained | What is LLM
BING IS POWERED BY GPT-4 (You Will NOT Want to Use Google After Watching)
M4 iPad Pro Hands-On, ChatGPT-4o vs. Google in AI Arms Race, “I Liked the Internet”
Le nouveau modèle de Chat GPT est perturbant
Комментарии