filmov
tv
Robust kernelized minimum enclosing balls in machine learning
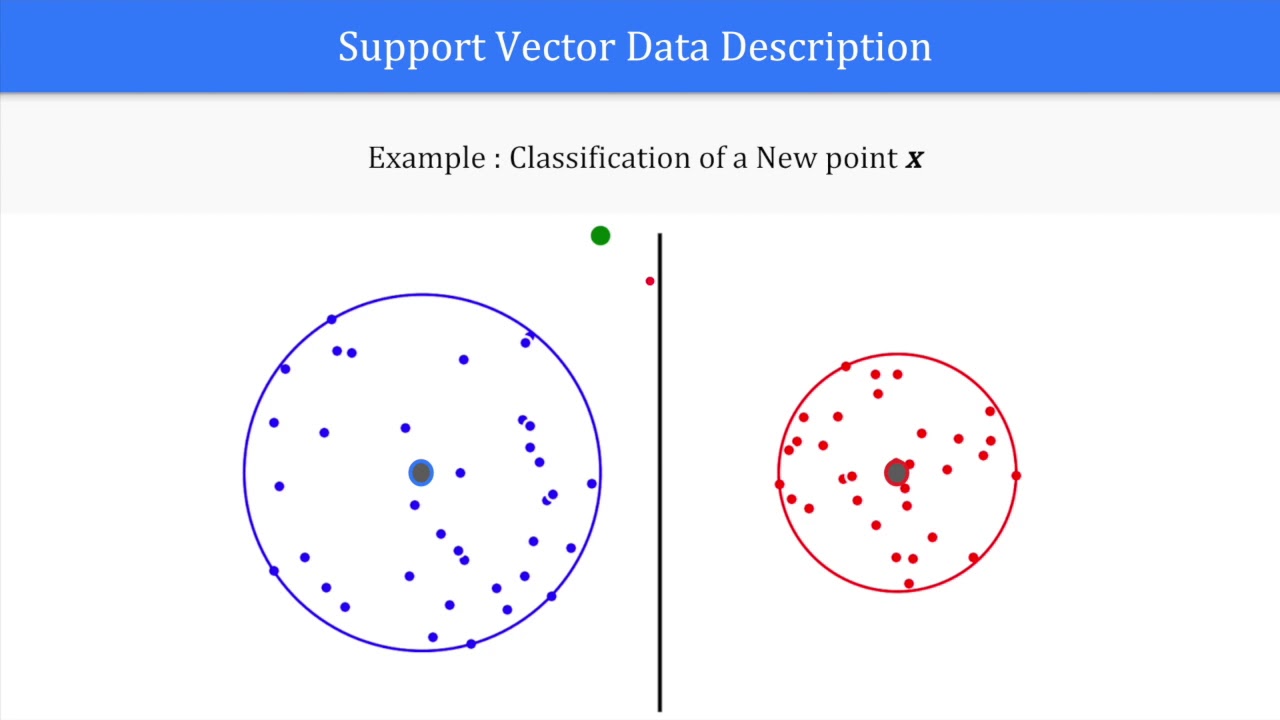
Показать описание
In this video, we first describe how to compute arbitrarily fine approximations of the Minimum
Enclosing Balls (MEBs) in a feature Hilbert space induced by a kernel using the Frank-Wolfe
optimization algorithm, and show how to make these balls robust by allowing outliers. We then
explain several applications of these robust kernelized MEBs in machine learning by presenting
applications to supervised multiclass classification, unsupervised clustering, and deep generative
models.
Enclosing Balls (MEBs) in a feature Hilbert space induced by a kernel using the Frank-Wolfe
optimization algorithm, and show how to make these balls robust by allowing outliers. We then
explain several applications of these robust kernelized MEBs in machine learning by presenting
applications to supervised multiclass classification, unsupervised clustering, and deep generative
models.