filmov
tv
Ben Glocker: 'Causality matters in medical imaging'
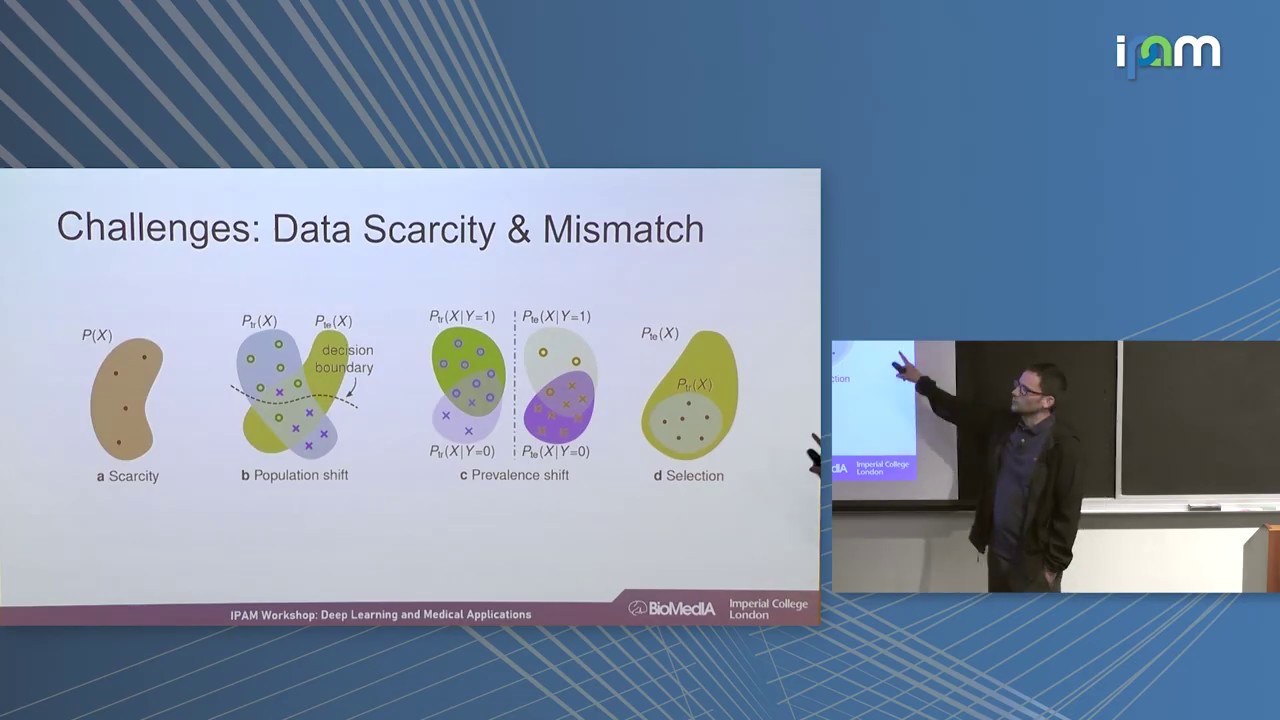
Показать описание
Deep Learning and Medical Applications 2020
"Causality matters in medical imaging"
Ben Glocker - Imperial College London, Department of Computing
Abstract: We use causal reasoning to shed new light on key challenges in medical imaging: 1) data scarcity, which is the limited availability of high-quality annotations, and 2) data mismatch, whereby a trained algorithm may fail to generalize in clinical practice. We argue that causal relationships between images, annotations, and data-collection processes can not only have profound effects on the performance of predictive models, but may even dictate which learning strategies should be considered in the first place. Semi-supervision, for example, may be unsuitable for image segmentation - one of the possibly surprising insights from our causal considerations in medical image analysis. We conclude that it is of utmost importance for the success of machine-learning-based image analysis that researchers are aware of and account for the causal relationships underlying their data.
Institute for Pure and Applied Mathematics, UCLA
January 30, 2020
"Causality matters in medical imaging"
Ben Glocker - Imperial College London, Department of Computing
Abstract: We use causal reasoning to shed new light on key challenges in medical imaging: 1) data scarcity, which is the limited availability of high-quality annotations, and 2) data mismatch, whereby a trained algorithm may fail to generalize in clinical practice. We argue that causal relationships between images, annotations, and data-collection processes can not only have profound effects on the performance of predictive models, but may even dictate which learning strategies should be considered in the first place. Semi-supervision, for example, may be unsuitable for image segmentation - one of the possibly surprising insights from our causal considerations in medical image analysis. We conclude that it is of utmost importance for the success of machine-learning-based image analysis that researchers are aware of and account for the causal relationships underlying their data.
Institute for Pure and Applied Mathematics, UCLA
January 30, 2020
Ben Glocker: 'Causality matters in medical imaging'
Round Table Discussion - Causality in Medical Image Computing
Training data for deep learning: what is needed? - Ben Glocker
Causality in Medical Imaging by Daniel C. Castro and Ben Glocker
Uncertainty, causality and generalization: Improve image-based predictive modelling | Ben Glocker
Daniel Coelho De Castro - Causality Matters in Medical Imaging
Uncertainty, Causality and Generalization | Ben Glocker
CauseMIC 2023 - Introduction
Session 2 Q&A - CauseMIC Tutorial
Discovering Patterns in Medical Images with Intelligent Algorithms | Ben Glocker
MIUA 2021 Keynote Lecture: Dr Ben Glocker - Towards Safer AI in Medical Imaging
CauseMIC 2023 - Fabio De Sousa Ribeiro
Deep Learning for image segmentation - Ben Glocker - ECR 2018
Research talk: Causality for medical image analysis
Counterfactuals in Medical Imaging by Nick Pawlowski and Kayhan Batmanghelich
CauseMIC 2023 - Jessica Schrouff
Test-time adaptable neural networks for robust medical image segmentation - Ender Konukoglu
[MISS 2016] Ben Glocker - Deep Learning for Brain Lesion Segmentation
Successes and pitfalls in Benchmarking Biomedical Image Analysis Algorithms - Lena Maier-Hein
Causal Inference in Deep Learning (Podcast Overview with Brady Neal)
Jonathan Richens - Improving the Accuracy of Medical Diagnosis with Causal Machine Learning
The Quest for Robust Machine Learning - Ben Glocker
Introduction to Potential Outcomes by Kristin Linn
Domain Aware Medical Image Classifier Interpretation by Counterfactual Impact Analysis
Комментарии