filmov
tv
Exploratory Data Analysis (EDA) with Python
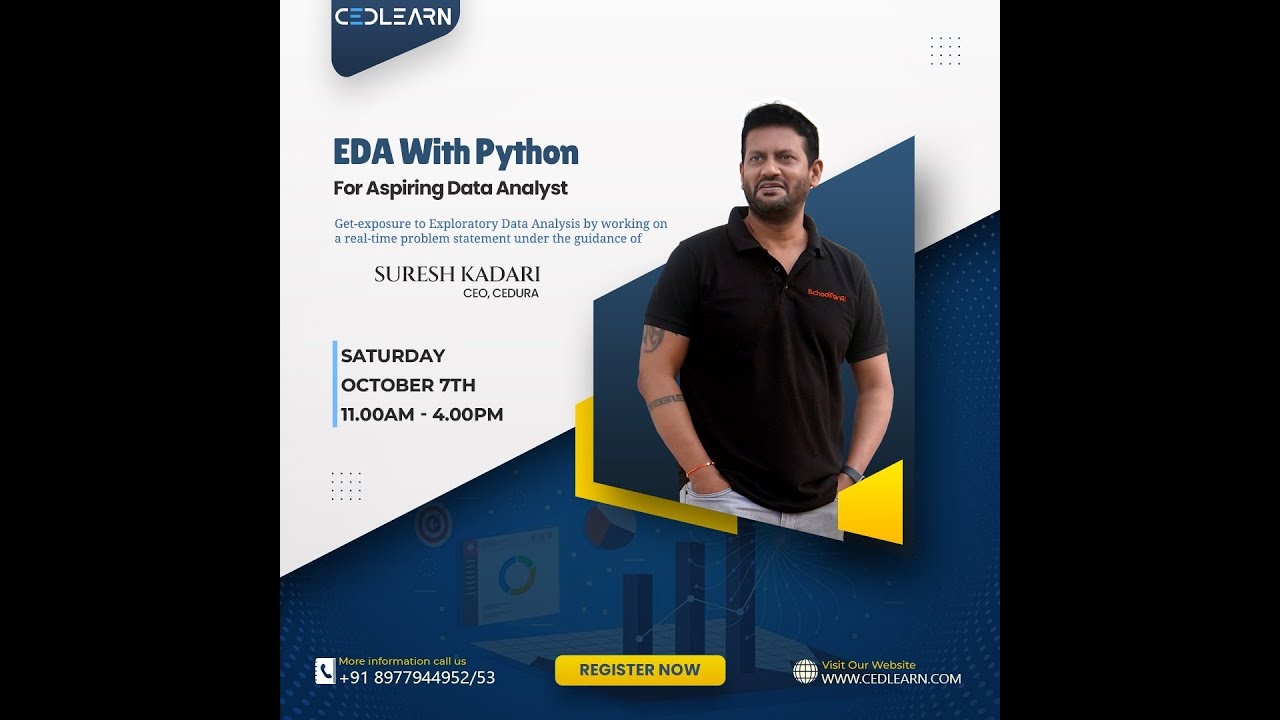
Показать описание
Exploratory Data Analysis (EDA) is a crucial step in data analysis where the primary focus is to understand the characteristics of the dataset. By employing statistical and graphical techniques, EDA helps analysts and data scientists to uncover patterns, relationships, anomalies, and insights within the data. EDA is typically the first step after data collection and cleaning and is essential for making informed decisions, building accurate models, and deriving meaningful conclusions.
In Python, EDA is commonly performed using libraries such as Pandas, Matplotlib, Seaborn, and Plotly. Here is a breakdown of the steps involved in EDA with Python:
1. Loading Data:
Load the dataset into a Pandas DataFrame, enabling easy manipulation and analysis of the data.
2. Understanding the Data:
Summary Statistics: Compute basic statistics like mean, median, and standard deviation.
Data Types and Missing Values: Check data types of columns and identify any missing or null values.
3. Cleaning Data:
Handling Missing Values: Impute or remove missing values to ensure data integrity.
Outlier Detection: Identify and deal with outliers that can skew analysis.
4. Analyzing Data:
Univariate Analysis: Explore individual variables using histograms, box plots, and frequency distributions.
Bivariate Analysis: Investigate relationships between two variables using scatter plots, heatmaps, and correlation matrices.
Multivariate Analysis: Examine relationships involving multiple variables simultaneously.
5. Visualizing Data:
Utilize various types of plots (bar plots, line charts, violin plots, etc.) to visualize data patterns and trends.
Leverage interactive visualization libraries like Plotly for in-depth exploration.
6. Drawing Insights:
Derive meaningful insights based on the analysis, aiding in decision-making processes.
Formulate hypotheses for further analysis or modeling.
7. Iterative Process:
EDA is often iterative. Initial findings might lead to more specific questions, prompting further analysis.
Python's rich ecosystem of libraries makes it a powerful tool for EDA. The choice of visualizations and analysis techniques depends on the nature of the dataset and the objectives of the analysis. EDA is not just a one-time task; it’s an ongoing process that evolves as the data analysis progresses, ensuring a comprehensive understanding of the data at hand.
In Python, EDA is commonly performed using libraries such as Pandas, Matplotlib, Seaborn, and Plotly. Here is a breakdown of the steps involved in EDA with Python:
1. Loading Data:
Load the dataset into a Pandas DataFrame, enabling easy manipulation and analysis of the data.
2. Understanding the Data:
Summary Statistics: Compute basic statistics like mean, median, and standard deviation.
Data Types and Missing Values: Check data types of columns and identify any missing or null values.
3. Cleaning Data:
Handling Missing Values: Impute or remove missing values to ensure data integrity.
Outlier Detection: Identify and deal with outliers that can skew analysis.
4. Analyzing Data:
Univariate Analysis: Explore individual variables using histograms, box plots, and frequency distributions.
Bivariate Analysis: Investigate relationships between two variables using scatter plots, heatmaps, and correlation matrices.
Multivariate Analysis: Examine relationships involving multiple variables simultaneously.
5. Visualizing Data:
Utilize various types of plots (bar plots, line charts, violin plots, etc.) to visualize data patterns and trends.
Leverage interactive visualization libraries like Plotly for in-depth exploration.
6. Drawing Insights:
Derive meaningful insights based on the analysis, aiding in decision-making processes.
Formulate hypotheses for further analysis or modeling.
7. Iterative Process:
EDA is often iterative. Initial findings might lead to more specific questions, prompting further analysis.
Python's rich ecosystem of libraries makes it a powerful tool for EDA. The choice of visualizations and analysis techniques depends on the nature of the dataset and the objectives of the analysis. EDA is not just a one-time task; it’s an ongoing process that evolves as the data analysis progresses, ensuring a comprehensive understanding of the data at hand.